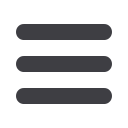

S249
ESTRO 36 2017
_______________________________________________________________________________________________
(TCGA) that are providing unprecedented information
about the human genome. NGS has the potential to
identify all kinds of genetic variation (not only SNPs) at
base-pair resolution throughout the human genome in a
single experiment. The identification of rare genomic
variants through these new sequencing technologies,
should allow us to unravel the heritability of complex
traits, such as radiation sensitivity. The genetic variants
identified through Radiogenomic studies could lead to the
development of an assay to predict a patient's risk of
toxicity. Such an assay could help to individualize
radiotherapy protocols leading to safer and more effective
outcomes.
SP-0474 From radiotherapy & dosimetry data to better
plans
M. Hoogeman
1
, S. Breedveld
1
, R. Bijman
1
, B. Heijmen
1
1
Erasmus MC Cancer Institute, Department of Radiation
Oncology, Rotterdam, The Netherlands
In radiotherapy patients are treated with a personalized
treatment plan, which optimizes the linear accelerator
settings for the delivery of a curative dose to the target
while sparing surrounding healthy tissues. Those settings
are calculated following a mathematical optimization,
balancing the treatment goals as specified by the
physician regarding target prescription dose and tolerable
doses to healthy tissues.
The generation of a radiotherapy treatment plan is a
complex procedure. The quality is not only highly
dependent on the planner skills, but also on the physician
or institutional preferences regarding the prioritization of
the sparing of organs-at-risk in relation to the coverage of
the planning target volume. A complicating factor is that
the semi-automated optimization can take up to hours or
even a day to complete and that it is often not know a-
priori what can be ultimately achieved in the trade-off
between organs-at-risk sparing and target coverage.
Another challenge in treatment planning is that the
quality of a treatment plan is only partly captured by
quantitative metrics such as DVH parameters and that
prediction models of toxicity are not yet integrated in the
process of treatment planning.
In this presentation the above challenges will be
addressed. It will be discussed if (big) radiotherapy data
of plans and dosimetry can make treatment plans better.
Prediction models combined with comparative treatment
planning can be used for decision making and further
personalizing the treatment. Some practical examples will
be presented regarding the selection of patients for
proton therapy. In addition, we will address to what
extent the accuracy of the prediction models impact such
clinical decisions. Finally, the role of treatment-plan
automation will be discussed in improving the quality of
treatment planning and different approaches of
automation will be compared.
SP-0475 Moving Big Data into Clinical Practice – A
positive outlook
S. Vinod
1
1
Liverpool Hospital, Cancer Therapy Centre, Liverpool
BC, Australia
The evidence-base underlying treatment of oncology
patients is derived from the 2-3% of patients enrolled in
prospective clinical trials. Outcomes in these highly
selected patients are then applied to the general patient
population. However, adherence to guideline treatment
varies from 44% in lung cancer to 91% in breast cancer due
to clinician uncertainty about the efficacy and toxicity of
evidence-based treatments in individual patients. An
alternative source of evidence is Big Data. This is what we
already collect in routine clinical practice including
clinical data, imaging data and genomic data. The type
and nature of data collected and the platform of
collection varies, however current systems can overcome
this to successfully enable distributed learning. Multi-
institutional data can be used to develop predictive
models relating outcomes to specific patient, tumour and
treatment characteristics. The strength of Big Data is in
the sheer number of patients and hence applicability of
findings to the general clinic population. Moving Big Data
into clinical practice requires translation of model outputs
to decision support systems to enable shared decision
making between clinicians and patients. It also requires
trust of the model by patients and clinicians. There is a
need to demonstrate that model predictions based on
objective parameters are superior to clinician’s subjective
judgement alone. Clinical trials of decision support
systems are necessary to evaluate whether Big Data can
change clinical practice. Only then can we truly deliver
personalised medicine tailored to an individual patient’s
specific parameters.
Symposium: Locally advanced breast cancer
SP-0476 Personalised local and locoregional
radiotherapy in breast cancer
T. Tramm
1
1
Aarhus University, Department of Clinical Medicine - The
Department of Pathology, Aarhus, Denmark
Genomic profiling has unveiled the heterogeneity of
breast cancer, and revealed prognostic differences and
prediction on benefit from systemic therapy. Although
the literature on gene expression profiles related to
prediction for response to different systemic treatment
strategies has been substantial, only a limited number of
studies have described molecular signatures associated
with local control and benefit from radiotherapy (RT).
The use of single markers or combinations of
immunohistochemical markers to divide patients
according to risk of LRR is potentially easily applicable in
a daily clinical setting.
Especially, the immunohistochemical approximations of
the intrinsic subtypes (based on
e.g.
ER, PR, HER2 and
Ki67) have attracted attention. Most consistently, the
Luminal A subtype has been associated with a low risk of
loco-regional recurrence (LRR). In a subgroup analysis of
the Canadian hypofractionation trial, it has also been
examined, if different treatment schemes may be more or
less suitable for the various subtypes, but no interaction
between hypofractionation and intrinsic subtypes was
found.
A number of molecular signatures prognostic of LRR have
also been identified, but until recently, the majority of
these signatures have failed to validate in independent
cohorts. Two studies by a Dutch group did not succeed in
identifying a specific gene-set predicting risk of
recurrence after breast conserving therapy (BCT), though
a gene-profile based on the wound response signature was
described as being of independent prognostic value. Later,
the same group developed a 111-gene signature, but it did
not show independent prognostic value in multivariate
analysis, and lost prognostic impact when tested in other
cohorts. A Swedish gene-profile aiming to identify patients
developing LRR despite of RT after BCT has also not been
independently validated.
The ideal setting for identification of a prognostic factor
is in a non-treated study population. Gene profiles
predicting LRR after BCT are, however, not strictly
prognostic, but include an element of prediction of
benefit from RT, since the vast majority of patients
treated with BCT have been treated with RT.
A few prognostic gene-expression profiles predicting risk
of LRR after mastectomy have, however, also been
published. One of these, the 18-gene classifier, was
developed from 135 non-irradiated patients treated with