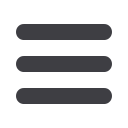

S248
ESTRO 36 2017
_______________________________________________________________________________________________
randomised trial design moving from pilot to phase II to
phase III, with the aim of reducing locoregional failure.
The primary endpoint for all 3 trials is 3 year local regional
failure, key secondary outcomes will focus on patient
reported outcome measures.
Joint Symposium: ESTRO-RANZCR: Big data to better
radiotherapy
SP-0472 The pros, cons, process and challenges for
achieving better radiotherapy through data -an
introduction
L.C. Holloway
1,2,3,4
1
Ingham Institute and Liverpool and Macarthur Cancer
Therapy Centres, Medical Physics, Sydney, Australia
2
University of Wollongong, Centre for Medical Radiation
Physics, Wollongong, Australia
3
University of Sydney, Institute of Medical Physics,
Sydney, Australia
4
University of New South Wales, South West Sydney
Clinical School, Sydney, Australia
The magnitude and use of data is expanding in many areas
including medicine. The opportunities for using data to
improve our knowledge and understanding are many and
varied including demographic, disease and outcome
investigations. Within current radiotherapy practice data
may be collected in a very rigorous approach within for
instance a clinical trial framework and data is also
collected in an ongoing fashion during standard clinical
practice. It is possible for us to gain knowledge from both
rigorously collected data and clinical practice data.
The gold standard of randomised clinical trial (RCT)
evidence is only provided from 2-3% of retrospective
patients who have been enrolled in RCTs and is only
directly applicable to a limited number of patients due to
strict trial eligibility criteria, necessary to ensure trial
rigour. Clinical practice data may provide us with the
opportunity to develop additional evidence to support
evidence from RCTs, utilising data from potentially all
previous patients and including patients who do not fit
RCT eligibility criteria. Considering data from both RCTs
and clinical practice may also enable us to learn from the
differences between the two. Different approaches to
learning from data have been undertaken. These range
from include common statistical approaches to machine
learning approaches. All data learning approaches require
the development and then validation of models.
Validation must be undertaken carefully to ensure that the
developed model is validated on independent datasets.
To utilise data we need data; ideally large datasets from
multiple treatment centres with varied clinical practice
and of high quality. Achieving this requires a number of
challenges to be addressed. Collecting large datasets can
be very challenging in the medical field due to ethics,
privacy and national and international regulations as well
as the practical and technical challenges of collecting
large datasets ( e.g. when using multiple medical images).
One approach to addressing this is termed ‘distributed
learning’ where datasets remain within the local
treatment centres. Computer algorithms can then be used
to both assess (as in practice comparison and demographic
studies) and learn from (as in model development
providing evidence for future treatment decisions) these
datasets. The requirement for varied clinical practice
generally requires international datasets where local
treatment guidelines may vary between countries. This
requires collaboration between centres and an active
effort to ‘translate’ between practices to ensure that data
items considered are consistent between the datasets.
Translation is necessary for simple items such as language
differences but also more challenging differences such as
different scoring scales or different approaches to
normalisation in quantitative data. The use of a standard
ontology which may need to be actively developed can
help streamline this.
Data quality will be an ongoing challenge. Ideally every
parameter within a dataset will be correctly recorded,
curated and with minimal variation in any scoring scales
or assessment criteria. Particularly within clinical practice
datasets it is highly unlikely that every parameter within
a dataset is correct although this varies both between and
within centres. There are two practical issues to be
considered, the first that of missing data where particular
parameters are not recorded for all patients or not
recorded for some patients and the second that of
incorrect data entries. Although a complete full quality
dataset is always preferred imputation approaches can be
used successfully to address missing data, increase dataset
size and thus increase model confidence. Incorrect data
entries are more challenging, however if incorrect data
entries are random and datasets are large then the impact
of this will be minimised and seen primarily in model
confidence parameters.
Although it is important to be aware of the limitations and
challenges of use of data, there is growing evidence that
use of data can improve our knowledge and understanding
of radiotherapy.
SP-0473 From Genomics and Radiogenomics data to a
better RT
A. Vega
1
1
Fundación Pública Galega Medicina Xenómica, Hospital
Clinico Santiago de Compostela, Santiago de
Compostela, Spain
The completion of The Human Genome Project in 2001,
after more than 10 years of international collaborative
efforts along with progress in technology, heralded an era
of enormous advances in the field of genetics. The study
of the genetic susceptibility behind the different response
of the irradiated tissue of patients treated with
radiotherapy, known today as Radiogenomics, is an
example of this. One of the major aims of Radiogenomics
is to identify genetic variants, primarily common variation
(SNPs), associated with normal tissue toxicity following
Radiotherapy. A large number of candidate-gene
association studies in patients with or without
Radiotherapy side-effects have been published in recent
years. These studies investigated SNPs in genes related to
radiation pathogenesis, such as DNA damage, DNA repair,
tissue remodeling and oxidative stress. Most of the studies
suffered from methodological shortcomings (small sample
sizes, lack of adjustment for other risk factors/covariates
or multiple testing). The Human Genome Project and the
subsequent International HapMap Project, provided
extremely valuable information on the common variation
of human DNA in different populations. This information
and the development of high-density SNP arrays, in which
all genome variability is considered (from 500000 to a few
million SNPs), enabled Genome Wide Association Studies
(GWAS) and an hypothesis-free case-control approach.
GWASs are a major breakthrough in the attempts to
unravel the genetics of common traits and diseases.
Simultaneous analysis of thousands of variants requires a
large sample size to achieve adequate statistical power.
The need for a large number of samples makes it essential
to collaborate and share data. A Radiogenomics
Consortium (RGC) was established in 2009 with
investigators from throughout the world who shared an
interest in identifying the genetic variants associated with
patient differences in response to radiation therapy. To
date, RGC collaborative large-scale projects have led to
statistically-powered gene-association studies, as well as
Radiogenomic GWASs. The recent availability of Next
Generation Sequencing (NGS) and advances in
Bioinformatics, have promoted major initiatives such as
The 1000 Genomes Project and The Cancer Genome Atlas