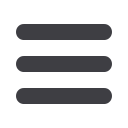

S244
ESTRO 36 2017
_______________________________________________________________________________________________
Recent studies highlight the relevance of DNA repair
defects in genome instability and tumour development.
Little is known about the impact of DNA repair aberrations
on patient prognosis or treatment outcome. However, new
targeted treatment options, such as PARP inhibitors, can
exploit these repair defects if present. Here we tested
whether gene expression analysis could identify DNA
repair defects, with the ultimate aim to determine an
association with clinical outcome and identify patients for
targeted treatments.
Material and Methods
Mitomycin C (MMC) or PARP inhibitor olaparib
hypersensitivity is a hallmark of functional homologous
recombination (HR) or Fanconi anaemia (FA) pathway DNA
repair defects. We determined whole transcriptome
expression and sensitivity to MMC and olaparib in a panel
of 28 patient derived head and neck squamous cell
carcinoma (HNSCC) cell lines. Based on their sensitivity
(IC50 values), cell lines were classified as
Normal (N)
,
hypersensitive to both drugs (
MOS
) or hypersensitive to
mitomycin C but not olaparib (
MS
). To esta blish a “DNA
repair defect” signature, relevant genes were extracted
by differential expression analysis and used as input to
various machine learning algorithms. Performance was
evaluated using 20 repetitions of 5-fold int ernal cross
validation.
Probabilities of defects calculated by these m odels were
used in a multivariate cox proportional hazard model to
determine their prognostic capacity in a cohort of 84
HNSCC tumours, treated with chemo-radiation, and the
TCGA HNSCC cohort.
Results
Expression analysis of the three groups yielded genes
enriched for targets of transcription factors involved in
DNA damage response, including p53, demonstrating its
relevance to the system under study. The random forest
model performed best, achieving a high sensitivity of 0.91
and specificity of 0.86.
We validated our model in the Cancer Genome Project
dataset of drug sensitivities in cell lines. The predicted
repair defected groups had significantly lower IC50 values
for DNA damage inducing agents, including cisplatin (MS:
p=5.9e-05; MOS: p=0.042).
Encouraged by this data, we used our model in the patient
data sets. Increased probabilities of DNA repair defects
were associated with increased mortality, recurrence and
disease progression in chemo-radiated patients with
advanced tumours in our cohort (shown in figure) and with
survival in the TCGA HNSCC cohort.
Conclusion
We developed a model that exposes DNA repair defects
as it predicts hypersensitivity to DNA crosslinking agents
caused by such defects
in vitro
. The model successfully
predicted sensitivity in an independent dataset. We found
that increased probabilities of DNA repair defects were
associated with poorer outcome in patients, possibly a
result of the impact on genomic instability.
Proffered Papers: Highlights of proffered papers
OC-0464 Validation of a fully automatic real-time liver
motion monitoring method on a conventional linac
J. Bertholet
1
, R. Hansen
1
, E.S. Worm
1
, J. Toftegaard
1
, H.
Wan
2
, P.J. Parikh
2
, M. Høyer
1
, P.R. Poulsen
1
1
Aarhus University Hospital, Department of oncology,
Aarhus C, Denmark
2
Washington University- School of Medicine, Department
of Radiation Oncology, St-Louis, USA
Purpose or Objective
Intrafraction motion is a challenge for accurate liver
radiotherapy delivery. Real-time treatment adaptation
(gating, tracking) may mitigate the detrimental effects of
motion, but requires reliable target motion monitoring. In
this study, we develop and validate a framework for fully
automatic monitoring of thoracic and abdominal tumors
on a conventional linac by combining real-time marker
segmentation in kV images with internal position
estimation by an external correlation model (ECM). The
validation is based on experiments and simulations using
known external and internal motion for 10 liver SBRT
patients.
Material and Methods
A fully automatic real-time motion monitoring framework
was developed. The framework combines auto-
segmentation of arbitrarily shaped implanted fiducial
markers in CBCT projections and intra-treatment kV
images with simultaneous streaming of an external optical
motion signal. Fig. A illustrates the workflow: A pre-
treatment CBCT is acquired with simultaneous recording
of the motion of an external block on the abdomen. The
markers are segmented in every CBCT projection and a 3D
voxel model of each marker is generated. The 3D marker
motion is estimated from the observed 2D motion and used
to optimize an ECM of the 3D internal marker motion
INT(t) as a function of the external motion EXT(t). During
treatment, INT(t) is estimated from EXT(t) at 20Hz, while
MV-scatter-free kV images are acquired every 3s during
beam pauses. The markers are segmented in real-time
using the ECM to determine the search area and
projections of the 3D voxel model as templates. The ECM
is continuously updated with the latest estimated 3D
marker position. The method was validated using Calypso-
recorded internal motion and simultaneous camera-
recorded external motion of 10 liver SBRT patients. The
validation included both experiments with a
programmable motion stage and simulations hereof for the
first patient as well as simulations for the remaining
patients. The real-time estimated 3D motion was
compared to the known tumor motion. For comparison,
the position estimation error was also calculated without
ECM updates.
Results
The segmentation rate was 90% with a mean 2D
segmentation error of 1.5pixels. Fig. B compares the
estimated and actual target motion for a portion of the
phantom experiment for Patient 1. The simulations agreed
with the experimental root-mean-square error within
0.4mm (Table 1). For all patients, the mean 3D root-mean-
square error was 1.74mm with ECM updates and 2.47mm
without ECM updates (Table 1).