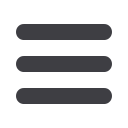

S457
ESTRO 36 2017
_______________________________________________________________________________________________
(gamma=0.7Gy/day), thus taking count of a possible
consequential effect between acute and late damage. The
figure reports calibration plot for all cases.
Conclusion
The study confirms formerly published results on effect of
abdominal surgery and dose to large rectal volumes as
potential risk factors for late FI.
Calibrations highlight a possible role of HF beyond linear
quadratic correction. Inclusion of time recovery
correction improved calibrations, but a further separate
effect of HF was still detectable. This might be related to
the selected alpha/beta (5Gy), which is currently
accepted for late rectal toxicity.
A more suitable value could be found for the longitudinal
definition used in these trials (i.e., toxicity starting in
acute phase and persisting during follow-up), instead of
using the assumption settled in studies focusing on
incidence of late peak events (such as rectal bleeding).
PO-0851 Artificial neural networks for toxicity
prediction in RT: a method to validate their
“intelligence”
E. Massari
1
, T. Rancati
2
, T. Giandini
1
, A. Cicchetti
2
, V.
Vavassori
3
, G. Fellin
4
, B. Avuzzi
5
, C. Cozzarini
6
, C.
Fiorino
7
, R. Valdagni
2
, M. Carrara
1
1
Fondazione IRCCS Istituto Nazionale dei Tumori,
Diagnostic Imaging and Radiotherapy- Medical Physics
Unit, Milan, Italy
2
Fondazione IRCCS Istituto Nazionale dei Tumori,
Prostate Cancer Program, Milan, Italy
3
Cliniche Humanitas-Gavazzeni, Radiotherapy, Bergamo,
Italy
4
Ospedale Santa Chiara, Radiotherapy, Trento, Italy
5
Fondazione IRCCS Istituto Nazionale dei Tumori,
Radiation Oncology 1, Milan, Italy
6
San Raffaele Scientific Institute, Radiotherapy, Milan,
Italy
7
San Raffaele Scientific Institute, Medical Physics, Milan,
Italy
Purpose or Objective
Artificial neural networks (ANNs) were used in the last
years for the development of models for the prediction of
radiation-induced toxicity following RT. In fact, ANNs are
powerful tools for pattern classification in light of their
ability to model extremely complex functions and huge
numbers of data. However, their major counterpoint is
that in some specific cases they might not deliver realistic
results due to their missing critical capacity. The objective
of this study was to develop a method for assessing
reliability of ANNs response over the entire range of
possible input variables. In particular, in this study the
method was applied to the selection of an ANN for the
prediction of late faecal incontinence (LFI) following
prostate cancer RT.
Material and Methods
The analysis was carried out on 664 patients (pts) of two
multicentre trials. The following information was
available for each pt: i) self completed pt reported
questionnaire (PRO) for LFI determination, ii) clinical data
(co-morbidity, previous abdominal surgery and use of
drugs), iii) dosimetric data (DVH and mean dose).
Several feed-forward ANNs with a proper balance between
complexity and number of training cases were developed,
with input variables and hidden neurons ranging between
3 and 5. Once the best ANNs were obtained, a method was
developed and applied to verify the reliability of their
response over the entire range of possible input variables.
The method consists in the development of a virtual
library of variables covering all the possible
ranges/permutations of continuous/discrete inputs. These
are all classified and penalties (pen) are assigned if ANN
outputs are not coherent with the real world expectance
(i.e., decreasing LFI probability with increasing dose to
the rectum).
Results
More than 1,000,000 different ANN configurations (i.e.,
architecture and internal weights and thresholds) were
developed. For the 200 ANNs showing the best
performance, area under the ROC curve (AUC), sensitivity
(Se), specificity (Sp) and pen were quantified. The best
ANN in terms of classification capability (i.e. AUC=0.79,
Se=74%, Sp=72%) was an ANN with 5 inputs (i.e., mean
dose, use of antihypertensive, previous presence of
haemorrhoids, previous colon disease, hormone therapy)
and 5 hidden neurons. However, the application of the
method to investigate its coherence with the real life
classification expectancy resulted in pen=3, indicating
that this wasn’t the most 'intelligent” ANN to select. The
best ANN with pen=0 was a less complex ANN (i.e. 3 inputs,
5 hidden neurons), resulting in AUC=0.67, Se=70%, Sp=57%.
Conclusion
A new method consisting in the development of a virtual
library of cases was established to evaluate ANN reliability
after its training process. Application of this method to
the development of an ANN for LFI prediction following
prostate cancer RT allowed us to select an ANN with the
best generalization capability.
PO-0852 External validation of a TCP model predicting
PSA relapse after post-prostatectomy Radiotherapy
S. Broggi
1
, A. Galla
2
, B. Saracino
3
, A. Faiella
3
, N. Fossati
4
,
D. Gabriele
5
, P. Gabriele
2
, A. Maggio
6
, G. Sanguineti
3
, N.
Di Muzio
7
, A. Briganti
4
, C. Cozzarini
7
, C. Fiorino
1
1
IRCCS San Raffaele Scientific Institute, Medical Physics,
Milano, Italy
2
Candiolo Cancer Center -FPO- IRCCS, Radiotherapy,
Candiolo Torino, Italy
3
Regina Elena National Cancer Institute, Radiotherapy,
Roma, Italy
4
IRCCS San Raffaele Scientific Institute, Urology, Milano,
Italy
5
University of Sassari, Radiotherapy, Sassari, Italy
6
Candiolo Cancer Institute -FPO- IRCCS, Medical Physics,
Candiolo Torino, Italy