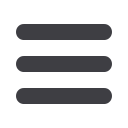

S458
ESTRO 36 2017
_______________________________________________________________________________________________
7
IRCCS San Raffaele Scientific Institute, Radiotherapy,
Milano, Italy
Purpose or Objective
A Poisson-based TCP model of 5-year biochemical
recurrence-free survival (bRFS) after post-prostatectomy
radiotherapy (RT) was previously introduced: best
parameters values were obtained by fitting a large (n=894
≥pT2, pN0, hormone-naïve patients) multi-centric
population including data from five prospective /
Institutional series; a satisfactory internal validation was
performed. Current investigation dealt with an
independent external validation on a large group of
patients pooled from two independent Institutional
databases with a minimum follow-up of 3 years.
Material and Methods
Based on the original model, bRFS may be expressed as: K
x (1-exp(-αeff D))
CxPSA
where: D is the prescribed dose; αeff
is the radiosensitivity factor; C is the number of clonogens
for pre-RT PSA=1ng/ml, assuming PSA to be proportional
to tumor burden; K (equal to 1-BxPSA) is the fraction of
patients who relapse due to clonogens outside the treated
volume, depending on pre-RT PSA and Gleason Score (GS).
The model works well when grouping patients according
to their GS value: best-fit values of αeff (range: 0.23-
0.26), C (10
7
) and B (0.30-0.50) were separately derived
for patients with GS<7, GS=7 and GS>7. For current
external validation, data of 352 ≥pT2, pN0, hormone-naïve
patients treated with conventionally fractionated
adjuvant (175) or salvage (177) intent after radical
prostatectomy were available from two Institutions not
previously involved in the training data set analysis. The
predicted risk of 5-year bRFS was calculated for each
patient, taking into account the slope and off-set of the
model, as derived from the original calibration plot. Five-
year bRFS data were compared against the predicted
values in terms of overall performance, calibration and
discriminative power.
Results
The median follow-up time, pre-RT PSA and D were 83
months (range: 36-216 months), 0.28 ng/mL (0.01-9.01
ng/mL) and 70.2Gy (66–80Gy); the GS distribution was:
GS<7: 118; GS=7: 185; GS>7: 49. The performances of the
model were excellent: the calibration plot showed a
satisfactory agreement between predicted and observed
rates (slope: 1.02; R
2
=0.62, Figure 1). A moderately high
discriminative power (AUC=0.68, 95%CI:0.62-0.73) was
found, comparable to the AUC for the original data set
(0.69, 95%CI:0.66-0.73). The predicted 5-year bRFS for the
whole population assessed as the weighted average of the
values referred to the three groups (i.e.: GS<7, =7, >7)
was 67%, compared to an observed 5-year bRFS equal to
68% ± 5% (95%CI). The agreement was slightly worse in the
GS<7 group (70% vs 79% ± 7%) compared to GS=7 (66% vs
66% ± 7%) and GS>7 (62% vs 51% ± 14%).
Conclusion
A comprehensive, radiobiologically consistent Poisson-
based TCP model of the response to post-prostatectomy
RT was validated for the first time on a completely
independent data set. A more extensive validation on a
larger population is actually in progress to further
corroborate its generalizability.
PO-0853 A method for automatic selection of
parameters in NTCP modelling
D. Christophides
1
, A.L. Appelt
2
, J. Lilley
3
, D. Sebag-
Montefiore
2
1
Leeds CRUK Centre and Leeds Institute of Cancer and
Pathology, University of Leeds, Leeds, United Kingdom
2
Leeds Institute of Cancer and Pathology - University of
Leeds and Leeds Cancer Centre, St James’s University
Hospital, Leeds, United Kingdom
3
Leeds Cancer Centre, St James’s University Hospital,
Leeds, United Kingdom
Purpose or Objective
The use of multivariate models in predicting NTCP has the
potential of improving predictive accuracy compared to
univariate models
1
. However the large numbers of clinical
parameters and dose metrics involved can make the
selection of the optimal multivariate model inconsistent
and time consuming.
In this study a genetic algorithm based method is utilised
to automatically generate ordinal logistic regression
models; subsequently the quality of the parameter
selection process is evaluated by comparison with
published results on the same patient cohort
2
.
Material and Methods
A general method for selecting optimal models for
outcome prediction in radiotherapy was developed
(Fig.1). The method was tested on data from 345 rectal
cancer patients, used in a previously published study
2
, to
generate ordinal logistic regression models for the
prediction of acute urinary toxicity during
chemoradiotherapy. Principal component analysis (PCA)
was used to derive principal components (PCs) that
summarise the variance in the DVH data. Overall 25
clinical parameters were considered in the analysis
including demographics, treatment regime, plan
parameters and stage of disease; as well as 8 PCs that
explained >95% of the variance in the DVHs.
Urinary toxicity was categorised as grade 0, 1 and 2≥
cystitis, according to the CTCAE v3.0. The method (Fig.1)
for optimising the models was implemented in Python and
the entire procedure was repeated 100 times, using
bootstrap sampling from the whole data set, to evaluate
the stability of the parameter selection.
Confidence intervals for the Akaike information criterion
(AIC) of the final models selected were estimated using