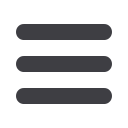

S494
ESTRO 36 2017
_______________________________________________________________________________________________
D. Montgomery
1
, K. Cheng
1
, Y. Feng
1
, D.B. McLaren
2
, S.
McLaughlin
3
, W. Nailon
1
1
Edinburgh Cancer Centre Western General Hospital,
Department of Oncology Physics, Edinburgh, United
Kingdom
2
Edinburgh Cancer Centre Western General Hospital,
Department of Clinical Oncology, Edinburgh, United
Kingdom
3
Heriot Watt University, School of Engineering and
Physical Sciences, Edinburgh, United Kingdom
Purpose or Objective
Prostate cancer is one of the few solid organs where
radiotherapy is applied to the whole organ. This is because
accurately identifying the dominant cancer foci on
magnetic resonance (MR) images, which can then be
mapped onto computerised tomography (CT) images for
radiotherapy planning, is difficult. The aim of this study
was to investigate the use of three-dimensional (3D)
texture analysis for automatically identifying the
dominant cancer foci on MR images acquired for diagnosis
and prior to the administration of androgen deprivation
therapy, which may shrink the tumour foci.
Material and Methods
On 14 patients with confirmed prostate cancer, 3D image
texture analysis was carried out on T2-weighted MR
images acquired for diagnosis on a Symphony 1.5T scanner
(Siemens, Erlangen, Germany). The prostate, bladder,
rectum and the location of the main cancer foci were
outlined on all images. In 5x5x5 pixel
3
volumes within the
prostate 446 3D texture analysis features were calculated.
These features were used to train an AdaBoost model,
which was used to predict the class of each 5x5x5 region
as either 'prostate” or 'focal lesion.” Morphological
filtering was applied to each region to remove invalid
elements and to clean the final outline. The Dice similarity
coefficient was used to assess the agreement between the
clinical and predicted contours.
Results
Figure 1 shows an example of a contour produced by the
algorithm where the Dice similarity coefficient was 0.98.
Table 1 shows the Dice coefficients calculated between
the clinical contours and the contours predicted by 3D
image analysis. 11 of the 14 cases had a Dice score greater
than 0.65 and 8 of the 14 cases had a score greater than
0.9, indicating good agreement between the clinical and
predicted contours. In 3 cases the image analysis
technique failed to identify the focal lesion.
Figure 1
: Clinical contour in blue and predicted contour
generated by 3D texture analysis shown in red on three
T2-weighted MR images from the same patient (Patient 6).
Table 1
: Dice coefficient between the clinical contours
and the contours predicted by image analysis.
Conclusion
The 3D image analysis results presented are encouraging
and demonstrate the potential of this approach for
automatically identifying focal disease on T2-weighted MR
images. However, further investigation is required to
establish why the approach fails in certain circumstances
and to establish the performance of the approach on a
much larger patient cohort.
PO-0903 Patient-induced susceptibility effects
simulation in magnetic resonance imaging
J.A. Lundman
1
, M. Bylund
1
, A. Garpebring
1
, C.
Thellenberg Karlsson
1
, T. Nyholm
1
1
Umeå University, Department of Radiation Sciences,
Umeå, Sweden
Purpose or Objective
The role of MRI is increasing in radiotherapy. A
fundamental requirement for safe use of MRI in
radiotherapy is geometrical accuracy. One factor that can
introduce geometrical distortion is patient-induced
susceptibility effects. This work aims at developing a
method for simulating these distortions. The specific goal
being to objectively identify a balanced acquisition
bandwidth, keeping these distortions within acceptable
limits for radiotherapy.
Material and Methods
A simulation algorithm based on Maxwell’s equations and
calculations of shift in the local B-field was implemented
as a dedicated node in Medical Interactive Creative
Environment (MICE), which is available as a free
download. The algorithm was validated by comparison
between the simulations and analytical solutions on digital
phantoms. Simulations were then performed for four body
regions using CT images for eight prostate cancer patients.
For these patient images, CT Hounsfield units were
converted into magnetic susceptibility values for the
corresponding tissues, and run through the algorithm.
Figure 1: Simulated normalized local B-field for one of the
patients [ppm].
Results
The digital phantom simulations showed good agreement
with analytical solutions, with only small discrepancies
due to pixelation of the phantoms. For a bandwidth of 440
Hz at 3 T, the calculated distortions in the patient-based