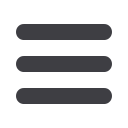

S490
ESTRO 36 2017
_______________________________________________________________________________________________
quantitative
image-based
structural
tissue
characterization was performed.
Material and Methods
T2-weighted and T1-weighted MRI after contrast agent
(CA) injection at 1.5T were acquired in thirteen patients
before RT (MRI1) and at about 12 months of follow-up
(MRI2). In order to reduce possible errors due to non-
quantitative values of signal intensity, a normalization
step was performed between MRI1 and MRI2 of each
patient, using a histogram matching method.
Right and left internal obturator muscle contours were
manually delineated upon T2w MRI1 by an expert and then
automatically propagated on MRI2 by an elastic
registration method.
The following textural features were extracted in each
volume: histogram-based indices (mean intensity,
variance, 95
th
percentile, entropy, skewness, kurtosis),
GLCM (Grey-Level Co-occurrence Matrix)-based indices
(energy, correlation, homogeneity, entropy, contrast,
dissimilarity), NGTDM (Neighborhood Grey-Tone Different
Matrix)-based indices (coarseness, contrast, busyness,
complexity, strength) and fractal dimension.
To assess changes in internal obturator muscles, a
comparison of the parameters extract on MRI1 and MRI2
was carried out by Wilcoxon test, with significant p-value
< 0.05.
Results
Exemplificative T1w MRI1 and MRI2 with relative muscles
histograms were shown in Figure 1.
From a qualitative
assessment, a homogenous higher enhanced area (red
circle in Figure 1) was localized in MRI2 in a region near
the prostate.
Quantitatively, significant increase in mean, variance and
95
th
percentile values on both T1w MRI and T2w MRI2 was
also found, as well as variation of indices describing
histogram shape as visible by the histograms reported in
Figure 1.
Moreover, changes of GLCM and NGTDM-based indices
confirmed that the spatial distribution of this intensity
enhancement was concentrated in a homogeneous local
area, as suggested by increased homogeneity and
correlation indices and decreased complexity and fractal
dimension (Table 1).
Conclusion
In patients who underwent RT for prosta te cancer
treatment, an increase in signal intensity of t he internal
obturator muscles was observed. Specifi cally, this
enhancement was concentrated in the area near the
prostate, likely to be included in high dose regions. This
evidence was present both in T2w and T1w post CA
injection MRI and can be compatible with an inflammatory
status that normally follows RT. This inhomogeneous
structural variation may be explained by the spatial dose
distribution. Moreover, correlations with toxicity scores
should be investigated, considering the involvement of the
pelvic floor muscles in the urinary dysfunctions.
PO-0897 Atlas-based auto-segmentation of heart
structures in breast cancer patients
R. Kaderka
1
, R. Mundt
1
, A. Bryant
1
, E. Gillespie
1
, B.
Eastman
1
, T. Atwood
1
, J. Murphy
1
1
University of California San Diego, Department of 858-
822-4842, San Diego, USA
Purpose or Objective
Radiation therapy deposited in the heart increases the risk
of ischemic heart disease, and sudden cardiac death.
Reproducible contouring of the heart on CT imaging
represents a critical component of treatment planning,
though the literature demonstrates substantial variability
in contouring among providers. In this study we assess the
accuracy of an atlas-based auto-segmentation approach of
the whole heart and the left anterior descending artery
(LAD).
Material and Methods
We randomly selected a cohort of 38 breast cancer
radiotherapy patients treated between 2014 a nd 2016.
For all patients the whole heart and LAD were manually
contoured according to guidelines published by Feng et al.
(2011). The patients were divided into a training dataset
(N=18), and a test dataset (N=20). We used the training
dataset to create a contouring atlas using commercially