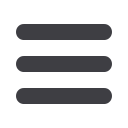

S549
ESTRO 36 2017
_______________________________________________________________________________________________
was observed in area of Hippocampus (less than 5 mm).
Conclusion
Conclusion
: With complete radiological diagnosis, HA-
WBRT can be delivered with oncological safety. Proper
margin definition of HA in delineation is to be confirmed
with individual technique.
PO-1004 Machine learning methods for automated
OAR segmentation
P. Tegzes
1
, A. Rádics
1
, E. Csernai
1
, L. Ruskó
2
1
General Electric, Healthcare, Budapest, Hungary
2
General Electric, Healthcare, Szeged, Hungary
Purpose or Objective
Manual contouring of organs at risk can take significant
time. The aim of this project is to use machine learning to
develop fully automated algorithms to delineate various
organs in the head and neck region on CT images.
Material and Methods
Machine learning models were built based on 48 CT
sequences of the head and neck region with 5 manually
contoured organs from the Public Domain Database for
Computational Anatomy. Data were randomly separated
to 32 train, 8 cross-validation and 8 test sequences. Three
different machine learning models were combined to
achieve automated segmentation. The first step uses a
support vector machine classifier to separate patient
anatomy from all other objects (a). The second step
applies slice-based deep learning classification to detect
the bounding box around the organ of interest (b). The
final step achieves voxel-level classification based on a
fully connected neural net on the voxel intensities of
suitably selected neighboring voxels (c). Very similar
model architectures were trained for all the different
organs.
Results
The body contour detection has been
previously trained
on another dataset containing full-body images and
achieved an average accuracy of 96.6%. The mean error of
the bounding box edges was 3mm, the corresponding dice
scores ranged from 72% to 94% depending on the organ of
interest. The first results of the voxel level segmentation
gave average dice values of 38% to 77% depending on the
investigated organ, and several opportunities for further
fine-tuning have been identified.
Conclusion
Machine learning methods can be competitive with
standard image processing algorithms in the field of organ
segmentation.
PO-1005 Automatic segmentation of cardiac sub-
structures in the treatment of HL
C. Fiandra
1
, M. Levis
1
, F. Cadoni
1
, V. De Luca
1
, F.
Procacci
1
, A. Cannizzaro
1
, R. Ragona
1
, U. Ricardi
1
1
University of Torino, Oncology, Torino, Italy
Purpose or Objective
to validate, in the context of treatment of Hodgkin
Lymphoma, three commercial software solutions for atlas-
based segmentation of cardiac sub-structures
Material and Methods
25 patients were selected and then divided into two
groups: 15 patients will make up the personalized atlas
and 10 patients on which will be applied atlases created
in order to assess its quality. For the selection of patients,
the following inclusion criteria were selected: patients
with HL presentation of a mediastinal mass at the onset of
the disease and the availability of CT imaging with
contrast. Two expert physicians have delineated on the
diagnostic CT with contrast the selected 15 patients
cardiac structures: the heart as a whole, the four
chambers of the heart, the coronary artery and valvular
structures; which will compose the atlas. We use three
commercial solutions (Velocity AI, MIM and RayStation) in
order to compare their results; the structures delineated
by doctors on the 5 control patients will be compared with
those automatically drawn by atlases, through the
conformality function (Dice Index (DI)). In addition, the
atlases underwent a clinical evaluation of the involved
physicians: in particular it was asked to a Radiation
Oncologist to analyze contours made by the three
software on reference patients to evaluate the goodness
of the warp made from atlases than those performed by
him. Clinical judgments were recorded on a scale of
numerical values: 1 = poor; 2 = medium; 3 = good.
Results
in terms of statistical analysis, the data obtained from the
values of Dice Index were compared structure by structure
between the three platforms. The Figure 1 shows only
structures with a Dice Index more than 0.5 (right atrium,
left atrium, the heart, the left side wall, interventricular
septum, aortic valve, left ventricle and right ventricle).
The differences between the 3 software were calculated
and the structures delineated by MIM have more
frequently higher values of Dice Index, compared to those
of Velocity and RayStation, with respectively 0.03 to 0.01
p-value. Instead the difference between Velocity and
RayStation is not statistically significant (p-value = 0.8).
Regarding the evaluation of the Radiation Oncologist as
compared to DI, values show that RayStation is the
software that realizes contours more applicable in clinical
practice, with statistically significant differences from
Velocity and MIM, with p-value respectively of 0.038 and
0.046. While the difference between Velocity and MIM is
not statistically significant (p-value = 0.083).
Conclusion
In general we can say that the contours applied by atlases
are valid, even if not yet optimal and they may represent
a starting point for the step of contouring, useful to speed
up this process; based on the values of Dice Index
collected in this study, MIM performs better while
RayStation appears the most powerful software from a
clinical point of view thus obtaining contours more