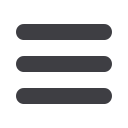

INFORMS Philadelphia – 2015
117
SC72
72-Room 203A, CC
Panel Discussion: IoT-enabled Data Analytics:
Opportunities, Challenges and Applications
Sponsor: Quality, Statistics and Reliability
Sponsored Session
Chair: Kaibo Liu, Assitant Professor, UW-Madison, 1513 University
Avenue, Madison, 53706, United States of America,
kliu8@wisc.edu1 - Panel Discussion: loT-enabled Data Analytics: Opportunities,
Challenges and Applications
Moderator: Kaibo Liu, Assitant Professor, UW-Madison, 1513
University Avenue, Madison, 53706, United States of America,
kliu8@wisc.edu, Panelists: Benoit Montreuil, George Q. Huang,
Soundar Kumara, Diego Klabjan
The goal of this session is to push the frontier in IoT application and the enabled
data analytics research. The session provides a forum where participants can
describe current research, identify important problems and areas of application,
explore emerging challenges, and formulate future research directions.
SC73
73-Room 203B, CC
Quality Engineering
Sponsor: Quality, Statistics and Reliability
Sponsored Session
Chair: Trevor Craney, Shell, Houston, TX, United States of America,
Trevor.A.Craney@shell.com1 - Integrated Approach for Field Reliability Prediction Based on
Accelerated Life Testing
Mingxiao Jiang, Medtronic, 7000 Central Ave NE, Fridley,
United States of America,
mingxiao.jiang@medtronic.comTo predict field reliability using analytic modeling, several important reliability
activities should be conducted, including FMEA, stress and usage condition
analysis, PoF, ALT, and cumulative damage modeling if needed. This paper builds
an integrated process and comprehensive modeling framework, especially with
cumulative damage rules when the certain field stresses are random processes. An
engineering product is provided as an application of proposed method.
2 - The Constant Shape Parameter Assumption in
Weibull Regression
Steve Rigdon, Professor, Saint Louis University, 3545 Lafayette
Ave, Salus 481, Saint Louis, MO, 63104, United States of
America,
srigdon@slu.edu,Georgia Mueller
The usual assumption in Weibull regression is that the scale parameter is a
function of the predictor variables and the shape parameter is constant. We
consider the problem of estimating parameters in the presence of a nonconstant
shape parameter and the effect of assuming a constant shape parameter when it
really isn’t constant. The misspecification of a constant shape parameter leads to
loss of power for tests of the slope parameters and inaccurate prediction intervals.
3 - Model Specification and Confidence Intervals for
Voice Communication
Sara Wilson, NASA Lnagley Research Center, Mail Stop 131,
Hampton, VA, 23681, United States of America,
sara.r.wilson@nasa.gov, Robert Leonard, David Edwards,
Kurt Swieringa, Jennifer Kibler
The performance of a system often depends on the accuracy of information
transferred via voice communications. This paper presents a case study from a
human-in-the-loop experiment using a simulated flight environment that
required a complex voice clearance issued by Air Traffic Control to a flight crew.
The lognormal and loglogistic distributions are found to model the time required
for voice communication, and extensive investigation of outliers was performed
to identify procedural anomalies.
4 - Determining Test Sample Size for Reliability Demonstration
Retesting after Product Design Change
Andre Kleyner, Global Reliability Engineering Leader,
Delphi Electronics & Safety, 2151 E. Lincoln Rd, M.S. CTC4E,
Kokomo, IN, 46902, United States of America,
andre.v.kleyner@delphi.com, David Elmore, Benzion Boukai
Last minute design changes after completed product testing is a common
occurrence. It is also common for a redesigned product to be retested to
demonstrate compliance to the original reliability requirements. This paper
discusses the application of Bayesian techniques to reduce the sample sizes
required for retesting after design change. The proposed method helps to reduce
the test sample size while demonstrating the required reliability and helping to
reduce the cost of product development.
SC74
74-Room 204A, CC
IEEE Intelligent Systems Invited Panel Discussion
on Healthcare Intelligence
Sponsor: Quality, Statistics and Reliability
Sponsored Session
Chair: Hui Yang, Associate Professor, Pennsylvania State University, 310
Leonhard Building, Industrial and Manufacturing Eng., State College,
PA, 16801, United States of America,
huy25@psu.edu1 - Panel Discussion on Healthcare Intelligence:
Turning Data into Knowledge
Moderator: Hui Yang, Associate Professor, Pennsylvania State
University, 310 Leonhard Building, Industrial and Manufacturing
Eng., State College, PA, 16801, United States of America,
huy25@psu.edu,Panelists: W. Art Chaovalitwongse,
Kwok Leu Tsui, Jing Li, Oguzhan Alagoz
This panel brings a panel of distinguished experts to share their perspectives and
answer questions pertaining to data sciences, operation research and healthcare
research. Panelists are: Dr. TSUI Kwok Leung, City University of Hong Kong; Dr.
Oguzhan Alagoz,University of Wisconsin-Madison; Dr. W. Art Chaovalitwongse,
University of Washington; Dr. Jing Li; Arizona State University.
SC75
75-Room 204B, CC
Advanced Manufacturing Systems and Planning
Cluster: Advanced Manufacturing
Invited Session
Chair: Jun-Qiang Wang, Professor, Northwestern Polytechnical
University, Box 554, No. 127 West Youyi Road, Department of
Industrial Engineering, Xi’an, 710072, China,
wangjq@nwpu.edu.cn1 - Real-time Data Driven Visual Decision Support System
for the Factory Floor
Mohammad Rahdar, Iowa State University, 133 University
Village, Unit F, Ames, IA, 50010, United States of America,
rahdar@iastate.edu, Guiping Hu, Dave Sly, Lizhi Wang
The manufacturing industries face significant challenges in operational planning
due to the uncertainties in demand, lead-time, logistic, etc. This study aims to
improve the efficiency of the production planning system and provide the
visibility of real-time operations. The decision support system can access real-time
data and use the models and analytical techniques to support the manufacturing
decision making.
2 - Data-based Scheduling System for Semiconductor Wafer
Fabrication Facility
Li Li, Professor, Tongji University, No.4800, Cao’an Road,
Shanghai, China,
lili@tongji.edu.cnBased on the analysis of the differences and relations between traditional and
data-based scheduling methods, we propose a data-based scheduling framework
and discuss how to implement it for a semiconductor manufacturing system.
Then we introduce the state-of-the-art research on the key technologies of data-
based scheduling and point out their development trends. Finally, we develop a
data-based scheduling prototype system and also use some examples to
demonstrate the superiority of the system.
3 - Cloud Manufacturing Ecosystem – Scheduling and Evolving
Shengkai Chen, Zhejiang University, School of Mechanical
Engineering, 38# Zheda Road, Hangzhou, China,
372927638@qq.com, Shuiliang Fang, Haoke Peng
In the Cloud Manufacturing Ecosystem, a benign mode and methodology for
massive services schedule is required, in order for the collaboration within the
whole Industry Chain. This paper studied the data of the resources and services,
and modeled the services scheduling problem in the chain. With the Big Data
analysis on the Cloud Platform, optimal assessment/schedule methods were
developed, which could gradually evolve the ecosystem to an optimal situation.
SC75