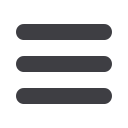

INFORMS Philadelphia – 2015
112
SC57
57-Room 109B, CC
Energy Technology, Climate Change, and Uncertainty
Sponsor: ENRE – Energy II – Other (e.g., Policy, Natural Gas,
Climate Change)
Sponsored Session
Chair: Erin Baker, University of Massachusetts, MIE Department, 220
ELAB, Amherst, MA, United States of America,
edbaker@ecs.umass.edu1 - Equilibrium vs. Optimality: Trading in Renewable
Energy Certificates
Ekundayo Shittu, George Washington University, Washington,
DC, United States of America,
eshittu@email.gwu.edu,
Linus Nyiwul
We propose the harmonization of independent renewable energy credit markets,
and study their impacts on a firm’s energy technology choice and capacity
decisions. The industry is struggling with this issue right now, and we inform this
policy debate by comparing market mechanisms in which each participant
independently maximizes their targets. We find that while there are optimal
market conditions, the equilibrium is not only unstable, the overall efficiency
gains are not always positive either.
2 - Managing Climate Risks with Carbon Mitigation:
A Stochastic Programming Approach with Merge
Delavane Diaz, Stanford University, Huang Engineering Center,
Stanford, CA, 94305, United States of America,
delavane@stanford.edu, Geoffrey Blanford
Carbon policy is fundamentally about risk management – balancing the costs of
reducing emissions and the benefits of avoided climate change, both of which are
uncertain due to incomplete scientific understanding and complex interactions.
This paper presents a framework for decisionmaking under uncertainty in
MERGE, examining optimal carbon mitigation given uncertainty about the
physical climate system and climate damages. This work provides insight into
managing downside risks of climate change.
3 - An Approximate Dynamic Programming Algorithm for Unit
Commitment with Energy Storage
Mort Webster,
mdw18@psu.eduWe present a novel formulation of a stochastic unit commitment model including
energy storage using approximate dynamic programming. We demonstrate that
the non-linear dynamics of energy storage lead to different optimal strategies for
using storage as compared with the typical linear formulation used in most UC
models.
4 - An Approach to Deep Uncertainty in Climate Change: Robust
Portfolio Decision Analysis
Erin Baker, University of Massachusetts, MIE Department,
220 ELAB, Amherst, MA, United States of America,
edbaker@ecs.umass.edu,Valentina Bosetti, Ahti Salo
We advance the concept of Robust Portfolio Decision Analysis and apply it to
analyzing public energy technology R&D portfolios in response to climate change.
We consider 3 sets of expert elicitations over 5 energy technologies. We identify
technology projects that are in all, none, or some of the non-dominated
portfolios, where non-dominated is defined in terms of multiple priors. We
discuss the implications for value of information and for generating new
alternatives with high option value.
SC58
58-Room 110A, CC
Resiliency and Reliability Optimization of Electric
Power Systems
Sponsor: ENRE – Energy I – Electricity
Sponsored Session
Chair: Frank Felder, Associate Research Professor,
Rutgers University, 33 Livingston Ave, New Brunswick, NJ, 08901,
United States of America,
ffelder@rci.rutgers.eduCo-Chair: David Coit, Professor, Rutgers University,
coit@rci.rutgers.edu1 - Long-Term Mitigation for Improved Restoration in
Power Networks
Emily Heath, Graduate Student, Rensselaer Polytechnic Institute,
110 8th St., Troy, NY, 12180, United States of America,
heathe@rpi.edu,Thomas Sharkey, John Mitchell
This research looks at how the best mitigation plan can be selected for a power
network using a ranking and selection procedure. The power system is modeled
using the direct current (DC) model, and a performance measure is developed to
measure how a mitigation plan can contribute to the rapid restoration of the
network following a disruption. We discuss the computational challenges of using
the DC model, and compare results using a flow-based model on the same
network.
2 - Combined Natural Gas and Electric System Operation
with Wind Energy
Dan Hu, Iowa State University, 3004 Black Engineering Bldg,
Ames, IA, United States of America,
danhu@iastate.edu,Sarah Ryan
We formulate a model of a combined natural gas and electric power system
including wind energy. A two-stage stochastic programming model for day-ahead
scheduling is proposed with uncertainty in wind power production. Joint
optimization of gas delivery and electricity production, with the ability to store
natural gas, help to maintain equilibrium in the combined system while meeting
demand with high reliability and low cost.
3 - An Adjustable Robust Optimization Approach to Provision of
Interruptible Load
Qi Zhang, Carnegie Mellon University, 5000 Forbes Avenue,
Pittsburgh, PA, 15213, United States of America,
qi.zhang13@gmail.com,Michael F. Morari, Ignacio E. Grossmann,
Jose M. Pinto, Arul Sundaramoorthy
In modern electricity markets, large electricity consumers can sell operating
reserve by providing capacities to reduce their electricity load upon request.
Providing such interruptible load can be very lucrative; however, one does not
know in advance when load reduction will actually be requested. In this work, an
adjustable robust optimization approach is applied to model this uncertainty,
using affine decision rules that allow recourse decisions in the resulting
scheduling problem.
SC59
59-Room 110B, CC
Just the Facts: Empirical Patterns in Strategy
Cluster: Strategy Science
Invited Session
Chair: Myles Shaver, University of Minnesota, 321-19th Ave S,
Suite 3-365, Minneapolis, MN, 55455, United States of America,
Mshaver@umn.edu1 - How Competition Affects the Governance of R&D Projects:
Evidence from Biotechnology Clinical Trials
Mazhar Islam, Drexel University in Philadelphia, PA
mui27@drexel.eduAlthough almost all biotechnology firms participate in R&D alliances, we
highlight that when one looks at a more micro-level of analysis – drug
compounds within a therapeutic area – the majority of projects are done
internally. Using a unique data set of clinical trials in 24 therapeutic areas in the
U.S. biotechnology industry between 1996 and 2008, we show that biotechnology
firms prefer internal organization absent competition from other biotechnology
firms in the therapeutic area. With greater competition, we observe that these
firms are more likely to utilize non-equity alliances compared to internal
development - presumably to speed time to market within a competitive arena.
We present two contingencies that aid in identifying the mechanism underlying
this empirical finding – scope of applicability of the drug compound and the
biotechnology firm’s previous success with drug development projects.
2 - Innovation and Competition among Different Size FIrms
Siddharth Sharma, PhD Candidate, Strategic Management,
Robert H. Smith School of Business, University of Maryland, MD,
United States of America,
siddharth@rhsmith.umd.edu,Wilbur Chung
We examine the Consumer Electronics trade show (CES) as a microcosm of
competitive interaction among different size firms. In this dense space, firms seek
to position their booths to maximize exposure during this punctuated event.
While industry heavy weights occupy key spots and little known ones are in the
periphery, we still observe that firms of quite different sizes can be neighbors. We
expect smaller firms armed with an innovation to seek out larger firms. We
develop a simulation model with different size firms that have differing
probability and value of innovations. Once their innovation draw is known, firms
chose where to locate on a two-dimensional space with heterogeneous demand
and look to maximize their demand. Firms compete by locating adjacent to others
to capture some of their neighbors’ demand. But locating with others can also
generate externalities – agglomeration economies – that may offset competition.
We compare the simulation’s predictions versus actual booth locations. The
setting and resulting simulation provide insights into the competitive dynamics
underlying industry evolution.
SC57