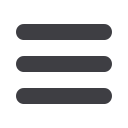

INFORMS Philadelphia – 2015
123
3 - Parallel Algorithms for MIP Feasibility
Lluís Miquel Munguía, Georgia Institute of Technology, 266 Ferst
Drive, Room 1343, Atlanta, GA, 30332, United States of America,
lluis.munguia@gatech.edu,Shabbir Ahmed, David A. Bader,
George L. Nemhauser, Yufen Shao
We present highly parallelizable methods for obtaining feasible solutions to Mixed
Integer Programming (MIP) instances. The use of several relaxations allows us to
leverage parallelism to successfully obtain feasibile solutions to large-scale
instances. We give computational results that demonstrate the effectiveness of the
parallel algorithms.
4 - Facets for Continuous Multi-mixing Set with General Coefficients
and Bounded Integer Variables
Kiavash Kianfar, Associate Professor, Texas A&M University,
TAMU 3131, College Station, TX, 77843-3131,
United States of America,
kianfar@tamu.edu,Manish Bansal
Bansal and Kianfar developed facet-defining inequalities for continuous multi-
mixing set where the coefficients satisfy certain conditions. We first generalize
their inequalities for the continuous multi-mixing set with general coefficients
and show that they are facet-defining in many cases. Next, we introduce a family
of valid inequalities for the continuous multi-mixing set with general coefficients
and bounded integer variables, and investigate their facet-defining properties.
SD12
12-Franklin 2, Marriott
Strong Relaxations and Computations for Mixed
Integer Nonlinear Programs
Sponsor: Optimization/Mixed Integer Nonlinear Optimization and
Global Optimization
Sponsored Session
Chair: Jeff Linderoth, University of Wisconsin-Madison, 1513
University Avenue, Madison, WI, 53706-1572,
United States of America,
linderoth@wisc.edu1 - Convex Hull of Two Quadratic or a Conic Quadratic and a
Quadratic Inequality
Sina Modaresi, Discover/University of Pittsburgh, 2402 W
Beardsley Road, Phoenix, AZ, United States of America,
sim23@pitt.edu, Juan Pablo Vielma
We consider an aggregation technique introduced by Yildiran [2009] to study the
convex hull of regions defined by two quadratic or by a conic quadratic and a
quadratic inequality. We show how this technique can be used to yield valid conic
quadratic inequalities for the convex hull of sets defined by two quadratic or by a
conic quadratic and a quadratic inequality. We also show that in many cases,
these valid inequalities characterize the convex hull exactly.
2 - Relaxations and Heuristics for the General Multiple Nonlinear
Knapsack Problem
Luca Mencarelli, CNRS LIX, Ecole Polytechnique, Palaiseau,
91120, France,
mencarelli@lix.polytechnique.fr,
Claudia D’ambrosio, Silvano Martello
We consider the multiple mixed-integer nonlinear knapsack problem. These
problems are very difficult to solve, both from a theoretical and a practical
viewpoint. We analyze different relaxations and extend known theoretical results
for the multiple linear knapsack problem. Moreover, we propose fast constructive
heuristic algorithms and a local search procedure.
3 - Recent Advances in CPLEX for Mixed Integer
Nonlinear Optimization
Pierre Bonami, IBM, Santa Hortensia 26, Madrid, Spain,
pierre.bonami@es.ibm.com, Andrea Tramontani
We present some of the new algorithmic techniques that have been recently
added to the IBM CPLEX solver to address nonlinear optimization models. We
focus in particular on mixed integer second order cone programming and
quadratic optimization. We present extensive computational analysis to assess the
performance gain from these techniques.
4 - Strong Convex Nonlinear Relaxations of the Pooling Problem
Jeff Linderoth, University of Wisconsin-Madison, 1513 University
Avenue, Madison, Wi, 53706-1572, United States of America,
linderoth@wisc.edu, Jim Luedtke, Claudia D’ambrosio
We investigate convex relaxations for the pooling problem. We characterize the
extreme points of the convex hull of our non-convex set, and we derive valid
nonlinear convex inequalities. Computational results demonstrate that the
inequalities can significantly strengthen the convex relaxation of even the most
sophisticated formulations of the pooling problem.
SD13
13-Franklin 3, Marriott
Uncertainty in Energy and Natural Resource Systems
Sponsor: Optimization/Optimization Under Uncertainty
Sponsored Session
Chair: Alexandra Newman, Professor, Colorado School of Mines,
Mechanical Engineering, Golden, CO, 80401, United States of America,
anewman@mines.edu1 - Complementarity Effects Between Wind and Hydro in the Context
of Power Generation Scheduling
Anderson Rodrigo De Queiroz, Assistant Professor, Federal
University of Itajubá, 1303 BPS Avenue, Itajubá, MG, 37500000,
Brazil,
ar_queiroz@yahoo.com.br, Saulo Ribeiro Silva,
Luana Marangon Lima
We present a computational model able to determine the optimal economic
generation scheduling in a system with hydro, thermal and wind power plants.
Our model takes into account the stochastic nature of wind speed and its
complementarity with water inflows. We use sampling-based decomposition
algorithms to solve such model and present results for a case study. Our analysis
suggests that complementary is relevant and should be taken into consideration
during the scheduling of generators.
2 - Global Thermal Coal Optimization under Uncertainty
Ashley Arigoni, PhD Candidate, Colorado School of Mines,
1500 Illinois St, Golden, CO, 80401, United States of America,
aarigoni@mymail.mines.eduOur objective is to minimize the cost to ship thermal coal to global fill demand
while respecting import and export port capacities, ship size constraints, and coal
specification requirements under spot market price uncertainty. Coal blending is
allowed at demand nodes to meet coal specification requirements. We develop a
two-stage stochastic model in which forward purchases are made before spot
market uncertainties are realized.
3 - Mitigating Uncertainties with Stochastic Decomposition:
Applications in Operations Management.
Yifan Liu, University of Southern California, 3715 McClintock
Ave, GER 240, Los Angeles, CA, 90089, United States of America,
yifanl@usc.edu,Suvrajeet Sen
Uncertainties are common in operation and production management. In this talk,
we will discuss applying stochastic decomposition for solving a wide range of
operations management applications including single period multi-products
inventory problem, multi-location transhipment problem and process flexibility
design problem. The first two problems are modeled as two-stage stochastic linear
programs while the last one contains first stage binary variables.
4 - Quantifying Uncertainty in an Optimization Model using
Exponential EPI-spline Density Estimation
Michael Teter, Ltc, Colorado School of Mines, Golden, CO, 80401,
United States of America,
mteter@mymail.mines.eduUsing a capital budgeting optimization model, we explore fusing hard and soft
information through constrained nonparametric density estimation in order to
quantify the uncertainty of the future. A comparison of solutions from data sets
derived from traditional distributions and those derived from epi-splines
demonstrate the benefits of nonparametric density estimation. These solutions
prescribe a set of technologies in which the U.S. Army should invest to maximize
effectiveness.
SD14
14-Franklin 4, Marriott
Topics in Dynamic Programming
Sponsor: Optimization/Optimization Under Uncertainty
Sponsored Session
Chair: Francesca Maggioni, Assistant Professor, University of Bergamo,
Via dei Caniana n 2, Bergamo, 24127, Italy,
francesca.maggioni@unibg.it1 - ADP for Risk-Averse Markov Decision Processes using Dynamic
Quantile-Based Risk Measures
Daniel Jiang, Princeton University, Sherrerd Hall,
Charlton Street, Princeton, NJ, 08540, United States of America,
drjiang@princeton.edu, Warren Powell
We consider Markov decision process (MDP) for which the objective is to
minimize a quantile-based risk measure of the sequence of future costs. In
particular, we consider dynamic risk measures constructed using the one-step
quantile (VaR) and the one-step conditional value at risk (CVaR). We propose
simulation-based approximate dynamic programming (ADP) algorithms, modeled
after Q-learning, and apply the algorithms in the context of an application in the
energy market.
SD14