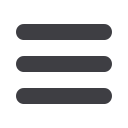

INFORMS Philadelphia – 2015
125
3 - Evacuation Modeling and Betweenness Centrality
Chrysafis Vogiatzis, Assistant Professor, North Dakota State
University, 1410 14th Avenue North, Room 202 Civil & Industrial
Engineering, Fargo, ND, 58102, United States of America,
chvogiat@ufl.eduDisaster management and evacuation modeling are vital for the welfare of
modern urban societies. However, it is true that evacuating large urban areas is a
very difficult problem due to the large scale of operations and the dynamic nature
of most hazard phenomena. In this talk, we present two novel islanding
techniques with the goal of decomposing large-scale network problems to smaller
ones of manageable size. Computational results are also presented to show the
success of our methodologies.
4 - Variable Versus Fixed Congestion Pricing under Day-to-Day
Traffic Dynamics
Zhengtian Xu, University of Florida, 511 Weil Hall, Gainesville,
FL, 32611, United States of America,
zhengtianxu@ufl.edu,
Yafeng Yin
This paper compares the effectiveness of fixed and variable congestion pricing on
evolving network flows to a target flow distribution under day-to-day traffic
dynamics. Fixed pricing charges constant tolls while variable pricing updates tolls
every day based on recent days’ traffic conditions. The paper proves that the latter
does not necessarily perform better than the former and provides conditions
when this situation arises. Numerical experiments are presented to demonstrate
the comparison.
5 - Vulnerability Modeling and Analysis of Complementary
Transportation Systems
Liu Hong, Associate Professor, Huazhong University of Science
and Technology, Room W.308, South 1,1037#, Luoyu Road,,
Wuhan, China,
liu.hong.science@gmail.com, Min Ouyang,
Xiaozheng He
This paper proposes a network-based approach to model and analyze the
vulnerability of complementary transportation systems, with main focus on
quantifying their complementary strength, assessing dynamic vulnerability and
discussing critical components. Two level complementary systems are used to
illustrate the tractability and effectiveness of the proposed method, including
railway and airline system in China (national level), metro and bus system in
Wuhan city in China (Urban level).
SD18
18-Franklin 8, Marriott
Theory and Applications of Coordinate Descent and
Alternating Direction Methods
Cluster: Modeling and Methodologies in Big Data
Invited Session
Chair: Brendan Ames, Assistant Professor, University of Alabama,
Department of Mathematics, Box 870350, Tuscaloosa, AL, 35487,
United States of America,
bpames@ua.edu1 - Block Coordinate Stochastic Gradient Method
Yangyang Xu, University of Minnesota, Institute of Math and
Application, Minneapolis, MN, United States of America,
xuyang.gucas@gmail.com, Wotao Yin
Stochastic gradient (SG) can quickly solve a problem with many components in
the objective to a moderate accuracy, and block coordinate descent (BCD) method
can quickly solve problems with multiple (blocks of) variables. In this talk, we
will introduce a block stochastic gradient (BSG) method that combines SG and
BCD for problems with many components in the objective and with multiple
blocks of variables. We will show its convergence and demonstrate its superiority
over SG and BCD.
2 - Proximal Methods for Sparse Discriminant Analysis
Brendan Ames, Assistant Professor, University of Alabama,
Department of Mathematics, Box 870350, Tuscaloosa, AL, 35487,
United States of America,
bpames@ua.eduWe consider the problem of simultaneously performing classification and feature
selection in the high-dimension, low sample size setting, where the number of
features of our data is large while the number of samples is limited. In particular,
we propose an alternating direction method for performing optimal scoring-based
linear discriminant analysis with a sparseness criterion, where proximal gradient
methods are used to update the decision variables in the alternating direction
framework.
3 - Iteration Complexity Analysis of Block Coordinate
Descent Methods
Mingyi Hong, Iowa State University, 3015 Black Engineering,
Ames, IA, 50011, United States of America,
mingyi@iastate.eduWe provide a unified iteration complexity analysis for a family of block coordinate
descent methods. We unify these algorithms under the so-called Block Successive
Upper-bound Minimization framework, and show that they achieve a global
sublinear iteration complexity. Moreover, for the case of block coordinate
minimization where each block is minimized exactly, we establish the sublinear
convergence rate of O(1/r) without per block strong convexity assumption.
4 - Self Equivalence of the Alternating Direction Method of Multipliers
Ming Yan, Assistant Professor, Michigan State University,
220 Trowbridge Rd, East Lansing, MI, 48824, United States of
America,
yanm@math.ucla.eduIn this talk, we show that ADM applied to a primal formulation is equivalent to
ADM applied to its Lagrange dual; ADM is equivalent to a primal-dual algorithm
applied to the saddle-point formulation of the same problem. In addition, when
one of the two objective functions is quadratic, we show that swapping the
update order of the two primal variables in ADM gives the same algorithm.
SD19
19-Franklin 9, Marriott
Computational Data Analytics
Sponsor: Computing Society
Sponsored Session
Chair: Dorit Hochbaum, University of California, IEOR, “Etcheverry
Hall, Berkeley, Ca, 94720, United States of America,
dhochbaum@berkeley.edu1 - Improved Algorithms Solving Generalizations of Isotonic
Median Regression
Cheng Lyu, University of California, 4141 Etcheverry Hall,
Berkeley, CA, 94720, United States of America,
chenglu@berkeley.edu, Dorit Hochbaum
Isotonic median regression (IMR) problem is a well-known statistical
nonparametric regression problem. We address here generalizations of IMR for
which we devise efficient algorithms. Our algorithms improve on the best
previously known complexity for the special case of IMR. Some applications of
independent interest of the generalized problems include total variation on a
path, signal processing, and nearly-isotonic regression.
2 - Big Data Aggregation and Truncation by
Pseudo-boolean Polynomials
Boris Goldengorin, C. Paul Stocker Visiting Professor, Ohio
University, 283 Stocker Center, Athens, OH, United States of
America,
goldengorin@gmail.comIn this talk we show how to aggregate and truncate the numerical data in huge m
(rows) times n (columns) tables by means of ordering the entries within their
columns in a non-decreasing (non-increasing) order. The ordered columns can be
represented by the corresponding permutations and differences between the
neighboring entries. Our computational study shows that for complete networks
containing thousands of vertices we are able to reduce the number of entries by
more than 90%.
3 - Adjacency-clustering for Yield Prediction in Integrated
Circuit Manufacturing (ICM)
Dorit Hochbaum, University of California, IEOR, Etcheverry Hall,
Berkeley, CA, 94720, United States of America,
dhochbaum@berkeley.edu, Sheng Liu
Accurate yield prediction in ICM enables early detection of processing problems.
It is noted that defects tend to be clustered and a circuit likely to be defective if its
neighbors are defective. This Neighborhood Effect NE is not captured in
traditional spatial modeling approaches. We model the yield prediction problem
with NE as a Markov Random Field model (MRF). A comparison with leading
approaches (GLM and GLMM) demonstrates that MRF provides superior accuracy
and time complexity.
SD19