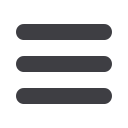

INFORMS Philadelphia – 2015
126
SD20
20-Franklin 10, Marriott
Queueing with Redundancy for Cloud Computing
Cluster: Cloud Computing
Invited Session
Chair: Mor Harchol-Balter, Professor, Carnegie Mellon University,
Computer Science Dept., 5000 Forbes Ave., Pittsburgh,
United States of America,
harchol@cs.cmu.edu1 - Exact Queueing Analysis of Redundancy-d Systems
Mor Harchol-Balter, Professor, Carnegie Mellon University,
Computer Science Dept., 5000 Forbes Ave., Pittsburgh,
United States of America,
harchol@cs.cmu.edu, Kristen Gardner,
Sam Zbarsky, Alan Scheller-wolf
Recent cloud research has proposed using redundant requests to reduce latency
by copying a request to multiple servers and waiting for only one copy to
complete. We study the Redundancy-d system, in which each arriving job sends
copies to d randomly selected servers. We provide the limiting state distribution.
We also derive the exact mean response time in this system for any number of
servers and any degree of redundancy.
2 - Scaling Redundancy to Many-server Systems
Kristen Gardner, PhD Student, Carnegie Mellon University,
Computer Science Dept., 5000 Forbes Ave., Pittsburgh, PA,
15213,
ksgardne@cs.cmu.edu,Mor Harchol-Balter,
Alan Scheller-wolf, Sam Zbarsky
This talk is a continuation of the previous talk on the Redundancy-d system.
Here, we study the system in the limit as the number of servers approaches
infinity. We derive the full response time distribution and use this result to discuss
the effect of the number of copies per job on response time.
3 - Optimal Scheduling of Partially Replicated Jobs
Rhonda Righter, Professor, University of California, Berkeley,
IEOR, UC, Berkeley, CA, 94720, United States of America,
rrighter@berkeley.edu,Mor Harchol-Balter, Esa Hyytia
We consider systems in which there is a mix of tasks that can be replicated and
tasks that cannot. We explore the effect of the amount of replication on latency,
and find the optimal service discipline in the presence of partial replication.
4 - Analysis and Routing in Parallel Queues with
Class-based Redundancy
Leela Nageswaran, PhD Candidate, Carnegie Mellon University,
5000 Forbes Avenue, Pittsburgh, United States of America,
lnageswa@andrew.cmu.edu,Alan Scheller-wolf
We study the performance of two parallel queues when some customers are
redundant: a redundant customer joins both queues and is considered served
when any one of his requests finishes service, instantly removing the other one.
We examine the policy other (non-redundant) customers use to join a queue
upon arrival. We find that while joining the shortest queue does not always
minimize delay if the entire state information is available, it is optimal if only the
queue lengths are observable.
SD21
21-Franklin 11, Marriott
Natural History Modeling for Medical
Decision Making
Sponsor: Health Applications
Sponsored Session
Chair: Julie Higle, Professor And Chair, University of Southern
California, Epstein Dept of Indus & Sys Eng, Los Angeles, CA, 90089,
United States of America,
higle@usc.edu1 - Developing and Validating Markov Decision Processes for
Chronic Diseases
Brian Denton, Associate Professor, University of Michigan,
1205 Beal Ave, Ann Arbor, United States of America,
btdenton@umich.eduChronic diseases are the leading cause of death in many countries including the
United States. Building models for chronic diseases can be challenging because of
the need to characterize severity of the disease, uncertainty in disease
progression, and a potentially large number of strategies for screening and
treatment. In this talk I will discuss my experiences in developing Markov
decision processes (MDPs) for optimization of disease screening and treatment
decisions in several contexts.
2 - Challenges and Opportunities for Developing
Natural History Models
Oguzhan Alagoz, UW-Madison, 3242 Mechanical Engineering
Building, 1513 University Aveneue, Madison, WI, 53706,
United States of America,
alagoz@engr.wisc.eduNatural history of a disease, which represents the onset and progression of a
disease without an intervention, provides critical inputs for operations research
models in health care. In this presentation, we will share our experiences in
developing natural history models for various diseases including end-stage liver
diseases and breast cancer.
3 - Robust Parameter Selection for Natural History Models
Julie Higle, Professor And Chair, University of Southern
California, Epstein Dept of Indus & Sys Eng, Los Angeles, CA,
90089, United States of America,
higle@usc.edu, Suvrajeet Sen
Natural history models are often used to facilitate an understanding of the
potential impact of disease screening and/or treatment options. We consider a
method for calibrating a natural history model that explicitly considers
uncertainties in the calibration targets. The calibration model is designed to yield
a robust parameter selection, especially with respect to medical decisions that
result.
4 - Modeling Ductal Carcinoma in Situ (DCIS)
Shadi Hassan Goodarzi, PhD Student, North Carolina State
University, Fitts Dept of ISE, Raleigh, No, 27695,
United States of America,
shassan3@ncsu.edu, Julie Ivy
Ductal Carcinoma In Situ (DCIS) is arguably a direct precursor of invasive breast
cancer. Approximately 14–53% of DCIS turn into IBC, after long follow-up
periods. So about 47%-86% of the DCIS cases are over diagnosed and as a result,
treatment can only cause harm for these patients. This framework will allow us to
study the progression of DCIS into IBC more clearly and as a result aid both
patients and doctors in decision making.
SD23
23-Franklin 13, Marriott
Queues in Heavy-Traffic: Approximations and Control
Sponsor: Applied Probability
Sponsored Session
Chair: Yunan Liu, Assistant Professor, North Carolina State University,
111 Lampe Drive, #400, Raleigh, No, 27695, United States of America,
yunan_liu@ncsu.edu1 - A Many-Server Heavy-Traffic Limit for the Overloaded
G_t/gi/n+gi Queue
Ahmet Korhan Aras, North Carolina State University, 307 Daniels
Hall, Raleigh, NC, United States of America,
akaras@ncsu.edu,
Yunan Liu, Xinyun Chen, Ward Whitt
We establish a many-server heavy-traffic FCLT for key performance processes
such as potential waiting time, number of abandonment and queue length for the
G_t/GI/n+GI queue in the overloaded regime. We obtain a stochastic differential
equation driven by a Gaussian process in the limit for the scaled waiting time
process. The Gaussian limit and Gaussian integral appear in the limit of the
departure process which is not a Brownian motion when the service distribution
is not exponential.
2 - Diffusion Approximation for Efficiency-driven Queues: A Space-
time Scaling Approach
Shuangchi He, National University of Singapore,
National University of Singapore, Singapore,
heshuangchi@nus.edu.sgUsing a scaling approach in both space and time, we obtain a diffusion model for
the virtual waiting time process in a GI/GI/n+GI queue in the ED regime. Besides
the commonly used scaling in space by the number of servers, we also change the
time scale by using the mean patience time as the factor. This approach leads to a
simple one-dimensional diffusion limit, enabling us to obtain useful performance
formulas such as the distributions of the steady-state virtual waiting time and
queue length.
3 - Non-markovian State-dependent Networks in Critical Loading
Chihoon Lee, Stevens Institute of Technology, Howe School of
Technology Management, Hoboken, NJ, United States of
America,
chihoon@stat.colostate.edu, Anatolii Puhalskii
We establish a heavy traffic limit theorem for the queue-length process in a
critically loaded single class queueing network with state-dependent arrival and
service rates. A distinguishing feature of our model is non-Markovian state
dependence. The limit stochastic process is a continuous-path reflected process on
the nonnegative orthant. We give an application to a generalised Jackson network
with state-dependent rates.
SD20