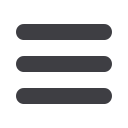

INFORMS Philadelphia – 2015
129
SD30
30-Room 407, Marriott
“Speed Networking” Coordination of
Subdivisions’ Interests
Sponsor: CPMS
Sponsored Session
Chair: Doug Samuelson, InfoLogix, Inc., 8711 Chippendale Court,
Annandale, VA, 22003, United States of America,
samuelsondoug@yahoo.com1 - “Speed Networking” Coordination of Subdivisions’ Interests
Doug Samuelson, InfoLogix, Inc., 8711 Chippendale Court,
Annandale, VA, 22003, United States of America,
samuelsondoug@yahoo.comWe imitate “speed networking” events in which couples spend ten minutes
conversing, then switch partners, allowing for eight or nine such meetings. This
allows subdivision officers to learn about other subdivisions with similar interests,
promote coordination of sessions, reduce schedule conflicts, and possibly
collaborate outside the annual meeting. All subdivision officers are encouraged to
attend and participate. The organizer will arrange pairings, following participants’
preferences.
SD31
31-Room 408, Marriott
Data Analytics and Statistical Learning
Sponsor: Data Mining
Sponsored Session
Chair: Shouyi Wang, Assistant Professor, University of Texas at
Arlington, 3105 Birch Ave, Grapevine, TX, 76051, United States of
America,
shouyiw@uta.edu1 - Co-clustering Based Dual Prediction for Cargo
Pricing Optimization
Yada Zhu, Research Staff Member, IBM, Thomas J. Watson
Research Center, 1101 Route 134 Kitchawan Rd, Yorktown
Heights, NY, 10598, United States of America,
yzhu@us.ibm.comIn the air cargo business, given the features associated with a pair of origination
and destination, how can we simultaneously predict both the optimal price for
the bid stage and the outcome of the transaction (win rate) in the decision stage?
In this paper, we propose a probabilistic framework and a COCOA algorithm to
simultaneously construct dual predictive models and uncover the co-clusters of
originations and destinations.
2 - An Efficient Orthogonal-polynomial-based Approach for Time
Series Representation and Prediction
Shouyi Wang, Assistant Professor, University of Texas at
Arlington, 3105 Birch Ave, Grapevine, TX, 76051,
United States of America,
shouyiw@uta.eduWe present a new efficient time series representation and prediction framework,
called orthogonal-polynomial-based variant-nearest-neighbor (OPVNN)
approach, for complex and highly nonlinear time series data. The proposed
approach achieved the most robust prediction performance compared to the state-
of-the-art time series modeling and prediction methods for the challenging
respiratory motion prediction problem. It has a great potential to handle complex
time series data streams efficiently.
3 - Online Social Network (OSN) Fake Account Detection System with
Cluster Level Features
Danica Xiao, PhD Candidate, University of Washington,
Seattle, 3900 Northeast Stevens Way, Seattle, WA, 98195,
United States of America,
xiaoc@uw.eduMost online social networks (OSN) are often faced with users with undesired
activities during the network’s growth and expansion. Most of them are
malicious. Many of malicious activities start with fake accounts (aka “sybil
accounts”) attack. This paper presents a supervised learning based system to
address such challenge.
4 - Unsupervised Data Mining for Medical Fraud Detection
Tahir Ekin, Assistant Professor, Texas State University, 601
University Dr. McCoy Hall 411, San Marcos, TX, 78666,
United States of America,
t_e18@txstate.edu, Greg Lakomski,
Rasim Muzaffer Musal
U.S. governmental agencies report that three to ten percent of the annual health
care spending is lost to fraud, waste and abuse. These fraudulent transactions
have direct cost implications to the tax-payers, in addition to diminishing the
quality of the medical services. This talk discusses the use of unsupervised data
mining approaches such as latent Dirichlet allocation for medical fraud detection.
Our main objective is to identify the billing behaviors and find providers that are
outliers.
SD32
32-Room 409, Marriott
Computational and Statistical Challenges in Big Data
Genomics
Cluster: Big Data Analytics in Computational Biology/Medicine
Invited Session
Chair: Li-San Wang, Associate Professor, University of Pennsylvania,
423 Guardian Drive, 1424 Blockley Hall, Philadelphia, PA, 19104,
United States of America,
lswang@upenn.edu1 - Big Data Analyses Reveal Many New Short Non-coding RNAs in
Health and Disease
Isidore Rigoutsos, Professor, Computational Medicine Center,
Jefferson Medical College, Thomas Jefferson University,
1020 Locust Street, Suite #M81, Philadelphia, PA, 19108,
United States of America,
isidore.rigoutsos@jefferson.eduBy analyzing transcriptomic datasets from healthy individuals and patients we
have uncovered numerous novel regulatory non-coding RNAs. These molecules
include novel microRNAs, isoforms of microRNAs, fragments of transfer RNAs
(tRNAs), and other. Importantly, we find that these molecules’ composition and
abundances are dependent on an individual’s race, population, and gender as well
as on tissue, tissue state and disease subtype.
2 - Awsomics: A Knowledge Discovery Infrastructure Based on
Annotated Genomic Data
Zhe Zhang, Bioinformatics Scientist, Children’s Hospital of
Philadelphia, 3535 Market Street, Suite 1067, Philadelphia, PA,
19104, United States of America,
zhangz@email.chop.eduKnowledge discovery is adversely lagging behind data and information generation
in the field of genomic research. To assist biomedical researchers to digest the
overwhelming amount of genomic data, we developed a system based on
Amazon Web Service. It includes an archive of curated data and results, various
methods supporting integrative analysis, and a web-based toolbox. It will be a
valuable resource for biomedical researchers to gain novel insights about the
complicated biological systems.
3 - Quality Control of Whole Genome and Exome Data in a Large
Sequencing Study of Alzheimer Disease
Adam Naj, Instructor, Department Of Biostatistics And
Epidemiology, University of Pennsylvania, 423 Guardian Drive,
229 Blockley Hall, Philadelphia, PA, 19104,
United States of America,
adamnaj@mail.med.upenn.eduThe Alzheimer’s Disease (AD) Sequencing Project (ADSP) is an NIH project to
sequence 578 familial genomes and 10,692 unrelated exomes of cases and
controls to identify causal genomic variants. Here we describe extensive
bioinformatics applications in a multi-center quality control effort: performing
genotype calling, integrating data from multiple calling pipelines, filtering low-
quality samples, and incorporating external annotation to facilitate identifying
rare variants affecting AD risk.
SD32