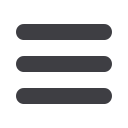

INFORMS Philadelphia – 2015
222
MC50
50-Room 106A, CC
Retail Supply Chain: From Demand Forecast to
Order Fulfillment
Sponsor: Manufacturing & Service Operations Management
Sponsored Session
Chair: Santiago Gallino, Tuck School of Business,
100 Tuck Hall, Hanover, NH, United States of America,
santiago.gallino@tuck.dartmouth.edu1 - How an e-Retailer can Profit from the Right Free Shipping Policy:
A Model and Evidence
Joseph (Jiaqi) Xu, The Wharton School, University of
Pennsylvania, 3730 Walnut Street, Suite 500, Philadelphia, PA,
United States of America,
jiaqixu@wharton.upenn.edu,Gerard Cachon, Santiago Gallino
We present a model of online retail profitability when customers purposely
increase their order size to qualify for free shipping. While this behavior results in
more sales, it also adds cost from less shipping revenue and more product returns.
We find that free shipping threshold often decreases profitability and is effective
only for retailers with high fulfillment cost relative to shipping revenue and with
low probability of return. The model is applied to data from an online retailer.
2 - Can Supply Chain Flexibility Facilitate Information Sharing?
Mohammad M. Fazel-Zarandi, PhD Candidate, Rotman School of
Management, 105 St.George Street, Toronto, M5S 3E6, Canada,
M.FazelZarandi10@Rotman.Utoronto.Ca,Oded Berman,
Dmitry Krass
We attempt to provide an explanation for a long-standing observation in supply
chain management: while simple contracts cannot induce credible forecast
sharing between different supply chain parties, firms often use them in practice,
and exchange information through unverifiable communication. Using a stylized
supply chain model, we show that if the reporting firm is uncertain about the
receiving firm’s reaction to its report, it may truthfully share its private
information in equilibrium.
3 - Improving Color Trend Forecasting using Social Media Data
Youran Fu, PhD Student, The Wharton School,
3730 Walnut St, Philadelphia, PA, United States of America,
youranfu@wharton.upenn.edu,Marshall Fisher
We partnered with a leading apparel retailer to investigate how to use social
media data to improve fashion color trend forecasting. We find that using fine-
grained Twitter data and a Google search volume index to predict style-color sales
three months out reduces forecast error by 11% compared to conventional
methods.
4 - Wisdom of Crowds: Forecasting using Prediction Markets
Ruomeng Cui, Assistant Professor, Indiana University, 309 E.
Tenth Street, Bloomington, IN, 47401, United States of America,
cuir@indiana.edu,Achal Bassamboo, Antonio Moreno-Garcia
Prediction markets are virtual markets created to aggregate predictions from the
crowd. We examine data from a public prediction market and internal prediction
markets run at three corporations. We study the efficiency of these markets in
extracting information from participants. We show that the distribution forecasts,
such as sales and commodity prices predictions, generated by the crowds are
perfectly calibrated. In addition, we run a field experiment to study drivers of
forecast accuracy.
MC51
51-Room 106B, CC
Dynamic Contracts in Operations Management
Sponsor: Manufacturing & Service Operations Management
Sponsored Session
Chair: Hao Zhang, Associate Professor, University of British Columbia,
Sauder School of Business, Vancouver, BC, V6T1Z2, Canada,
hao.zhang@sauder.ubc.ca1 - Optimal Long-term Supply Contracts with Asymmetric
Demand Information
Wenqiang Xiao, Associate Professor, New York University, Stern
School of Business, 44 West Fourth Street, 8-72, New York, NY,
10012, United States of America,
wxiao@stern.nyu.edu,
Ilan Lobel
We consider a manufacturer selling to a retailer with private demand information
arising dynamically over an infinite time horizon. We show that the
manufacturer’s optimal dynamic long-term contract takes a simple form: in the
first period, based on her private demand forecast, the retailer selects a wholesale
price and pays an associated upfront fee, and, from then on, the two parties stick
to a simple wholesale price contract with the retailer’s chosen price.
2 - Dynamic Mechanisms for Online Advertising
Hamid Nazerzadeh, University of Southern California, Bridge
Memorial Hall, 3670 Trousdale Parkway, Los Angeles, CA, 90089,
United States of America,
hamidnz@marshall.usc.edu,
Vahab Mirrokni
I will discuss designing dynamic contracts for selling display advertising. I will
show that under natural but rather restricted assumptions, the traditional
reservation contracts can be revenue-optimal. I will also present the optimal
mechanism in a general setting and discuss their practical implementations.
3 - Dynamic Short-term Contracts under Private Inventory
Information and Backlogging
Lifei Sheng, PhD Candidate, University of British Columbia, 2053
Main Mall, Vancouver, BC, V6T1Z2, Canada,
Fay.Sheng@sauder.ubc.ca,Mahesh Nagarajan, Hao Zhang
We study a setting where a supplier sells to a retailer facing random demand over
multiple periods. At the beginning of each period, the supplier offers a one-period
contract and the retailer decides his order quantity before the demand realizes.
The retailer carries leftover inventory or backlogs unmet demand, which is
unobservable by the supplier. We show interesting properties of the supplier’s
optimal contract and study special cases when the problem is tractable.
4 - Structures of Optimal Dynamic Mechanisms
Alexandre Belloni, Professor Of Decision Sciences, Duke
University, 100 Fuqua Drive, Duke University, Durham, NC,
27708, United States of America,
abn5@duke.edu,Peng Sun,
Bingyao Chen
Consider a principal procures up to one unit of a product/service in every period
from an agent who is privately informed about its marginal production cost in
each period. We identify regularity conditions on the distribution of private
information under which the optimal contracts offer at most two different
procurement levels depending on the newly reported cost. Our results rely on
“dynamic virtual valuation,” a generalization of the Myersonian virtual valuation
in the static setting.
MC52
52-Room 107A, CC
Analytics for IT Services
Sponsor: Service Science
Sponsored Session
Chair: Aly Megahed, Research Staff Member, IBM Research, 650 Harry
Road - Office D3-428, San Jose, CA, 95120, United States of America,
aly.megahed@us.ibm.com1 - Operations Research and Analytics Solutions for it
Service Providers
Aly Megahed, Research Staff Member, IBM Research,
650 Harry Road - Office D3-428, San Jose, CA, 95120,
United States of America,
aly.megahed@us.ibm.com,
Hamid Reza Motahari Nezhad, Peifeng Yin, Taiga Nakamura
Large IT service providers compete to win highly-valued outsourcing IT deals via
submitting proposals to potential clients. In this talk, you will learn about some of
the analytics and OR work done for managing such complex service
engagements. A case management approach that analyzes costs and prices of
deals in preparation will be presented. Additionally, a predictive analytics tool for
identifying the influential factors on the outcome of deals will be shared.
2 - Measuring Cloud Services Profitability
Ray Strong, Impact Of Future Technology, IBM Research,
650 Harry Road, San Jose, CA, 95120, United States of America,
hrstrong@us.ibm.com,Jeanette Blomberg, Sunhwan Lee, Anca
Chandra, Pawan Chowdhary, Susanne Glissmann, Robert Moore
The costs of providing cloud services are not easily attributable to revenue. We
present a complex modeling approach to understanding the profitability of
individual service offerings and individual service contracts. We explore ways of
creating long running models of cloud service performance in spite of the month-
to-month and pay-for-use nature of many cloud contracts. We suggest an
approach to estimating the total current value of a cloud service contract to a
vendor.
MC50