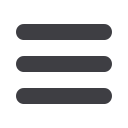

INFORMS Philadelphia – 2015
221
2 - Value of Dynamic Pricing in Congestible Systems
Jeunghyun Kim, University of Southern California, Marshall
School of Business, Bridge Hall 401, Los Angeles, CA, 90089,
United States of America,
jeunghyun.kim.2015@marshall.usc.edu,Ramandeep Randhawa
From UBER to express lanes on highways, dynamically changing the premium for
access to limited resources based on congestion is prevalent. Our research
question is: what is the value of dynamic pricing over static pricing in such
systems. By modeling a firm that caters to price- and delay-sensitive customers,
we analytically prove that the value can be significant and a simple dynamic
scheme of using only two price points reaps most of this value.
3 - Price Competition with Customer Search in Congested
Environments
Laurens Debo,Associate Professor, Dartmouth College,
100 Tuck Hall, Hanover NH 03755, United States of America,
laurens.g.debo@tuck.dartmouth.edu,Varun Gupta, Luiyi Yang
We study how firms compete in service rate when congestion-sensitive customer
search, at some cost, for the firm with the shortest line. We find that decreasing
search costs increases search and intensifies service rate competition, which
reduces firms’ equilibrium profits. Firms can get around by inflicting random
costs on customers.
4 - Learning and Earning for Congestion-prone Service Systems
Philipp Afeche, Associate Professor, University of Toronto,
105 St. George Street, Toronto, ON, M5S3E6, Canada,
afeche@rotman.utoronto.ca, Bora Keskin
We consider a firm that sells a service in a congestion-prone system to price- and
delay-sensitive customers. The firm faces Bayesian uncertainty about the
consumer demand for its service and can dynamically make noisy observations on
the demand. We characterize the structure and performance of the myopic
Bayesian policy and well-performing variants. Our results show that capacity
constraints have an important effect on performance.
MC47
47-Room 104B, CC
Energy Operations and Energy Efficiency
Sponsor: Manufacturing & Service Oper Mgmt/Sustainable
Operations
Sponsored Session
Chair: Nur Sunar, Assistant Professor, University of North Carolina,
Kenan-Flagler School of Business, Chapel Hill, NC,
United States of America,
Nur_Sunar@kenan-flagler.unc.edu1 - A Unifying Framework for Consumer Surplus under
Stochastic Demand
Georgia Perakis, MIT, 77 Massachusetts Avenue, Cambridge, MA,
02139, United States of America,
georgiap@mit.edu,
Maxime Cohen, Charles Thraves
We present a general extension of the consumer surplus for stochastic demand
under several capacity rationing rules. We derive this extension from a graphical
approach as well as from a utility maximization perspective. We then use this
definition to study the impact of demand uncertainty on consumers in interesting
applications including the electric vehicle market. We show that in many cases
demand uncertainty may actually hurt consumers.
2 - Optimal Utilization of Energy Storage for Energy Shifting
Gilvan (Gil) Souza, Professor, Indiana University, Kelley School of
Business, Bloomington, IN, 47405, United States of America,
gsouza@indiana.edu, Shanshan Hu, Shanshan Guo
Batteries may be used for energy shifting in the power system: storing electricity
when the power supply is abundant and cheap, and releasing electricity when the
supply is tight and more expensive. Both permanent capacity loss and useful life
of a battery are affected by discharge decisions in energy shifting. This paper
studies the optimal discharge decisions that maximize the total profit of energy
shifting in a battery’s entire life.
3 - Do Profitable Carbon Emission Reduction Opportunities Decrease
Over Time? A Perspective Based on CDP
Christian Blanco, University of California-Los Angeles,
Los Angeles, CA, United States of America
christian.noel.blanco@gmail.com,Felipe Caro, Charles Corbett
Using climate change-related surveys collected by CDP (formerly the Carbon
Disclosure Project), we investigate if firms experience decreasing opportunities for
profitable initiatives to reduce greenhouse gas emissions. We also characterize
payback and marginal abatement costs of these energy efficiency investments
over time.
4 - Strategic Commitment to a Production Schedule with Supply and
Demand Uncertainty: The Renewable Power
Nur Sunar, Assistant Professor, University of North Carolina,
Kenan-Flagler School of Business, Chapel Hill, United States of
America,
Nur_Sunar@kenan-flagler.unc.edu,John Birge
How should a renewable power producer strategically commit to a production
schedule in a day-ahead electricity market? How does this commitment affect the
day-ahead price? Motivated by these important questions, we introduce and
analyze via the ODE theory a supply function competition model with demand
and supply uncertainty. Using our novel equilibrium characterization, we study
the implications of different penalty schemes and subsidy for equilibrium
production schedules and market outcomes.
MC49
49-Room 105B, CC
Emerging Topics in Supply Chain Management
Sponsor: Manufacturing & Service Oper Mgmt/Supply Chain
Sponsored Session
Chair: Hakjin Chung, Stephen M. Ross School of Business, University
of Michigan, Ann Arbor, MI, United States of America,
hakjin@umich.eduCo-Chair: Kun Soo Park, Assistant Professor, KAIST College of
Business, 85 Hoegi-ro, Dongdaemun-gu, Seoul, 130722, Korea,
Republic of,
kunsoo@kaist.ac.kr1 - The Newsvendor under Demand Ambiguity: Combining Data with
Moment and Tail Information
Soroush Saghafian, Harvard University, 79 JFK Street,
Cambridge, MA, 02138, United States of America,
Soroush.Saghafian@asu.edu, Brian Tomlin
Data-driven approaches typically assume that the planner has no information
beyond the evolving history of demand observations. The planner may, however,
have partial information about the demand distribution in addition to demand
observations. We propose a non-parametric, maximum-entropy based technique,
termed SOBME (Second Order Belief Maximum Entropy), which allows the
planner to effectively combine demand observations with partial distributional
information.
2 - Managing The Supply-demand Mismatch with Complementary
Product Flow Options
Alexander Angelus, University of Texas,
Jindal School of Management, Dallas, TX, United States of
America,
alexandar.angelus@utdallas.edu,Ozalp Ozer
To address the pervasive supply-demand mismatch in multi-stage supply chains
with stochastic demand, we use the option to expedite shipments downstream to
manage excess demand, and allow for returns of stock upstream to deal with
excess inventory. We identify the optimal policy that decomposes this multi-
dimensional problem into single-dimensional subproblems. Our numerical studies
of supply chains with both expediting and returns of stock find those two product
flow options to be complementary.
3 - Capacity Investment with Demand Learning
Anyan Qi, Assistant Professor, University of Texas at Dallas, 800
W Campbell Rd, Richardson, TX, 75080, United States of
America,
axq140430@utdallas.edu, Amitabh Sinha
We study a firm’s strategy to adjust its capacity using information learned from
observed demand. We characterize the firm’s optimal policy and develop an
easily-implementable and data-driven heuristic about when and by how much
the firm should adjust its capacity. We also numerically validate the performance
of our heuristic.
4 - Sequential Capacity Allocation under Order Manipulation:
Efficiency and Fairness
Kun Soo Park, Assistant Professor, KAIST(Korea Advanced
Institute of Science and Technology), 410 Supex Bldg,
85 Hoegiro, Dongdaemun-g, Seoul, Korea, Republic of,
kunsoo@business.kaist.ac.kr, Seyed Iravani, Bosung Kim
We analyze the strategic behaviors of the supplier and manufactures in sequential
capacity allocations when the manufacturers’ order strategy is not necessarily
truthful to the supplier. We show how an allocation changes under order
manipulation and consider two directions to improve sequential allocation
mechanisms under order manipulation from the perspective of efficiency and
fairness of an allocation.
MC49