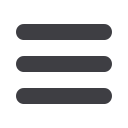

INFORMS Philadelphia – 2015
311
4 - Analysis of Best Practices for Energy Efficient Buildings through
Building Energy Modeling in Design
Chung-suk Cho, Assistant Professor, Khalifa University, Al Saada
St. and Muroor Rd., Abu Dhabi, 127788, United Arab Emirates,
chung.cho@kustar.ac.ae, Young-ji Byon
Building energy performance modeling evaluates energy efficient design options.
There are significant amount of misused opportunities of energy efficiency-related
decisions that could be incorporated into the building design through quality
building energy performance modeling. The best practice analysis will help
optimize the building design and will allow the design team to prioritize
investment in the strategies that will have the greatest effect on the building’s
energy use.
5 - Two-stage Optimal Demand Response with Battery Energy
Storage Systems
Yanyi He, Senior Scientist, IBM, 1001 E Hillsdale Blvd,
Foster City, CA, 94404, United States of America,
heyanyidaodao@gmail.com, Zhaoyu Wang
Proposes a two-stage co-optimization framework for the planning and energy
management of a customer with battery energy storage systems (BESSs) and
demand response (DR) programs. The first stage is to assist a customer to select
multiple DR programs to participate and install batteries to coordinate with the
demand side management. The second stage is to perform energy management
according the planning decisions, including dispatches of batteries, loads, and
DGs.
TB79
79-Room 302, CC
Software Demonstration
Cluster: Software Demonstrations
Invited Session
1 -
Statistics.com- A Survey of Data Analytics Methods
Peter Bruce, Founder and President, The Institute for Statistics
Education at
Statistics.comThis workshop will survey the field of data analytics, reviewing both traditional
statistical methods and machine learning methods, including predictive modeling,
unsupervised learning, text mining, statistical inference, time series forecasting,
recommender systems, network analytics, and more. It will be a broad brush
treatment aimed at newcomers, as well as those with knowledge in one area who
wish to understand where other analytic methods fit into the picture.
2 - River Logic - Code-free modeling for large-scale LP and MIP
problems using Enterprise Optimizer
Eric Kelso, VP Product Management, River Logic
Enterprise Optimizer is a code-free, visual LP and MIP optimization modeling
platform. Using EO’s intuitive drag-and-drop interface, learn how to rapidly
create integrated process and financial models. Also learn about EO’s wizard-
driven data integration, query designer, user-defined schema, dashboard builder,
VBA integration, APIs and job automation component. Outputs demonstrated
include detailed unit costs and audit-quality P&L, Balance Sheet and Cash Flow
statements. The entire session will be spent discussing major features and
showing real-world applications.
Tuesday, 12:30pm - 2:30pm
Exhibit Hall A
Tuesday Poster Session
Contributed Session
Chair: Min Wang, Drexel University, 3141 Chestnut Street,
Philadelphia, PA, United States of America,
mw638@drexel.eduCo-Chair: Allen Holder, Rose-Hulman Mathematics, Terre Haute, IN,
United States of America,
holder@rose-hulman.eduCo-Chair: Wenjing Shen, Drexel University, Philadelphia, PA,
United States of America,
ws84@drexel.edu1 - Surgery Scheduling with Recovery Resources
Maya Bam, University of Michigan, Industrial and Operations
Engineering, 1205 Beal Ave., Ann Arbor, MI, 48109,
United States of America,
mbam@umich.edu, Mark Van Oyen,
Mark Cowen, Brian Denton
Surgery scheduling is complicated by the post-anesthesia care unit, the typical
recovery resource. Based on collaboration with a hospital, we present a novel, fast
2-phase heuristic that considers both surgery and recovery resources. We show
that each phase of the heuristic has a tight provable worst-case performance
bound. Moreover, the heuristic performs well compared to optimization based
methods when evaluated under uncertainty using a discrete event simulation
model.
2 - Inventory Control with Unknown Demand and
Nonperishable Product
Tingting Zhou, Rutgers University, 1 Washington Park, Newark,
NJ, 07102, United States of America,
tingzhou@rutgers.edu,Michael Katehakis, Jian Yang
We study an inventory control problem with unknown discrete demand
distribution, focusing on the analysis of an adaptive algorithm based on empirical
distributions and the newsvendor formula. When items are nonperishable, the
algorithm can achieve a near square-root-of-T bound on its regret over the ideal
case where demand distribution were known.
3 - Optimizing Information System Security Investments with Risk:
Insights for Resource Allocation
Yueran Zhuo, PhD Candidate, University of Massachusetts
Amherst, Isenberg School of Management, Amherst, MA, 01003,
United States of America,
yzhuo@som.umass.edu, Senay Solak
Information security has become an integral component of a firm’s business
success, and thus investing on information security countermeasures is an
important decision problem for many businesses. We use a portfolio approach to
study the optimal investment decisions of a firm, where the uncertainty of
information security environment is captured through a stochastic programming
framework. Results cast managerial insights for information security investment
planning by a firm.
4 - Adaptive Decision-Making of Breast Cancer Mammography
Screening: A Heuristic-Based Regression Model
Fan Wang, University of Arkansas,, Fayettevlle, AR,
United States of America,
fxw005@uark.edu,Shengfan Zhang
The American Cancer Society currently recommends all U.S. women undergo
routine mammography screenings beginning at age 40. However, due to the
potential harms associated with screening mammography, such as overdiagnosis
and unnecessary work-ups, the best strategy to design an appropriate breast
cancer mammography screening schedule remains controversial. This study
presents a mammography screening decision model that aims to identify an
adaptive screening strategy while considering disadvantages of mammography.
We present a two-stage decision framework: (1) age- specific breast cancer risk
estimation, and (2) annual mammography screening decision-making based on
the estimated risk. The results suggest that the optimal combinations of
independent variables used in risk estimation are not the same across age groups.
Our optimal decisions outperform the existing mammography screening
guidelines in terms of the average loss of life expectancy. While most earlier
studies improved the breast cancer screening decisions by offering lifetime
screening schedules, our proposed model provides an adaptive screening decision
aid by age. Since whether a woman should receive a mammogram is determined
based on her breast cancer risk at her current age, our “on-line” screening policy
is adaptive to a woman’s latest health status, which causes less bias in reflecting
the individual risk of every woman.
5 - Optimization of Netting Scheme in Large-scale Payment Network
Shuzhen Chen, University of Science & Technology of China,
No. 98, Jinzhai Road, Hefei, China,
csz@mail.ustc.edu.cnAs netting becomes combined with real-time settlement, an efficient netting
method is required to deal with the large-scale payment network. Network
optimization may not be optimal due to repeated searching of shortest path. A
new method is proposed to optimize the netting process by assembling payments
in two specific routes. It can minimize the amount of total payments for the
whole network and ensure unchanged net payment for each bank. Moreover, it
has polynomial time-complexity.
6 - Wasserstein Metric and the Distributionally Robust TSP
Mehdi Behroozi, University of Minnesota, Minneapolis, MN,
United States of America,
behro040@umn.edu,John Gunnar Carlsson
Recent research on the robust and stochastic travelling salesman problem and the
vehicle routing problem has seen many di?erent approaches for describing the
region of uncertainty, such as taking convex combinations of observed demand
vectors or imposing constraints on the moments of the spatial demand
distribution. One approach that has been used outside the transportation sector is
the use of statistical metrics that describe a distance function between two
probability distributions. In this paper, we consider a distributionally robust
version of the Euclidean travelling salesman problem in which we compute the
worst-case spatial distribution of demand against all distributions whose earth
mover’s distance to an observed demand distribution is bounded from above. This
constraint allows us to circumvent common overestimation that arises when
other procedures are used, such as fixing the center of mass and the covariance
matrix of the distribution.
POSTER SESSION