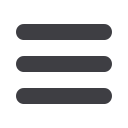

INFORMS Philadelphia – 2015
352
5 - Credit Scoring using Dynamic State Space Model under
Statistical Volatility
Linna Du, Data Scientist, CACS, 2259 Adam Clayton Powell,
New York, NY, 10027, United States of America,
linna.du@gmail.comIn emerging market where the credit score and credit history are not trustworthy,
the estimation and prediction of the credit score and prepayment risks are very
important. In the paper, we propose a dynamic state space model considering the
volatility and dynamic feature of the lending market. We found that the time
varying volatility model provides better prediction than other time series models.
We also identify the key factors that drive the lending risks.
TD21
21-Franklin 11, Marriott
Disease Modeling in OR
Sponsor: Health Applications
Sponsored Session
Chair: Emine Yaylali, Senior Service Fellow, Centers for Disease Control
and Prevention, 1600 Clifton Road, Atlanta, GA, 30333,
United States of America,
wqq3@cdc.gov1 - The Potential Impact of Reducing Indoor Tanning on Melanoma
Prevention in the United States
Yuanhui Zhang, CDC, Chamblee GA 30341,
United States of America,,
yfp5@cdc.gov,Donatus Ekwueme,
Sun Hee Rim, Meg Watson, Gery Guy
More than 700,000 adults in the United States are treated for melanoma each
year, resulting in annual direct medical costs of $3.3 billion dollars and 9,000
deaths. We developed a Markov model to estimate the health and economic
impacts of reducing indoor tanning for melanoma prevention in the United States
under certain assumptions. According to this model, reducing indoor tanning may
result in favorable savings in medical costs and life-years, comparable to other
national prevention efforts.
2 - Estimating the Impact of HIV Care Continuum Interventions on the
Reproduction Number
Yao-Hsuan Chen, CDC, Chamblee GA 30341,
United States of America
,xhj1@cdc.gov,Andrew Hill,
Paul G. Farnham, Stephanie L. Sansom
We used a compartmental model to study HIV transmission in the United States
from 2006 through 2020 among heterosexuals, men who have sex with men,
including bisexual men, and injection drug users. We analyzed the impact of
interventions to improve HIV diagnosis, care, and treatment on the reproduction
number. Analyses using this model can provide insights into the long-term
effectiveness of HIV prevention strategies.
3 - Stratifying Risk Groups in Compartmental Epidemic Models:
Where to Draw the Line?
Margaret L. Brandeau, Professor, Stanford University, MS&E
Department, Stanford, CA, 94305, United States of America,
brandeau@stanford.edu,Jeremy D. Goldhaber-fiebert
Disease models used to support cost-effectiveness analyses of health interventions
are often stratified to reflect population heterogeneity (e.g., age, gender, risk
behaviors). We examine the impact of population stratification in dynamic disease
transmission models: specifically, the impact of different divisions of a population
into a low-risk and a high-risk group. We show that the way in which the
population is stratified can significantly affect cost-effectiveness estimates.
4 - Developing a Dynamic Compartmental Model of HIV in the
United States
Emine Yaylali, Senior Service Fellow, Centers for Disease Control
and Prevention, 1600 Clifton Road, Atlanta, GA, 30333,
United States of America,
wqq3@cdc.gov, Paul G. Farnham,
Stephanie L. Sansom, Katherine A. Hicks, Emily L. Tucker,
Amanda Honeycutt
Over 1 million people in the US are living with HIV. To observe trends in HIV and
evaluate the effectiveness of prevention interventions, we developed a dynamic
compartmental model of disease progression and transmission. The population
was stratified by age, sex, circumcision status, race/ethnicity, transmission group,
and risk level. People progressed between compartments defined by disease status
and care and treatment stage. Outcomes included HIV incidence, prevalence, and
care status.
TD22
22-Franklin 12, Marriott
Contact Centers
Sponsor: Applied Probability
Sponsored Session
Chair: Rouba Ibrahim, University College London, London, N7 8EP,
United Kingdom,
rouba.ibrahim@ucl.ac.uk1 - Telephone Call Centers: Asymptotic Optimality of Myopic
Forecasting-scheduling Scheme
Han Ye, University of Illinois at Urbana Champaign, 350 Wohlers
Hall, 1206 South Sixth Street, Champaign, IL, 61820, United
States of America,
hanye@illinois.edu, Noah Gans, Haipeng Shen,
Yong-Pin Zhou
We determine workforce schedules for call center arrivals that are doubly
stochastic. Period-by-period arrival rates follow a hidden AR(1) process, and only
arrival counts are observed. We formulate stochastic programs to minimize long-
run average staffing costs, subject to a long-run average constraint on
abandonment. We show that, in steady state, repeated, myopic solution of the
single-period problem is stable, has low cost, and meets the abandonment
constraint.
2 - A Structural Model for Agents’ Strategic Behavior in Call Centers
Dongyuan Zhan, University of Southern California,
Los Angeles, CA, United States of America,
Dongyuan.Zhan.2015@marshall.usc.edu, Amy Ward,
Seyed Emadi
We do an empirical study of agent behavior in call centers. We begin by observing
that regression analyses have low explanatory power, even though the data
shows that agents speed up or slow down depending on the system load and their
fatigue level. This leads us to investigate utility based structural models for agent
behavior.
3 - Capacity Sizing with a Random Number of Agents
Rouba Ibrahim, University College London, London, N7 8EP,
United Kingdom,
rouba.ibrahim@ucl.ac.ukWe study the problem of staffing many-server queues with general abandonment
and a random number of servers. For example, uncertainty in the number of
servers may arise in virtual call centers where agents are free to set their own
schedules. We rely on a fluid model to determine optimal staffing levels, and
demonstrate the asymptotic accuracy of the fluid prescription. We also
characterize the optimal staffing policy with self-scheduling agents.
TD23
23-Franklin 13, Marriott
Markov Decision Models and Approximations for
Manufacturing
Cluster: Stochastic Models: Theory and Applications
Invited Session
Chair: Tugce Martagan, Eindhoven University of Technology, 5600 MB
Eindhoven, Eindhoven, Netherlands,
T.G.Martagan@tue.nl1 - Robust Approximate Dynamic Programming and Structured
Policies for Degradable Energy Storage
Marek Petrik, IBM, 1101 Kitchawan Rd., Yorktown Heights, NY,
10598, United States of America,
mpetrik@us.ibm.comBatteries hold great promise for energy storage in arbitrage in electric grids but
can degrade rapidly with use. In this talk, we analyze the impact of storage
degradation on the structure of optimal policies and describe robust approximate
dynamic programming methods that take advantage of the policy structure.
2 - Component Reservation for Asymptotically Optimal Allocation in
Assemble to Order Production Systems
Haohua Wan, University of Illinois at Urbana-Champaign,
104 South Mathews Ave., Urbana, IL, United States of America,
hwan3@illinois.edu,Qiong Wang
Component reservation is not myopically optimal as it sometimes holds back
components from existing demands. We prove that in many cases, without
reservation, component allocation cannot be asymptotically optimal, i.e., the
percentage difference of the discounted inventory cost from its lower bound does
not converge to zero as demand and production volumes increase, even though
such convergence is achievable under other policies that reserve components for
high-value product demands.
TD21