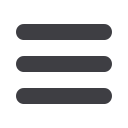

INFORMS Philadelphia – 2015
356
4 - Modeling Time-varying Autocorrelation for Time
Series Classification
Mustafa Gokce Baydogan, Assistant Professor, Bogaziçi
University, Department of Industrial Engineering, Bebek,
Istanbul, 34342, Turkey,
mustafa.baydogan@boun.edu.tr,George Runger
We introduce a novel approach to model the dependency structure in time series
(TS) that generalizes the concept of autoregression to local auto-patterns. A
learning strategy that is fast and insensitive to parameter settings is the basis for
the approach. This unsupervised approach to represent TS generally applies to a
number of data mining tasks. We provide a research direction that breaks from
the linear dependency models to potentially foster other promising nonlinear
approaches.
TD32
32-Room 409, Marriott
Data Mining
Contributed Session
Chair: Gustavo Lujan-Moreno, Arizona State University, Tempe, AZ,
United States of America,
glujanmo@asu.edu1 - Open-source Statistical Packages: The True Cost of
“Free” Software
Ronald Klimberg, Saint Joseph’s University, 35 Moorlinch Blvd.,
Medford, NJ, 08055, United States of America,
klimberg@sju.edu,
Rick Pollack, Susan Foltz Boklage
Open source software is typically free and widely accessible to the public. In the
statistical realm, R is the dominant open-source player. Is it really free? Where do
you go for support? Are their possible significant costs associated with using R?
Further, to what degree should open-source statistical software be used and
taught in academia? This article will explore these questions, as well as others, in
discussing what are often the hidden costs of using open-source statistical
software
2 - Study on Effects on Emotional Intensities of Negative Online
Reviews on its Usefulness
Cuiping Li, Huazhong University of Science and Technology, 1037
Luoyu Rd, Hongshan District,Wuhan, Hubei, 430074, China,
412543536@qq.com, Qian Yuan, Shuqin Cai
Aimed at recognizing high quality reviews from mass data, this paper explores
how reviews’ negative emotions influence the usefulness of negative online
reviews by using data mining technology and regression analysis. The result
reveals that strong negative emotions reduce negative reviews’ usefulness and
moderate negative emotions have opposite effect. Results also show that different
intensities of negative emotions have significant interactions on reviews’
usefulness.
3 - Impact of Library Online Resource use on Students
Academic Outcome
Fan Zhang, University of Pittsburgh, 1048 Benedum Hall,
Department of Industrial Engineering, Pittsburgh, PA, 15261,
United States of America,
faz31@pitt.edu,Louis Luangkesorn,
Ziyi Kang, Yunjie Zhang, Shi Tang
University libraries have a need to demonstrate the impact of their resources on
the University mission: academics and research. However, for electronic
resources, research has shown that students often do not recognize they are using
library resources, making surveys and other assessments not useful. We use
undergraduate demographic and academic outcome data along with logs of online
library resource access to determine if relationship exists between online resource
use and academic outcome.
4 - A Case-Crossover Study to Evaluate the Effect of Player Affective
State on Performance in Video Game
Gustavo Lujan-Moreno, Arizona State University, Tempe, AZ,
United States of America,
glujanmo@asu.eduUsing an electroencephalogram (EEG) headset we examined whether there was a
significant change in the affective state reported by the EEG when a participant
made a mistake while playing a popular video game. There were five affective
constructs that were examined: engagement, frustration, meditation, short and
long term excitement. We propose a case-crossover methodology to analyze this
type of events. Results show that there is a significant difference in three affective
states.
5 - Enterprise Social Networking and Firm Creativity
Donghyun Kim, Delta State University, 1003 West Sunflower
Road, Cleveland, MS, 38733, United States of America,
dkim@deltastate.edu,Jaemin Kim
This study examines the influence of firm’s IT social networking (SN) capacity on
the firm’s creativity and innovation. Analyzing data on utility patents of 7 firms
using enterprise SN, we tested our predictions on a balanced panel of the firms’
data. The results illustrate how IT SN capacity can aid in the generation of an
idea.
TD33
33-Room 410, Marriott
Decision and Prediction Models in Healthcare
Sponsor: Health Applications
Sponsored Session
Chair: Jakob Kotas, University of Washington, Dept. of Applied
Mathematics, Box 353925, Seattle, WA, 98195,
United States of America,
jkotas@uw.edu1 - A Stochastic Program with Chance-constrained Recourse for
Surgery Scheduling and Rescheduling
Gabriel Zenarosa, PhD Candidate, University of Pittsburgh, 3700
O’Hara Street, Benedum Hall 1048, Pittsburgh, PA,
15261-3048, United States of America,
glz5@pitt.edu,Andrew J. Schaefer, Oleg Prokopyev
Aggregate surgical expenditures in the US amount to a significant percentage of
GDP. About 42% of hospital revenues are generated by operating rooms (ORs),
yet ORs run at only 68% capacity on average. The most important issues in OR
management are centered on scheduling. Advance schedules improve OR
efficiency; however, surgeries are rescheduled in practice as they rarely go as
planned. We present a stochastic program with chance-constrained recourse for
surgery scheduling and rescheduling.
2 - Dynamic Scheduling of a Post-discharge Follow-up Organization
to Reduce Readmissions
Sean Yu, Indiana University-Bloomington, 1275 E. Tenth Street,
Bloomington, IN, 47405, United States of America,
xy9@indiana.edu, Shanshan Hu, Jonathan Helm
Hospital readmissions are a growing problem. Many readmissions are preventable
by properly monitoring patients post-discharge. We consider an organization that
dynamically will schedule and staff post-discharge monitoring schedules for a
cohort of patients being randomly discharged from client hospitals. We formulate
this problem as an infinite horizon dynamic program that can be solved using
approximate dynamic programming.
3 - Predictive Capabilities in Hierarchical Node-based Clustering of
Time Series
Hootan Kamran, PhD Candidate, University of Toronto,
12 Rodney Blvd., North York, ON, M2N4B6, Canada,
hootan@mie.utoronto.ca, Dionne Aleman, Kieran Moore,
Mike Carter
Flu activity is shown to be affected by local variables such as climate. Therefore,
localized activity must be monitored to study spatiotemporal spread patterns of
the disease. Using a 10-year flu dataset from 103 hospitals in Ontario, we
compare predictive capabilities extracted from existing aggregation scheme
(LHIN) with those extracted from the novel hierarchical node-based clustering
scheme and show that the new method will extract more statistically significant
predictive capabilities.
4 - A Stochastic Dynamic Programming Model for
Response-guided Dosing
Jakob Kotas, University of Washington, Dept. of Applied
Mathematics, Box 353925, Seattle, WA, 98195,
United States of America,
jkotas@uw.edu,Archis Ghate
We discuss a stochastic dynamic programming (DP) model to assist with dosing
decisions in response-guided dosing (RGD). The goal in this framework is to
deliver the right dose to the right patient at the right time. We present robust,
optimal learning, and optimal stopping variants of this problem. The structure of
optimal policies in these problems will be explored both analytically and
numerically.
TD32