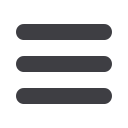

INFORMS Philadelphia – 2015
404
WB12
12-Franklin 2, Marriott
Optimization Stochastic II
Contributed Session
Chair: Ruediger Schultz, Prof., University of Duisburg-Essen, Faculty of
Mathematics, Thea-Leymann-Str. 9, Essen, D-45127, Germany,
ruediger.schultz@uni-due.de1 - Sampling-based Approximation Schemes for Capacitated
Stochastic Inventory Control Models
Wang Chi Cheung, Graduate Student, Massachusetts Institute of
Technology, 77 Massachusetts Ave, Cambridge, MA, 02139,
United States of America,
wangchimit@gmail.com,David Simchi-levi
We study the multi-period capacitated stochastic inventory control problem in a
data-driven setting, where the demand distributions can only be accessed through
samples. We apply the Sample Average Approximation (SAA) method, and
establish a polynomial upper bound on the number of samples needed for
achieving near-optimality. However, the underlying SAA problem is #P-hard.
Thus we provide a polynomial time approximation scheme, which involves a
subgradient sparsification procedure.
2 - Competitive Capacity Investment under Uncertainty
Xishu Li, PhD Candidate, Erasmus University, Dept.
Technology&Operations Management, Burgemeester Oudlaan 50,
Rotterdam, Ro, 3062 PA, Netherlands,
x.li@rsm.nl,Rob Zuidwijk,
Rommert Dekker, Rene De Koster
Our research explores a fleet capacity investment problem under market
uncertainty. We study how competition between firms affects investment
strategies, and investigate the optimal investment policy. Here, we focus on a
single vessel type with the intention to extend our results to also incorporate
green vessels.
3 - ADMM for Two-Stage Stochastic Programs with Quadratic
Objective Function
Sebastian Arpon, Universidad Adolfo Ibañez, Diagonal Las Torres
2640, Peñalolen, Santiago, Chile,
sebarpon@gmail.com,
Tito Homem-de-mello, Bernardo Pagnoncelli
We discuss a decomposition method for two-stage stochastic programs with
quadratic objective functions. Our algorithm is based on the Alternating Direction
Method of Multipliers (ADMM) developed in the literature, and decomposes the
problem by scenarios. Some attractive features of the algorithm are the low
computational cost per iteration and its suitability for parallelization. We discuss
some aspects related to convergence of the method and present numerical results
to illustrate the ideas.
4 - The Dynamic Multi-newsvendor Problem
Zhaohu Fan, PhD Student, The Pennsylvania State University,
244 Leonhard Building, State College, PA, 1680001, United States
of America,
zxf109@psu.edu, Terry Friesz, Yiou Wang, Tao Yao
We articulate a dynamic model of newsvendors where a set of service providers
form an oligopoly that is equilibrium tending.The price setting mechanism
involving the providers resembles the replicator dynamics of evolutionary game
theory. We show that generalization of the news vendor problem to a Cournot-
Nash differential game based on replicator dynamics in a stochastic setting takes
the form of a stochastic differential variational inequality.
5 - Stochastic Programming in Gas Transportation using
Symbolic Computation
Ruediger Schultz, Prof., University of Duisburg-Essen, Faculty of
Mathematics, Thea-Leymann-Str. 9, Essen, D-45127, Germany,
ruediger.schultz@uni-due.deNomination validation, i.e., to decide technical feasibility of a transportation order
with balanced in- and output, is among the challenges in daily operation of gas
networks. We address the problem in the steady-state case with uncertain orders.
In particular we provide parametric solution procedures for polynomial equations
resulting from Kirchhoff’s Laws based on insights and procedures from
computational algebra.
WB13
13-Franklin 3, Marriott
Robust Optimization: Theory and Applications
Sponsor: Optimization/Optimization Under Uncertainty
Sponsored Session
Chair: Chaitanya Bandi, Kellogg School of Management,
Northwestern University, Evanston, United States of America,
c-bandi@kellogg.northwestern.edu1 - On the Adaptivity Gap in Two-stage Robust Linear Optimization
under Constraints
Vineet Goyal, Columbia University IEOR department,
500 West 120th Street, 304 Mudd, New York, NY, 10027,
United States of America,
vg2277@columbia.edu,Brian Lu
We consider two-stage adjustable robust linear optimization problem with
uncertain constraint coefficients that models many important applications
including resource allocation with uncertain requirements. The adjustable
problem is hard to approximate within a factor better than O(log n) in general.
We show that the static solution gives a O(log^2 n)-approximation for the
adjustable robust problem. Surprisingly, this is nearly the best possible
approximation for the problem.
2 - A Robust Optimization Approach to Optimizing
Expected Performance
Nataly Youssef, MIT, 20 Palermo Street, Cambridge, MA,
United States of America,
youssefn@mit.eduWe propose a tractable approach for optimizing the expected performance of
stochastic systems via robust optimization. We model uncertainty via
parameterized polyhedral sets inspired by probabilistic limit laws and
characterized by variability parameters. We then cast the performance
optimization problem as a robust optimization problem. We demonstrate the
tractability and accuracy of our approach via an inventory management example.
3 - Resource Allocation under Coherent Distortion Risk Measures
Chaitanya Bandi, Kellogg School of Management,
Northwestern University, Evanston, IL, United States of America,
c-bandi@kellogg.northwestern.edu,Paat Rusmevichientong
We consider high dimensional resource allocation problems faced by a decision
maker with a sophisticated risk attitude modeled by a fairly general risk measure
known as a coherent distorted risk measure (CDRM) which encompasses many
popular risk measures such as spectral risk measures and law-invariant coherent
risk measures. We address the problem of tractability and obtain explicit closed
form solution for the this problem while identifying new properties of the optimal
solution.
WB14
14-Franklin 4, Marriott
Risk-Averse Control of Markov Systems
Sponsor: Optimization/Optimization Under Uncertainty
Sponsored Session
Chair: Andrzej Ruszczynski, Rutgers University, 100 Rockafeller Road,
Rutgers Business School, Piscataway, NJ, 08854,
United States of America,
rusz@business.rutgers.edu1 - Risk-averse Control of Markov Chains in Discrete and
Continuous Time
Andrzej Ruszczynski, Rutgers University, 100 Rockafeller Road,
Rutgers Business School, Piscataway, NJ, 08854,
United States of America,
rusz@business.rutgers.eduWe shall consider risk-averse control problems for controlled Markov chains in
discrete and continuous time. The concept of a dynamic risk measure and its of
time consistency will be resined. We shall derive optimality conditions and discuss
solution methods for discrete-time problems. For continuous-time problems, we
shall derive the structure of time-consistent Markov risk measures and optimality
conditions.
2 - Process-based Risk Measures for Observable and Partially
Observable Discrete-time Controlled Systems
Jingnan Fan, Rutgers University, 100 Rockafeller Road, Rutgers
Business School, Piscataway, NJ, 08854, United States of America,
jingnan.fan@rutgers.edu, Andrzej Ruszczynski
For controlled discrete-time stochastic processes we introduce process-based
dynamic risk measures to measure risk of processes. We also introduce a new
concept of conditional stochastic time consistency and we derive the structure of
risk measures enjoying this property. We show that they can be equivalently
represented by a collection of static law-invariant risk measures on the space of
functions of the state space. This structure can be applied to Markov controlled
problems, including POMDP.
3 - Risk-averse Optimal Learning for Clinical Trial Design
Curtis Mc Ginity, Rutgers University, Piscataway, NJ,
United States of America,
curtis.mcginity@rutgers.eduWe formulate the risk-averse optimal learning problem for the exploration vs.
exploitation dilemma in clinical trial design. We establish the class of logistic
toxicity models leading to log-concave posteriors in the Markov model. We then
offer risk-averse approximate dynamic programming methods of the resulting
single- and multistage problems. Finally, we compare performance of prominent
policies for this problem class in terms of multivariate stochastic dominance.
WB12