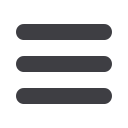

INFORMS Philadelphia – 2015
410
3 - Why Revenue Management is a Good Thing?
Emmanuel Carrier, Delta,
emmanuel.carrier@delta.comFrom its roots in the airline industry, RM has expanded to many industries such
as hospitality, retail and B2B. While they are affecting a growing number of B2C
and B2B transactions, RM practices have become increasingly controversial with
consumers. In this paper, we look at long series of empirical data to show that RM
is a win-win strategy for producers and consumers and leads to higher utilization
rates. We discuss how to keep this legacy alive given the emergence of “big data”
techniques.
4 - A Heuristic Approach to Predicting Customer Lifetime Values for
Apartment Tenants
Jian Wang, Vice President, Research & Development, The
Rainmaker Group, 4550 North Point Parkway, Alpharetta, GA,
30022, United States of America,
jwang@letitrain.comEstimating tenant lifetime values is important for apartment revenue
management. We propose a heuristic approach to predicting renewal likelihoods
and estimating tenant lifetime values. We then present empirical results based on
real apartment data.
WB30
30-Room 407, Marriott
Information Systems I
Contributed Session
Chair: Xu Han, Uconn School of Business, 2100 Hillside Rd, Storrs, CT,
06268, United States of America,
xu.han@business.uconn.edu1 - The Impact of it Maturity and IS Planning Process on IS
Planning Success
Tomoaki Shimada, Associate Professor Of Operations
Management, Kobe University, 2-1 Rokkodai-cho, Nada-ku,
Kobe, 657-8501, Japan,
shimada@b.kobe-u.ac.jp,
Robert De Souza, James Ang, Yoshiki Matsui, Darren Ee
In this study, we examine the impact of information technology (IT) maturity and
information system (IS) planning process on IS planning success. Using data
collected from the self-administrated questionnaire survey, we show complex
relationships between IT maturity and IS planning success as well as between IS
planning process and IS planning success in a non-linear regression approach.
2 - Why Do High-tech Firms Offer Perks at Work?
Xuan Ye, PhD Student, New York University, 44 West 4th ST,
KMEC 8-186, New York, NY, 10012, United States of America,
xye@stern.nyu.edu,Prasanna Tambe
We study whether and why high-tech firms rely more heavily on non-wage
benefits, such as free meals, transportation subsidies, and athletic facilities
(“work-related perks”). We find that employers engaged in IT innovation are
more likely to offer work-related perks. Additionally, we find that high-tech firms
offer work-related perks to attract and motivate IT workers who can quickly
adapt to technological change.
3 - Merger and Acquisitions in it Industry
Kangkang Qi, Michigan State University, 632 Bogue Street,
BCC N204, East Lansing, United States of America,
qikang@broad.msu.eduI study M&A in IT industry. There are three major research questions: (1) Does
making M&A really help IT firms with long term profitability and/or innovation
gains? (2) For each specific M&A, what are the antecedents that are related to
market reaction? (3) Can we attribute the misalignment between firms’
characteristics and M&A decision to CEO overconfidence/narcissism?
4 - Nursing Home Rating System Fraud Detection
Xu Han, Uconn School of Business, 2100 Hillside Rd, Storrs, CT,
06268, United States of America,
xu.han@business.uconn.edu,Niam Yaraghi, Ram Gopal
Potential fraud may exist in the rating procedure of CMS’s Nursing Home
Compare System, leading to misuses of ratings. This study empirically examines
the factors affecting the ratings. We find a significant association between ratings
and profits, pointing to a financial incentive to cheat. We show that this
association does not always lead to legitimate efforts, but can induce cheating. A
prediction model is then developed, and 6% of the suspect nursing homes are
identified as likely cheaters.
WB31
31-Room 408, Marriott
Data Analytics for Manufacturing and Healthcare
Enterprise System
Sponsor: Data Mining
Sponsored Session
Chair: Kaibo Liu, Assitant Professor, UW-Madison, 1513 University
Avenue, Madison, WI, 53706, United States of America,
kliu8@wisc.edu1 - Quantitative Imaging in Medicine
Teresa Wu, Arizona State University, Tempe, AZ, United States of
America,
teresa.wu@asu.eduThe ASU-Mayo Clinic Imaging Informatics Laboratory is a collaborative effort
between the Industrial Engineering program at Arizona State University and the
Department of Radiology at Mayo Clinic Arizona. Our goal is to improve patient
care by analyzing and managing information in radiology images and databases.
In this talk, I will briefly discuss some on-going projects on the use of quantitative
imaging in the clinical context.
2 - Process Execution Monitoring and Controlled Violations
Russell Barton, Senior Associate Dean, Penn State, Smeal College
of Business, 210 Business Building, University Park, PA, 16802,
United States of America,
rrb2@psu.edu,Akhil Kumar
Service processes do not lend themselves to SPC methods common in
manufacturing settings. Monitoring activity timing and activity sequencing
presents special opportunities for statistical characterization, and opportunities for
taking corrective action. Violations in activity timing and/or sequencing are
unavoidable. We show how to monitor a running process, and through constraint
satisfaction find a schedule for its completion to minimize total penalty from the
violations.
3 - Data Driven Approach for Modeling the Coupled Dynamics of
Machine Degradation and Repair Processes
Hoang Tran, Texas A&M University, College, TX,
United States of America,
tran@tamu.edu, Satish Bukkapatnam
We proposed a data driven approach to model the coupled dynamics of recurring
degradation and restoration processes that take place in manufacturing systems.
Unlike previous methods, interactions between the two processes that influence
downtimes and throughput rate can be explicitly considered. Theoretical and
numerical analyses prove that our model can capture multimodal distribution and
dynamic couplings between the time between failures and the time to repair.
WB32
32-Room 409, Marriott
Data Mining in Health Care
Contributed Session
Chair: Hamed Majidi Zolbanin
Oklahoma State University, 309 S. West St, Unit 6, Stillwater, Ok,
74075, United States of America,
hamed.majidi@gmail.com1 - Predicting Inpatient Ward Demand Based on The Emergency
Department Patient Characteristics
Nooshin Valibeig, Northeastern University, 334 Snell engineering,
Boston, 02115, United States of America,
nooshin.valibeig@gmail.com, Jacqueline Griffin
Bed assignment is the process of assigning patients to the targeted ward in a
reasonable time or to an overflow ward when the assignment time increases.
Predicting demand for each ward helps bed managers to decrease assignment time
and overflow assignments which results in lower costs and better quality of care.
In our study we apply data mining methods on historical data of an emergency
department(ED) to predict the probability of inpatient admission and potential
targeted ward for ED patients.
2 - Mining Process Patterns from Noisy Audit Logs with Application
to Emr Systems
He Zhang, Assistant Professor, University of South Florida, 4202
E. Fowler Avenue, CIS1040, Tampa, 33620, United States of
America,
hezhang@usf.edu,Sanjay Mehrotra, David Liebovitz,
Carl Gunter, Bradley Malin
We present a four-step framework to analyze process models with noise. The first
step is to establish correlations among events and separate each trace of access
logs into blocks. These blocks are then clustered into several groups and the
original traces of access logs are transformed to traces consisting of high level
blocks. The traces are then clustered into subgroups, each of which can be used to
analyze the process. We implement our approach using data from a large
academic medical center.
WB30