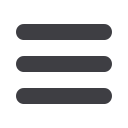

INFORMS Philadelphia – 2015
432
WC17
17-Franklin 7, Marriott
Optimization Network
Contributed Session
Chair: Charles Nicholson, Assistant Professor, University of Oklahoma,
202 W. Boyd St., Room 116-H, Norman, OK, 73071,
United States of America,
cnicholson@ou.edu1 - 1 Refueling Location Problem on a Continuous Tree Network
Sang Jin Kweon, Ph. D. Student, The Pennsylvania State
University, 232 Leonhard Building, The Pennsylvania State
University, University Park, PA, 16802, United States of America,
svk5333@psu.edu, Jose A. Ventura, Seong Wook Hwang
In this talk, we consider a location problem for 1-refueling station on a tree
network. We aim to locate the station anywhere on the network, including along
the edge. Our objective is to locate a 1-refueling station to maximize the total
traffic flow covered in round trips. For this, we derive reduction properties
regarding the problem size and optimality conditions. Then, we develop an exact
algorithm to determine the set of optimal locations for the refueling station.
2 - Near Optimal Design of Wavelength Routed Optical Networks
Prahalad Venkateshan, Associate Professor, Indian Institute of
Management, Ahmedabad, Vastrapur, Ahmedabad, India,
prahalad@iimahd.ernet.in, Yogesh Agarwal
The problem of designing a wavelength routed optical transport network is
considered. Valid inequalities are used to augment traditional network design
formulations. The resulting network is optimal for a majority of the problem
instances tested and in those cases where it is not, a trial-and-error method finds
near-optimal solutions. Computational tests are reported on relatively larger
problem sizes than have been reported in literature on the wavelength routing
problem.
3 - Metrics of Compactness: The Benefits of a Cluster-first,
Route-second Approach to the Min-max K WRPP
Oliver Lum, University of Maryland, 3103 Mathematics Building,
University of Maryland, College Park, MD, 20742, United States
of America,
oliver@math.umd.edu, Bruce Golden, Edward Wasil,
Carmine Cerrone
In practice, it is often desirable for the routes of vehicles to exhibit certain
properties that are not included in the objective function. Two such properties are
compactness and separation. We discuss existing metrics that attempt to capture
these traits, propose a new metric that combines these intuitions into a single
value, and compare computational results for route-first, and cluster-first
heuristics for the Min-Max K Windy Rural Postman.
4 - Optimal Flow Analysis, Prediction and Application
Charles Nicholson, Assistant Professor, University of Oklahoma,
202 W. Boyd St., Room 116-H, Norman, OK, 73071,
United States of America,
cnicholson@ou.edu, Weili Zhang
The fixed charge network flow problem is a classic NP-hard problem with many
applications. To the best of our knowledge, this is the first paper that employs
statistical learning technique to analyze the characteristics of optimal solutions.
We develop an accurate propensity model based on this analysis to predict which
arcs will have positive flow in an optimal solution. This propensity score allows
for multiple applications such as identification of critical components in complex
networks.
WC18
18-Franklin 8, Marriott
Optimization Robust I
Contributed Session
Chair: Zhi Chen, Department of Decision Sciences, PhD B1-02 Biz2
Building, NUS Business School, Singapore, 117592, Singapore,
chenzhi@u.nus.edu1 - Competitive Difference Analysis of One-way Trading with
Limited Information
Yingjie Lan, Peking University, Guanghua School of
Management, Beijing, 100871, China,
ylan@pku.edu.cn,
Wei Wang
We consider robust one-way trading with limited information on price as a game.
Our analysis finds the best guarantee of difference from the optimal performance.
We provide closed-form solution, and reveal for the first time all possible worst
case scenarios. Numerical experiments show that our policy is more tolerant of
information inaccuracy than Bayesian policies, and can earn higher average
revenue than other robust policies while keeping a lower standard deviation.
2 - A Robust Optimization Inventory Model with Uncertain Demand
and Lead Time
Mohammad Rahdar, Iowa State University, 133 University
Village, Unit F, Ames, IA, 50010, United States of America,
rahdar@iastate.edu, Lizhi Wang, Guiping Hu
This study aims to improve the efficiency of a supply chain system by optimizing
the ordering strategy in a manufacturing facility with uncertain demand and lead-
time. A robust optimization model which has tri-level structure is proposed to
explicitly address the uncertainty, and make planning decisions which are robust
against all scenarios. We propose an exact algorithm for the tri-level programming
model and report the numerical results.
3 - An Optimal Solution to a PCA Based Multi-Response Problem
Nasser Fard, Associate Professor, Northeastern University, 334
Snell Engineering Center, 360 Huntington Avenue, Boston, MA,
02115, United States of America,
n.fard@neu.edu,Huyang Xu,
Yuanchen Fang
It is shown that each eigenvalue obtained from the application of PCA in multi-
response optimization has a set of eigenvectors, and different eigenvectors
corresponding to each eigenvalue will lead to different results. A method for
determining the optimal eigenvector combination is proposed. These eigenvectors
are used to calculate the multi-response performance indices, and then the
optimal factor-level combination will be uniquely determined.
4 - Distributionally Robust Optimization with Semi-infinite
Ambiguity Sets
Zhi CHEN, Department of Decision Sciences, PhD B1-02 Biz2
Building, NUS Business School, Singapore, 117592, Singapore,
chenzhi@u.nus.edu,Melvyn Sim, Xu Huan
We investigate semi-infinite ambiguity sets that involve infinite expectation
constraints besides moments and support. We study the associated intractable
distributionally robust optimization by considering relaxed ambiguity sets with
finite constraints. Based on worst-case distribution, we demonstrate an algorithm
that tightens the relaxation gradually. We present expressive examples of these
ambiguity sets, and show examples where the worst-case distribution can be
relatively easy verified.
5 - Robust Optimization for a Location Problem with Codependent
Uncertain Constraints
Juan Carlos Espinoza Garcia, ESSEC Business School,
Av Bernard Hirsch, CS 50105, Cergy-Pontoise, 95021, France,
juancarlos.espinoza@essec.edu, Laurent Alfandari
We consider an uncertain problem where the same parameter is liable to
variation in both constraints and objective function. We model this problem
under the Gamma-Robustness paradigm adapting the framework to include
codependency between the uncertain equations. We apply this model to a
location problem with uncertain demands and conduct computational
experiments on a housing problem.
WC19
19-Franklin 9, Marriott
Heuristics
Sponsor: Computing Society
Sponsored Session
Chair: Michael Hahsler, SMU, P.O. Box 750123, Dallas, TX, 75275,
United States of America,
mhahsler@lyle.smu.edu1 - Heuristic Search of Good Decisions for Evaluating Soft
Constraints in Integer Programs
Steve Kimbrough, University of Pennsylvania, 3730 Walnut
Street, Philadelphia, PA, 19104, United States of America,
kimbrough@wharton.upenn.edu, Monique Guignard-Spielberg,
Frederic Murphy
Parameter sweeping seeks insight into (usually simulation) models by examining
a plurality of model results obtained using a number of judiciously chosen
parameter settings. We introduce decision sweeping (of optimization models), in
which we collect a number of judiciously chosen decisions (feasible and infeasible
settings of the decision variables) from the larger space of decisions. We focus on
the resulting insights, especially with regard to integer programs with soft
constraints.
2 - Ordering Objects: What Heuristic Should We Use?
Michael Hahsler, SMU, P. O. Box 750123, Dallas, TX, 75275,
United States of America,
mhahsler@lyle.smu.eduSeriation, i.e., finding a suitable linear order for a set of objects given data and a
merit function, is a basic combinatorial optimization problem with applications in
modern data analysis. Due to the combinatorial nature of the problem, most
practical problems require heuristics. We have implemented over 20 different
heuristics and more than 10 merit functions. We will discuss the different
methods in this presentation and compare them empirically using datasets from
several problem domains.
WC17