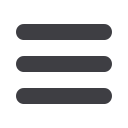

INFORMS Philadelphia – 2015
433
WC21
21-Franklin 11, Marriott
Healthcare Capacity Planning Models
Sponsor: Health Applications
Sponsored Session
Chair: Mike Carter, University of Toronto, Mechanical & Industrial
Engineering, 5 King’s College Rd., Toronto, ON, M5S 3G8, Canada,
mike.carter@utoronto.ca1 - Influence of Surge Capacity Protocols on Hospital Bed
Capacity Planning
Carolyn Busby, PhD Candidate, University of Toronto,
Mechanical & Industrial Engineering, 5 King’s College Rd.,
Toronto, ON, M5S 3G8, Canada,
carolyn.busby@mail.utoronto.ca,
Mike Carter
Management of finite hospital resources changes as hospitals near capacity. As
such, we need to consider these “surge protocols” in order to accurately model
bed capacity needs. Preliminary work is presented on a generalize discrete event
simulation that models inpatient bed usage by medical and surgical patients, as
well as off-servicing rules and surge protocols (ambulance consideration, early
discharge, altered admission rates, cancellation of elective procedures etc.)
2 - Tools to Support Managing Access in the Veterans
Health Administration
Renata Konrad, Worcester Polytechnic Institute, Worcester, MA,
United States of America,
rkonrad@wpi.edu, Sharon Johnson,
Bengisu Tulu
Creating access to health services requires balancing supply and demand. A
capacity cushion must be maintained, representing extra supply to absorb
variability or the result is increased wait time. We describe a tool that links
analysis of clinic data with actionable strategies to reduce variability in supply and
demand in primary and specialty care clinics in the Veterans Health
Administration. The tool is piloted in four clinics and has the potential to improve
decisions surrounding access.
3 - Designing Offload Zones to Reduce Offload Delay
Peter Vanberkel, Professor, Dalhousie University, P.O. Box 15000,
Halifax, Canada,
Peter.VanBerkel@Dal.Ca,Richard Boucherie,
Corine Laan, Alix Carter
We examine the offload zone - an area where multiple patients who arrive by
ambulance can wait allowing the ambulance crew to return to service
immediately. Although a reduction in offload delay was anticipate, it was
observed that the offload zone is often at capacity. In this study we investigate
why this is the case and use a continuous time Markov chain to evaluate
interventions to prevent offload zone congestion.
4 - Analyzing Long-term Care Transition Data with a Multi-state
Semi-markov Model
Hambisa Keno, PhD Candidate, Purdue University, School of
Industrial Engineering, West Lafayette, IN, United States of
America,
hkeno@purdue.edu,Nan Kong, Steven Landry,
Mark Ward, Wanzhu Tu, Chris Callahan
Capacity reconfiguration between nursing homes and home-and-community-
based settings is a challenging decision for long-term care delivery. A good
indicator to the capacity requirement in these facilities is length of stay. Semi-
Markov models have been used to characterize patient flows in a single care
facility. We extend these models to the context of multi-facility care networks.
Further, we embed higher-order Markov chains to assess the impact of care
pathway on the model fitting.
WC22
22-Franklin 12, Marriott
Learning and Queues
Sponsor: Applied Probability
Sponsored Session
Chair: Ricky Roet-Green, University of Toronto, 37 zola gate, Thornhill,
L4J9A7, Canada,
rgricky@gmail.comCo-Chair: Michael Kim, University of Toronto, 5 King’s College Road,
Toronto, Canada,
mikekim@mie.utoronto.caCo-Chair: Andrew Lim, National University of Singapore/Department
of Decision Sciences, Mochtar Riady Building, BIZ1 08-69, 15 Kent
Ridge Drive, Singapore, Singapore,
andrewlim@nus.edu.sg1 - The Armchair Decision: to Depart Towards the Queue or Not
Ricky Roet-Green, University of Toronto, 37 Zola Gate, Thornhill,
L4J9A7, Canada,
rgricky@gmail.com, Refael Hassin
Consider a GPS user that inspects the traffic from his armchair at home. Given the
expected delays, would she drive to the service facility? A common assumption in
queueing models is that there is no time gap between observing the queue and
joining it. We challenge it by allowing the queue to evolve while the customer is
on her way. We show that as opposed to intuition, customers who balk when the
queue is mid-congested departs towards it when it is highly congested.
2 - From Product Form Queues to Queue Decomposition:
The State-dependent Mn/gn/1 Example
Opher Baron, University of Toronto, 105 St. George St, Toronto,
ON, Canada,
opher.baron@rotman.utoronto.ca,
Hossein Abouee Mehrizi
In the analysis of Product Form compatible queueing systems we decomposed
them into subsystems that can be analyzed independently of each other.
Queueing decomposition (QD) was used to approximate solutions for other
queueing networks. We formulate QD and discuss its implementation for exact
analysis. We demonstrate QD for many systems focusing on Mn/Gn/1 where
arrivals and service times are state dependent and service rates can change at
arrivals and departures is analyzed.
3 - Rational Abandonment from Priority Queues:
Equilibrium Strategy and Pricing Implications
Vahid Sarhangian, University of Toronto, 105 St. George Street,
Toronto, ON, Canada,
vahid.sarhangian11@rotman.utoronto.ca,
Philipp Afeche
The literature on the economics of queues predominantly focuses on the queue-
joining decisions of customers and ignores subsequent abandonment decisions.
Such abandonment behavior is particularly important in priority queues, which
are quite prevalent in practice. We study the equilibrium joining and
abandonment behavior of utility-maximizing customers in the context of an
observable two-class priority queue and identify important pricing implications.
WC23
23-Franklin 13, Marriott
Control of Queues
Sponsor: Applied Probability
Sponsored Session
Chair: Douglas Down, McMaster University, 1280 Main Street West,
Hamilton, Canada,
downd@mcmaster.ca1 - ATM Replenishment Scheduling
Yu Zhang, UNC Chapel Hill, B04 Hanes Hall, UNC Campus,
Chapel Hill, NC, 27599, United States of America,
yuzhang@live.unc.edu, Vidyadhar Kulkarni
We develop an ATM replenishment policy for a bank that operates multiple ATMs
with an aim to minimize the cost of stock-outs and replenishments, taking into
account the economies of scale involved in replenishing multiple ATMs
simultaneously. We present the structure of the optimal strategy that minimizes
the long run cost per unit time and study a heuristic policy which is easy to
implement.
2 - An Emergency Department Resource Allocation Model for
Patients of Deteriorating Health
Mark Lewis, Professor, Cornell University, Rhodes Hall, Ithaca,
NY, 14853, United States of America,
mark.lewis@cornell.edu,Douglas Down, Carri Chan
We consider the allocation of medical service providers (MSPs) when patients
health continues to deteriorate while waiting for service. The decision maker
must balance the need to see more severely injured patients with the need to not
allow those that are injured to continue to deteriorate. Conditions are provided
when to prioritize each patient class.
3 - Robust Performance and Optimization of a Series Queue
Michael Veatch, Gordon College, 255 Grapevine Rd, Dept. of
Mathematics, Wenham, MA, 01984, United States of America,
Mike.Veatch@gordon.eduRobust optimization (RO) is conservative, but when Central Limit Theorem
uncertainty sets are used in an RO queue, the result is optimistic. We explain
why, and propose a correction based on a diffusion model of a queue with general
arrival and service times. For a series queue, the method is tractable for
performance analysis and for optimal allocation of server capacity.
4 - Service Rate Control of an On/Off Server
Douglas Down, McMaster University, 1280 Main Street West,
Hamilton, Canada,
downd@mcmaster.ca, Guang Mo,
Vincent Maccio
We study a server that may be turned on and off, where there is a delay to turn
on the server. In addition, the service rate of the server can be chosen from a
finite set. Using a cost function that involves server usage and holding costs, we
discuss the following questions. Under what conditions should the server never
be turned off? If it is advantageous to turn off the server, when should it be
turned off? When the server is on, what speed should be chosen?
WC23