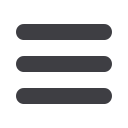

INFORMS Philadelphia – 2015
483
2 - Novel Sampling Technique for High Dimensional Stochastic
Optimization Problem
Nishant Dige, Graduate Student Industrial Engineering,
University of Illinois at Chicago, 1200 W Harrison Street,
Chicago, IL, 60607, United States of America,
ndige2@uic.edu,Urmila Diwekar
Computational speed is critical in optimizing large scale stochastic problems and
the major bottleneck is the computational intensity of samples. For sampling
randomness is important but uniformity governs its accuracy. This paper presents
a novel sampling approach; combining LHS & Sobol Sampling for better
uniformity in single as well as multi dimensions & also to avoid clustering effect
for higher dimensions. We have implemented this technique on stochastic supply
chain network design problem.
3 - Inventory Management Based on Target-oriented
Robust Optimization
Yun Fong Lim, Associate Professor, Singapore Management
University, 50 Stamford Road, #04-01, Singapore, 178899,
Singapore,
yflim@smu.edu.sg,Chen Wang
We propose a target-oriented robust optimization approach to solve a multi-
product, multi-period inventory problem subject to capacity constraints. The
product demands are characterized by uncertainty sets. We find an ordering
policy that maximizes the uncertainty sets such that all demand realizations from
the sets result in a cost lower than a pre-specified target. We prove that a static
policy is optimal and it can achieve a balance between the expected cost and the
associated cost variance.
4 - Optimal Learning of Demand for The Nested Lagged
Commitment Problem
Nana Aboagye, PhD Candidate, Princeton University,
Sherrerd Hall, Charlton Street, Princeton, NJ, 08544,
United States of America,
aboagye@princeton.edu,
Warren Powell
We address the problem of making lagged commitments to resources in order to
maximize revenue over time while sequentially making decisions. The motivating
application is hotel resource management and a separate dimension involves
learning how the market will respond to price. We consider two cases: where
demand is unknown but static and where demand is unknown and dynamic. We
use the optimization algorithm called the Knowledge gradient to learn the
optimal demand function.
5 - Continuity of Robust Optimization Problems with Respect to the
Uncertainty Set
Philip Allen Mar, Dept. of MIE, University of Toronto,
5 King’s College Road, Toronto, ON, M5S 3G8, Canada,
philip.mar@mail.utoronto.ca,Timothy Chan
We discuss the stability properties of robust problems satisfying the Strong Slater
condition, with respect to their uncertainty sets. We show, by way of results in
Linear Semi-Infinite Optimization, that the optimal values of the robust
optimization problem are Lipschitz continuous with respect to the Hausdorff
distance between their respective uncertainty sets. We also present implications
for measuring a price of robustness and approximating robust optimization with
complex uncertainty sets.
WE14
14-Franklin 4, Marriott
Risk-Aware Decision Making under Uncertainty
Sponsor: Optimization/Optimization Under Uncertainty
Sponsored Session
Chair: Ruiwei Jiang, University of Michigan, 1205 Beal Ave., Ann
Arbor, MI, 48109, United States of America,
ruiwei@umich.edu1 - A Composite Risk Measure Framework for Decision Making
under Uncertainty
Pengyu Qian, Columbia University, Columbia Business School c/o
PhD Office, 3022 Broadway,311 Uris Hall, New York, NY, 10027,
United States of America,
qianpengyu@pku.edu.cn, Zaiwen Wen,
Zizhuo Wang
In this talk, we present a unified framework for decision making under
uncertainty. Our framework is based on the composite of two risk measures
accounting for parametric (given distribution) and distributional uncertainty
respectively. The framework generalizes many existing models. We also propose
new models within this framework whose solutions have probabilistic guarantees
and are less conservative comparing to traditional models. Numerical experiments
demonstrate the strength of our models.
2 - Risk-averse Two-stage Stochastic Program with
Distributional Ambiguity
Ruiwei Jiang, University of Michigan, 1205 Beal Ave., Ann Arbor,
MI, 48109, United States of America,
ruiwei@umich.edu, Y
ongpei Guan
We develop a risk-averse two-stage stochastic program (RTSP) taking into account
the distributional ambiguity. We derive an equivalent reformulation for RTSP that
applies to both discrete and continuous distributions. Also, the reformulation
reflects its linkage with a full spectrum of coherent risk measures under varying
data availability.
3 - Risk Sharing in Classification Problems
Constantine Vitt, PhD Candidate, Rutgers University,
1 Washington Park, Newark, NJ, 07102,
United States of America,
constantine.vitt@rutgers.edu,
Darinka Dentcheva, Hui Xiong
We develop a new approach to solving classification problems based on the theory
of coherent measures of risk and risk sharing. We view labeled training data as
random samples from populations with unknown distributions, subject to change.
The key idea of the proposed methodology is to associate individual measures of
risk with the misclassification of each class. We analyze the problem theoretically
and propose a numerical method to identify the proper risk sharing among the
classes.
WE15
15-Franklin 5, Marriott
Optimization Methodology II
Contributed Session
Chair: Vahid Nourbakhsh, PhD Student, UC Irvine, The Paul Merage
School of Business, The Paul Merage School of Business, University of
California-Irvine, Irvine, CA, 92697, United States of America,
vahidn@uci.edu1 - Reliability Optimization for Multi-components System in the
Design Phase
Qianru Ge, PhD Candidate, Technology University of Eindhoven,
Paviljoen E.03, IE&IS, Eindhoven, 5612 AZ, Netherlands,
q.ge@tue.nlWe develop an optimization model to determine the optimal failure rate of critical
components in a system. Since the system is under a service contract, a penalty
cost should be paid by the OEM when the total system down time exceeds a
predetermined level, which complicates the evaluation of the life cycle costs.
Furthermore, in the design phase for each critical component, the failure rate can
be chosen from a certain range.
2 - Multistage News Vendor Problem with Targets
Vishwakant Malladi, Doctoral Student, UT Austin, Austin, TX,
78703, United States of America,
Vishwakant.Malladi@phd.mccombs.utexas.eduWe analyze the optimal control policy of a multi-stage new vendor problem with
targets. The results show a tractable and intuitive control policy for each stage.
3 - Efficient Methodology to Maximizing Total Noise Reduction and
Minimize Total Cost in Traffic Design
Golshan Madraki, PhD Candidate, Ohio University,
15 Station St. Apt F, Athens, Oh, 45701,
United States of America,
g.madraki@gmail.comThree crucial variables that affect the efficiency of a Traffic noise barrier are: the
distance from receivers, height of the barrier and the material of the barrier. A
novel combination of methodologies is proposed to maximize the efficiency of the
barrier with minimum cost. An example model of the barriers are simulated by
TNM2.5 software used to perform Factorial design of experiment to fit a meta-
model. LP-metric method is applied to solve the multi-objective math-model.
4 - On Solving Bi-level Programming Problem with Fuzzy Random
Variable Coefficients
Vishnu Pratap Singh, Research Scholar, Indian Institute of
Technology Kharagpur, Department of Mathematics, Kharagpur,
721302, India,
vishnupratapsingh56@gmail.com,
Debjani Chakraborty
This paper represents the bi-level linear programming problem in an imprecise
and uncertain mixed environment. The aim of this paper is to introduce leader
and the follower’s demand as fuzzy random variable. To determine the optimal
value of the leader and follower’s objectives a new methodology is developed for
bi-level linear programming model in presence of fuzzy random variable.
A numerical example is solved to demonstrate the methodology.
WE15