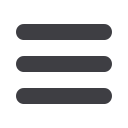

INFORMS Philadelphia – 2015
488
2 - Use the Whole Buffalo: Binary Classification which Encounters
Diverse Data Types
David Elkind, National Security Consultant & Statistical Modeler,
Novetta Solutions, 1320 N Veitch St, Arlington, VA, 22201,
United States of America,
delkind@novetta.comContemporary data collection gathers many different data formats, yet
conventional analysis views data from a flat perspective in which an observation
is a single unit type. This perspective leaves some data types unexploited when
they cannot be made to fit a single paradigm. We propose to use model stacking
to make predictions informed by all available data types. We accomplish this by
using several SVMs, each of which has a kernel function appropriate to the subset
of features it is learning.
3 - Big Data and Causality
Xuan Wang, Graduate Student, Louisiana State University - ISDS
Department, 2200 Business Education Complex, Nicholson
Extension, Baton Rouge, LA, 70803, United States of America,
xwang35@lsu.edu,Helmut Schneider
In the past decade, Big Data Analytics has mainly focused on data mining to make
better predictions. This research explores analytical techniques to discover causal
relationships and focuses on challenges of interpreting correlational relationships
in big data and discusses methods that help to distinguish between correlational
and potential causal effects.
WE30
30-Room 407, Marriott
Information Systems IV
Contributed Session
Chair: Shalini Wunnava, Assistant Professor, SUNY Potsdam,
44 Pierrepont Avenue, 209 Dunn Hall, Potsdam, NY, 13676,
United States of America,
wunnavss@potsdam.edu1 - Crowdsourcing and Project Management
Michael Chuang, SUNY, 1 Hawk Dr, New Paltz, NY,
United States of America,
mikeychuang@gmail.comCrowdsourcing has been increasingly employed in today’s projects, and has
become a phenomenon. Issues of crowdsourcing also demonstrate in various
facets, such as innovation and technology. However, there lacks a comprehensive
understanding of crowdsourcing’s potentials for projects. To bridge the gap, this
research aims at conducting a systematic review of literature, in hopes of better
understanding crowdsourcing for project management.
2 - The Effect of Project Artifacts, Ambidexterity, and Social Network
on Open Source Project Success
Ram Kumar, Professor, UNC-Charlotte, 203B Friday Building,
9201 University City Boulevard, Charlotte, NC, 28223,
United States of America,
rlkumar@uncc.edu, Orcun Temizkan
Open Source Software (OSS) development is a rapidly emerging, yet poorly
understood type of software development with extremely high project failure
rates. We present a model of OSS project success by integrating multiple theories
from social networking, innovation, and organizational theory. Our results
illustrate that ambidexterity along with artifact development success and social
network characteristics influences project success and highlight the bridging role
of ambidextrous developers
3 - Leveraging On-demand Markets to Manage a Hybrid Workforce
for IT Service Delivery
Su Dong, Assistant Professor, Fayetteville State University, School
of Business and Economics, 1200 Murchison Road, Fayetteville,
NC, 28311, United States of America,
sdong@uncfsu.edu,
Monica Johar, Ram Kumar
Organizations increasingly have access to temporary workers through markets for
on-demand workers. We present a model of a hybrid workforce in which
organizations effectively assign tasks to a mix of full-time and on-demand
workers. Full-time workers have to perform assigned tasks. Temporary (on-
demand) workers bid for tasks that are beneficial to them. Issues relating to
pricing, task allocation and knowledge management are explored.
4 - Designing Quality Control Tools for Enhanced Cyber-security in
Manufacturing
Ahmed Elhabashy, Graduate Student, Virginia Tech, 114 Durham
Hall, 1145 Perry Street, Blacksburg, VA, 24061, United States of
America,
habashy@vt.edu,William Woodall, Lee Wells,
Jaime Camelio
Manufacturing relies heavily upon the use of Quality Control tools to detect
quality losses and to ensure high quality parts production. However, current tools
are not designed to detect the effects of cyber-attacks, as they are based on
assumptions (sustained shifts, rational sub-grouping, etc.) that may no longer be
valid under the presence of an attack. The goal of this research is to design/adapt
current Quality Control tools by adopting principles from the Information
Technology domain.
5 - Biometrics: Adoption and Attitudes
Shalini Wunnava, Assistant Professor, SUNY Potsdam,
44 Pierrepont Avenue, 209 Dunn Hall, Potsdam, NY, 13676,
United States of America,
wunnavss@potsdam.eduBiometrics has become ubiquitous on present day digital devices; although the
usage is not very widespread currently, it is expected to see exponential growth in
the near future. What attitudes are fueling the adoption of biometrics? This
research question will be examined using the lenses of protection motivation
theory and technology acceptance model.
WE31
31-Room 408, Marriott
Statistical Roles in Stochastic Decision-Making
Sponsor: Data Mining
Sponsored Session
Chair: Victoria Chen, The University of Texas at Arlington, Dept. of
Ind., Manuf., & Sys. Engr., Campus Box 19017, Arlington, TX, 76019,
United States of America,
vchen@uta.edu1 - A Data-driven Optimization of Price, Promotion, Display, and
Feature at Product Category Level
Durai Sundaramoorthi, Lecturer In Management, Washington
University in St. Louis, One Brookings Drive, Olin Business
School, St. Louis, MO, 63131, United States of America,
sundaramoorthi@wustl.edu, Seethu Seetharaman
Data Mining can be broadly classified into two groups: supervised learning and
unsupervised learning. Supervised learning is the subject of interest in this
research as we deal with predicting the units of category-level products sold in
stores of a grocery chain. The goal of this research is two-fold. First, prediction
models are developed to predict demand. Second, optimum vector X is
determined to maximize the profit made by the chain.
2 - High-dimensional Adaptive Dynamic Programming with Mixed
Integer Linear Programming
Zirun Zhang, University of Texas, Arlington, 500 West First Street,
Arlington, TX, 76019, United States of America,
zhang.zirun@gmail.com, Victoria Chen, Jay Rosenberger
This study addresses the optimization of a real world, complex, dynamic system.
The objective is to control the environmental impact of aircraft deicing activities
at the Dallas-Fort Worth International Airport. To overcome the complexities such
as nonlinear transitions, non-convex objective function, and high-dimensional
decision space, an ADP method is introduced using treed regression and MILP.
The proposed ADP approach is also compared with a reinforcement learning
approach.
3 - Efficient Simulation-based Sampling for Approximate
Dynamic Programming
Danilo Macció, National Research Council of Italy (CNR-ISSIA),
Via De Marini 6, Genova, 16153, Italy,
ddmach@ge.issia.cnr.it,
Victoria Chen, Cristiano Cervellera
We propose a method to generate efficient state sample points for the solution of
continuous-state finite-horizon approximate dynamic programming problems.
The method is based on the notion on F-discrepancy, which measures how closely
a set of points follows a given distribution. The proposed algorithm can be used as
an alternative to uniform random sampling when it is difficult to define a priori
the state boundaries. Simulation results confirm in practice the effectiveness of
the method.
4 - Continuous-state Adaptive Dynamic Programming
Prashant Tarun, Associate Professor, Missouri Western State
University, Craig School of Business, 4525 Downs Drive,
St. Joseph, MO, 64507, United States of America,
ptarun@missouriwestern.edu, Victoria Chen, Huiyuan Fan
We present a sequential state space exploration (SSSE) approach to adaptively
adjust the state space ranges for the experimental design while also sampling
useful data for the statistical model. The SSSE approach is coupled with an
adaptive value function approximation (AVFA) algorithm that gradually grows
the complexity of the statistical model as more data are observed.
WE30