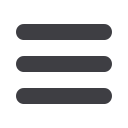

INFORMS Philadelphia – 2015
87
SB63
63-Room 112B, CC
Doing Good with Good OR I
Cluster: Doing Good with Good OR
Invited Session
Chair: Lisa Maillart, University of Pittsburgh, Pittsburgh, PA,
lisa.maillart@engr.pitt.eduCo-Chair: Itai Ashlagi, MIT, 100 Main St, Cambridge, MA, 02139,
United States of America,
iashlagi@mit.edu1 - Optimal Policy Design to Motivate Blood Donation: Evidence from
a Randomized Field Experiment and a Structural Model
Tianshu Sun, University of Maryland Smith School of Business,
3330 Van Munching Hall, College Park, MD, 20740-2840,
United States of America,
tianshusun@rhsmith.umd.eduUsing a randomized field experiment involving 80,000 participants, we test the
effect of different policies in driving donation. We find: blood banks can use group
reward to motivate group formation to increase donation; such group reward is
four times more cost effective than individual reward. We build a structural
model and perform simulations to identify optimal incentive and targeting
strategy.
2 - Improving Blood Collection Policies for Cryoprecipitate
Chenxi Zeng, Georgia Tech, Atlanta, GA, United States of
America,
czeng8@gatech.edu, Turgay Ayer, Chelsea White Iii,
Roshan Vengazhiyil, John Deshane
Working closely with the American Red Cross (ARC), we have developed and
analyzed a donor collection model for whole blood that is to be processed into
cryoprecipitate (cryo), a critical blood product for controlling massive
hemorrhaging. Our numerical results show that the proposed solution approaches
may reduce the expected cryo collection cost by up-to 70%, compared with the
current practice. Implementation of the model at the ARC supports our estimates.
3 - Ebola Treatment Facility Location Planning in Guinea
Chu Qian, Georgia Tech, GA, United States of America,
qianchu31@gmail.com, Charmaine Chan, Matt Daniels,
Javiera Javieria, Caleb Mbuvi, Ivan Renaldi, Jonathan Sutomo,
Kimberly Adelaar
In the recent Ebola outbreak, treatment facilities were critical but beds were
unavailable in some areas while unused in others. A spatial simulation was built
to project the spread within Guinea, overlaid with heuristics on when and where
to place treatment facilities. Results showed units set up quickly or in advance
could have saved more than 2000 lives.
SB64
64-Room 113A, CC
Applications of Decision Analysis & Large-Scale
Data Analytics
Sponsor: Decision Analysis
Sponsored Session
Chair: Mazen Skaf, Partner & Managing Director, Strategic Decisions
Group, 745 Emerson St, Palo Alto, Ca, 94301, United States of America,
mskaf@sdg.com1 - Expert Calibration and Elicitation for Large Scale
Investment Decisions
Saurabh Bansal, Assistant Professor, Penn State University, 405
Business Building, University Park, PA, 16802, United States of
America,
sub32@psu.edu, Genaro Gutierrez
We discuss a new approach for the use of expert calibration and elicitation for
estimating probability distributions. The approach has been in use since last two
years at a Fortune 500 firm for making an annual large-scale investment decision.
Practical insights for expert elicitation are also discussed.
2 - Reducing Risk and Improving Incentives in Funding Entrepreneurs
Samuel E. Bodily, Professor, Darden Graduate Business School,
Univ. of Virginia, 100 Darden Boulevard, Charlottesville, VA,
22903, United States of America,
BodilyS@Darden.virginia.eduBacker financing mechanisms that encourage an entrepreneur are identified, risk
analysis models are developed, and insights are obtained about how mechanisms
(e.g. equity capital, incentive gifts, insurance, revenue contracts, and derivative
swaps) can best reduce risk to the entrepreneur and give proper incentives, at
given cost to the backers. Attention is given to avoiding problems of moral hazard
and providing proper incentives. Certainty equivalents for financing alternatives
are derived.
3 - Using Decision Analysis and Large Data Analytics to Enhance
Decision Quality
Mazen Skaf, Partner & Managing Director, Strategic Decisions
Group, 745 Emerson St, Palo Alto, CA, 94301,
United States of America,
mskaf@sdg.comIncreasingly, in multiple domains, the use of large data analytics is enhancing the
way we apply DA from identifying a decision opportunity, to framing, generating
new alternatives, and evaluating alternatives. We present cases from various
industries to illustrate how using large data analytics contributes to decision
quality and how, in some situations enables a totally new approach to alternatives
generation and strategy development.
SB65
65-Room 113B, CC
Quantifying Uncertainty in Decision Analysis Practice
Sponsor: Decision Analysis
Sponsored Session
Chair: Christopher Hadlock, PhD Student, The University of Texas at
Austin, 1 University Station Austin, TX 78713,
United States of America,
cchadlock@austin.utexas.edu1 - A Theory of Tail Behavior for Decision Analysis
Brad Powley, Senior Consultant, Strategic Decisions Group, 745
Emerson Street, Palo Alto, CA, 94301, United States of America,
bpowley@sdg.comOn occasion, it is useful for a decision analyst to encode a decision maker’s
probability distribution with one or more infinite tails. However, an infinite tail of
one probability distribution might be heavier than that of another, adding a
wrinkle to probability encoding. This talk defines heavier tails and introduces a
theory of tail behavior tailored to help a decision analyst encode a probability
distribution with one or more infinite tails.
2 - Assessment Error and Discrete Approximations to
Continuous Distributions
Robert Hammond, Decision Analyst, Chevron, 1400 Smith St,
Houston, TX, United States of America,
rhammond@chevron.com,Eric Bickel
Decision analyses often use continuous probability distributions, elicited from
subject matter experts, and discretized for use in decision trees. In practice,
cognitive biases reduce the precision of the assessments, and discretization
introduces approximation error. We compare several discretization methods when
the assessments are imprecise, and show that assessment precision often has
significantly more impact on model performance than accuracy tradeoffs between
discretization methods.
3 - Decision Making under Incomplete Information:
Sequential Probability Assessment Heuristics
Tao Huang, The University of Texas at Austin, 204 E. Dean
Keeton Street, Stop C2200, ME department, ETC II, Room 5.152,
Austin, TX, 78712, United States of America,
huangt55@gmail.com, Eric Bickel
Expected utility of an alternative is not explicitly computable in cases where
probability mass function is partially known. Previous research has tried to
establish dominance to solve the problem. In practice, however, dominance is
rarely established and further probability assessments are needed. In this paper,
we propose a novel way called Sequential Probability Assessment Heuristic that
iteratively selects a feasible assessment based on a method from machine learning
to solve this problem.
4 - Assessment-adaptive Discretizations (AAD) for Decision
Analysis Practice
Christopher Hadlock, PhD Student, The University of Texas at
Austin, 1 University Station Austin, TX 78713, United States of
America,
cchadlock@austin.utexas.edu, Eric Bickel
It is common practice in decision analysis to discretize continuous uncertainties
into several points, and then assign probabilities to these points. Shortcuts pre-
specify percentiles to assess, along with the probabilities to assign to each of them.
However, shortcuts optimized towards matching the mean often differ from those
optimized to match the variance. We present assessment-adaptive discretization
methods, which adapt the probability assignments based upon the observed
assessments.
SB65