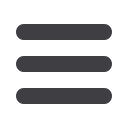

S462
ESTRO 36
_______________________________________________________________________________________________
Purpose or Objective
Artificial neural networks (ANNs) were used in the last
years for the development of models for the prediction of
radiation-induced toxicity following RT. In fact, ANNs are
powerful tools for pattern classification in light of their
ability to model extremely complex functions and huge
numbers of data. However, their major counterpoint is
that in some specific cases they might not deliver realistic
results due to their missing critical capacity. The objective
of this study was to develop a method for assessing
reliability of ANNs response over the entire range of
possible input variables. In particular, in this study the
method was applied to the selection of an ANN for the
prediction of late faecal incontinence (LFI) following
prostate cancer RT.
Material and Methods
The analysis was carried out on 664 patients (pts) of two
multicentre trials. The following information was
available for each pt: i) self completed pt reported
questionnaire (PRO) for LFI determination, ii) clinical data
(co-morbidity, previous abdominal surgery and use of
drugs), iii) dosimetric data (DVH and mean dose).
Several feed-forward ANNs with a proper balance between
complexity and number of training cases were developed,
with input variables and hidden neurons ranging between
3 and 5. Once the best ANNs were obtained, a method was
developed and applied to verify the reliability of their
response over the entire range of possible input variables.
The method consists in the development of a virtual
library of variables covering all the possible
ranges/permutations of continuous/discrete inputs. These
are all classified and penalties (pen) are assigned if ANN
outputs are not coherent with the real world expectance
(i.e., decreasing LFI probability with increasing dose to
the rectum).
Results
More than 1,000,000 different ANN configurations (i.e.,
architecture and internal weights and thresholds) were
developed. For the 200 ANNs showing the best
performance, area under the ROC curve (AUC), sensitivity
(Se), specificity (Sp) and pen were quantified. The best
ANN in terms of classification capability (i.e. AUC=0.79,
Se=74%, Sp=72%) was an ANN with 5 inputs (i.e., mean
dose, use of antihypertensive, previous presence of
haemorrhoids, previous colon disease, hormone therapy)
and 5 hidden neurons. However, the application of the
method to investigate its coherence with the real life
classification expectancy resulted in pen=3, indicating
that this wasn’t the most 'intelligent” ANN to select. The
best ANN with pen=0 was a less complex ANN (i.e. 3 inputs,
5 hidden neurons), resulting in AUC=0.67, Se=70%, Sp=57%.
Conclusion
A new method consisting in the development of a virtual
library of cases was established to evaluate ANN reliability
after its training process. Application of this method to
the development of an ANN for LFI prediction following
prostate cancer RT allowed us to select an ANN with the
best generalization capability.
PO-0852 External validation of a TCP model predicting
PSA relapse after post-prostatectomy Radiotherapy
S. Broggi
1
, A. Galla
2
, B. Saracino
3
, A. Faiella
3
, N. Fossati
4
,
D. Gabriele
5
, P. Gabriele
2
, A. Maggio
6
, G. Sanguineti
3
, N.
Di Muzio
7
, A. Briganti
4
, C. Cozzarini
7
, C. Fiorino
1
1
IRCCS San Raffaele Scientific Institute, Medical Physics,
Milano, Italy
2
Candiolo Cancer Center -FPO- IRCCS, Radiotherapy,
Candiolo Torino, Italy
3
Regina Elena National Cancer Institute, Radiotherapy,
Roma, Italy
4
IRCCS San Raffaele Scientific Institute, Urology, Milano,
Italy
5
University of Sassari, Radiotherapy, Sassari, Italy
6
Candiolo Cancer Institute -FPO- IRCCS, Medical Physics,
Candiolo Torino, Italy
7
IRCCS San Raffaele Scientific Institute, Radiotherapy,
Milano, Italy
Purpose or Objective
A Poisson-based TCP model of 5-year biochemical
recurrence-free survival (bRFS) after post-prostatectomy
radiotherapy (RT) was previously introduced: best
parameters values were obtained by fitting a large (n=894
≥pT2, pN0, hormone-naïve patients) multi-centric
population including data from five prospective /
Institutional series; a satisfactory internal validation was
performed. Current investigation dealt with an
independent external validation on a large group of
patients pooled from two independent Institutional
databases with a minimum follow-up of 3 years.
Material and Methods
Based on the original model, bRFS may be expressed as: K
x (1-exp(-αeff D))
CxPSA
where: D is the prescribed dose; αeff
is the radiosensitivity factor; C is the number of clonogens
for pre-RT PSA=1ng/ml, assuming PSA to be proportional
to tumor burden; K (equal to 1-BxPSA) is the fraction of
patients who relapse due to clonogens outside the treated
volume, depending on pre-RT PSA and Gleason Score (GS).
The model works well when grouping patients according
to their GS value: best-fit values of αeff (range: 0.23-
0.26), C (10
7
) and B (0.30-0.50) were separately derived
for patients with GS<7, GS=7 and GS>7. For current
external validation, data of 352 ≥pT2, pN0, hormone-naïve
patients treated with conventionally fractionated
adjuvant (175) or salvage (177) intent after radical
prostatectomy were available from two Institutions not
previously involved in the training data set analysis. The
predicted risk of 5-year bRFS was calculated for each
patient, taking into account the slope and off-set of the
model, as derived from the original calibration plot. Five-
year bRFS data were compared against the predicted
values in terms of overall performance, calibration and
discriminative power.
Results
The median follow-up time, pre-RT PSA and D were 83
months (range: 36-216 months), 0.28 ng/mL (0.01-9.01
ng/mL) and 70.2Gy (66–80Gy); the GS distribution was:
GS<7: 118; GS=7: 185; GS>7: 49. The performances of the
model were excellent: the calibration plot showed a
satisfactory agreement between predicted and observed
rates (slope: 1.02; R
2
=0.62, Figure 1). A moderately high
discriminative power (AUC=0.68, 95%CI:0.62-0.73) was
found, comparable to the AUC for the original data set
(0.69, 95%CI:0.66-0.73). The predicted 5-year bRFS for the
whole population assessed as the weighted average of the
values referred to the three groups (i.e.: GS<7, =7, >7)
was 67%, compared to an observed 5-year bRFS equal to
68% ± 5% (95%CI). The agreement was slightly worse in the
GS<7 group (70% vs 79% ± 7%) compared to GS=7 (66% vs
66% ± 7%) and GS>7 (62% vs 51% ± 14%).