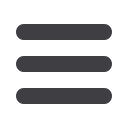

S860
ESTRO 36
_______________________________________________________________________________________________
EP-1595 NTCP models for early toxicities in patients
with prostate or brain tumours receiving proton therapy
A. Dutz
1,2
, L. Agolli
1,3
, E.G.C. Troost
1,2,3,4,5
, M.
Krause
1,2,3,4,5
, M. Baumann
1,2,3,4,5
, A. Lühr
1,2,3,4
, S. Löck
1,3,5
1
OncoRay - Center for Radiation Research in Oncology,
Faculty of Medicine and University Hospital Carl Gustav
Carus- Technische Universität Dresden, Dresden,
Germany
2
Helmholtz-Zentrum Dresden-Rossendorf, Institute of
Radiooncology, Dresden, Germany
3
Department of Radiation Oncology, Faculty of Medicine
and University Hospital Carl Gustav Carus- Technische
Universität Dresden, Dresden, Germany
4
German Cancer Research Center DKFZ, Heidelberg and
German Cancer Consortium DKTK partner site Dresden,
Dresden, Germany
5
National Center for Tumor Diseases, partner site
Dresden, Dresden, Germany
Purpose or Objective
To identify patients who are likely to benefit most from
proton therapy, based on the potential reduction in
normal tissue complication probability (NTCP) compared
to photon therapy. The NTCP models required for this
comparison were developed using clinical data on early
side effects for patients with brain or prostate cancer
having received proton therapy.
Material and Methods
Eighty patients with primary brain tumours and 30 patients
with adenocarcinoma of the prostate who received proton
therapy were included in this study. For the brain tumour
patients, the radiation-induced early toxicities alopecia,
erythema, pain and fatigue were considered, while for
prostate cancer proctitis, diarrhoea, urinary frequency,
urgency and incontinence, obstructive symptoms and
radiation-induced cystitis were investigated. The
occurrence of these side effects was correlated with
different dose-volume parameters of associated organs at
risk. NTCP models were created using logistic regression.
A retrospective comparative treatment planning study was
conducted to predict the potential reduction in NTCP of
proton therapy compared to volumetric modulated arc
therapy using the created models. For patients with brain
tumours different subgroups were defined to identify
patient groups which show a particularly high reduction in
the considered toxicities.
Results
For patients with primary brain tumours significant
correlations between the occurrence of alopecia grade 2
as well as erythema grade ≥ 2 and the dose-volume
parameters D5% and V25Gy of the skin were found. Plan
comparison showed an average reduction in NTCP for
alopecia grade 2 of more than 5 % (see figure) and for
erythema grade ≥ 2 of about 5 % using proton therapy. For
patients with a brain tumour located in the skull base,
with a clinical target volume less than 115 cm³ or with a
prescribed dose less than 60 Gy, a potential reduction in
NTCP for alopecia grade 2 of about 10 % could be achieved.
For patients with prostate cancer significant correlations
between obstructive symptoms grade ≥ 1 and the dose
parameter D30% of the bladder as well as radiation-
induced cystitis grade ≥ 1 and D20% of the bladder were
found. Plan comparison showed an average reduction in
NTCP for obstructive symptoms ≥ grade 1 of about 25 %
and for radiation-induced cystitis about 15 % using proton
therapy.
Conclusion
We found significant correlations between the occurence
of early toxicities and dose-volume parameters of
associated organs at risk for patients with primary brain
tumours or prostate cancer receiving proton therapy. A
reduction of NTCP could be predicted for proton therapy
based on comparative treatment planning. After
validation, these results may be used to identify patients
who are likely to benefit most from proton therapy, as
suggested by the model-based approach [1].
[1] Langendijk JA et al. (2013) Radiother Oncol 107, 267 -
273.
EP-1596 Developing and validating a survival prediction
model for NSCLC patients using distributed learning
A. Jochems
1
, T. Deist
1
, I. El-Naqa
2
, M. Kessler
2
, C. Mayo
2
,
J. Reeves
2
, S. Jolly
2
, M. Matuszak
2
, R. Ten Haken
2
, J. Van
Soes
1
, C. Oberije
1
, C. Faivre-Finn
3
, G. Price
3
, P. Lambin
1
,
A. Dekker
1
1
MAASTRO Clinic, Radiotherapy, Maastricht, The
Netherlands
2
University of Michigan, Radiation oncology, Ann-Arbor,
USA
3
The University of Manchester, Manchester Academic
Health Science Centre, Manchester, United Kingdom
Purpose or Objective
The golden standard for survival prediction in NSCLC
patients, the TNM stage system, is of limited quality for
patients receiving (chemo)radiotherapy[1]. In this work,
we develop an up-to-date predictive model for survival
prediction based on a large volume of patients using a big
data distributed learning approach. Distributed learning is
defined as learning from multiple patient datasets without
these data leaving their respective hospitals.
Furthermore, we compare performance of our model to a
TNM stage based model. We demonstrate that the TNM
stage system performs poorly on the validation cohorts,
whereas our model performs significantly above the
chance level.
Material and Methods
Clinical data from 1299 lung cancer patients, treated with
curative intent with chemoradiation (CRT) or radiotherapy
(RT) alone were collected and stored in 3 different cancer
institutes. Two-year post-treatment survival was chosen
as the endpoint. Data from two institutes (1152 patients
at Institute 1 and 147 at Institute 2) was used to develop
the model while data from the 3
rd
institute (207 patients
at Institute 3) was used for model validation.
A Bayesian network model using clinical and dosimetric
variables was adapted for distributed learning (watch the
animation: link censored). The Institute 1 cohort data is
publicly available at (link censored) and the developed
models can be found at (link censored).
Results
A Bayesian network (BN) structure was determined based
on expert advice and can be observed in figure 1. Variables
included in the final model were TNM stage, age,