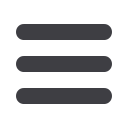

INFORMS Nashville – 2016
233
3 - Productivity Assessment Through Knowledge Generation In
Automotive Manufacturing Sector, Utilizing Statistical Methods
Amir Abolhassani, West Virginia University, 1233 Pineview Drive,
Apt 14, Morgantown, WV, 26505, United States,
Amirabolhassani@gmail.com, Bhaskaran Gopalakrishnan
The research investigates current strategies that help automobile manufacturers to
enhance their productivity. The study utilizes statistical methods to define the
most important effective factors on the most well-known productivity
measurement, Hours per Vehicle (HPV), in the automotive industry in North
American manufacturing plants.
4 - A Machine Learning Approach For Developing Intersection Safety
Performance Function
Hamidreza Ahady Dolatsara, Auburn University, Suite 3301,
345 West Magnolia Ave., Auburn, AL, 36849, United States,
hamid@auburn.edu, Fadel Mounir Megahed
This study introduces a non-subjective method for identifying intersection related
crashes based on their distance to the center of intersection. Then utilizes data of
those crashes for developing Safety Performance Functions (SPFs). This study
utilizes machine learning algorithm to investigate histogram of crashes’ distance
to the center of intersections. The histogram classified to intersection and non-
intersection related parts. After classifying the crashes, intersection SPFs are
developed.
TA02
101B-MCC
Predictive Modeling for Wind Power
Sponsored: Data Mining
Sponsored Session
Chair: Seth Guikema, University of Michigan, 1205 Beal Ave.,
Ann Arbor, MI, 48109-2117, United States,
sguikema@umich.edu1 - Adverse Event Prediction: Forecasting Wind Power Ramps
Andrea Staid, Sandia National Labs, Albuquerque, NM,
United States,
astaid@sandia.govWind power ramp events (large changes in output over a short period of time) are
of particular concern in power systems with high wind penetration. They are also
often difficult to predict. We present statistical methods for combining multi-
source data to better predict the adverse ramp events that are typically not
captured in a standard weather forecast. We present a case study using data from
the Bonneville Power Administration and focus on farm-specific ramps.
2 - Predicting Low-wind Events To Inform Planning And
Policy Incentives
Kristen R. Schell, University of Michigan, Ann Arbor, MI,
United States,
krschell@umich.edu, Seth Guikema
Wind power is currently the most cost-effective source of renewable energy, from
the perspective of life-cycle cost estimates. Given major government backing to
support the general expansion of renewable energy, wind power continues to be
the investment of choice for developers, largely due to its generally higher
resource potential and capacity factors. Hence, as wind power becomes
increasingly integrated into the electric grid, the power system in turn becomes
increasingly vulnerable to “wind droughts”, or periods of low-to-no wind power.
This study uses large-scale wind data to predict low-wind events, to better inform
system planning and renewable policy incentives.
3 - Learning Of Imbalanced Data For Predicting The Power Outage
Elnaz Kabir, PhD Student, University of Michigan,
1205 Beal Avenue, Ann Arbor, MI, 48109-2117, United States,
ekabir@umich.edu, Seth Guikema
In this article we want to model the number of power outages during the
hurricanes. Making accurate prediction of power outage can be really valuable for
the utility companies as well as customers and public agencies to make better
response planning. Our data-set is highly zero inflated and imbalanced because
power outages occur rarely. Since no techniques for dealing with imbalanced data
sets are consistently better for all conditions, we investigate several methods to
find the most appropriate strategy for our data set.
TA03
101C-MCC
Entertainment Analytics
Invited: Entertainment Analytics
Invited Session
Chair: Christian Peukert, University of Zurich, Rämistrasse 71, Zurich,
8006, Switzerland,
christian.peukert@uzh.ch1 - Freemium Pricing: A Stylized Framework And Evidence From
A Large-scale Field Experiment
Jörg Claussen, LMU Munich, Munich, Germany,
j.claussen@lmu.de, Jörg Claussen, Copenhagen Business School,
Frederiksberg, Denmark,
j.claussen@lmu.de, Julian Runge,
Julian Runge, Stefan Wagner
Marketers struggle to find optimal designs for their freemium offerings. We
present a stylized framework of freemium pricing that systematizes key choice
variables and identifies their interaction with relevant outcome variables. Firms
set the share of free product features and the price of premium content and
implement viral mechanisms. These choices affect monetization not only via their
effect on users’ conversion to paying customers, but also via their effects on usage
behavior and viral activities. We apply our framework to a large-scale field
experiment and show that a reduction of free product features increases
conversion rates and viral activities, and has ambivalent effects on usage.
2 - Building An Online Reputation With Free Content: Evidence From
The E-book Market
Dainis Zegners, LMU Munich,
d.zegners@lmu.deAn important strategy to build a reputation is to offer products for free to induce
buyers to provide feedback. I argue that giving away free products to build a
reputation can be a double-edged strategy. It does not only attract buyers with a
high preference, but also buyers with a low preference, who give worse feedback.
I test the strength of this negative effect on reputation using data from an online
platform where I observe self-published authors either selling their e-books at a
positive price or giving them away as free content. Consistent with a negative
selection effect on reputation, I observe that buyers who receive an e-book as free
content rate it worse than buyers who buy it at a positive price.
TA04
101D-MCC
Advances in Using Market Models for Power
Transmission Planning
Sponsored: Energy, Natural Res & the Environment,
Energy I Electricity
Sponsored Session
Chair: David Pozo, Pontificia Universidad Catolica, Avendia Vicuna
Mackenna #4860, Puebla del Principe, 7820436, Chile,
davidpozocamara@gmail.com1 - Non-cooperative Multi-regional Transmission Planning
Saamrat Kasina, Johns Hopkins University, Ames 214,
Baltimore, MD, 21218, United States,
bkasina1@jhu.edu,Benjamin Field Hobbs
Traditional transmission planning methods overlook the political boundaries
within which planning entities operate. However, in reality, there are multiple
regional transmission planning agencies with limited coordination with each
other during planning. We develop a bi-level model that defines the relationship
between multiple non-cooperating transmission planners, generation investors,
and the energy market equilibrium. We ask how the transmission plans from
such a process differ from those from a cooperative planning process and what
the value of cooperation is, if any.
2 - Bi-level Network Planning Model Considering Generation-market
Equilibria Subject To Inefficient Network Pricing
Pengcheng Ding, Johns Hopkins University, Baltimore, MD,
United States,
pangchingting@gmail.com, Benjamin Hobbs
We consider proactive planning of transmission subject to the response of a
generation market with imperfect long-run transmission pricing based on a MW-
km charging system, similar to that of the UK and elsewhere. MW-km-based
charging distorts siting decisions of both thermal and renewable plants relative to
the social optimum because (1) congestion and locations of needed
reinforcements are ignored and (2) all generation types at a location pay the same
charge, no matter how they use the grid.
TA04