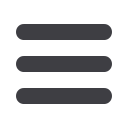

INFORMS Nashville – 2016
234
3 - Reliable Renewable Generation And Transmission Expansion
Planning: Co-optimizing System’s Resources For Meeting
Renewable Targets
David Pozo, Pontificia Universidad Católica de Chile, Santiago,
Chile,
davidpozocamara@gmail.com,Alexandre Moreira,
Alexandre Street, Enzo E Sauma
We propose a two-stage renewable generation and transmission expansion
planning model that jointly finds the best subset of new transmission assets and
renewable sites to be developed. The main goal of this co-optimization planning
model is to address renewable targets while accounting for the least-cost reserve
scheduling to ensure reserve deliverability under generation and transmission
outages and renewable variability. A case study with realistic data from the
Chilean system is presented and solutions obtained with different level of security
are tested against a set of 10,000 simulated scenarios of renewable injections and
system component outages.
TA05
101E-MCC
Short-term Operation, Maintenance, and Long-term
Planning for Power Systems
Sponsored: Energy, Natural Res & the Environment, Energy I
Electricity
Sponsored Session
Chair: Murat Yildirim, Georgia Institute of Technology, 755 Ferst Dr,
Atlanta, GA, 30312, United States,
murat.v.yildirim@gmail.com1 - Topics On Optimal Power Flow
Richard O’Neill, FERC, Richard.O
’Neill@ferc.gov2 - Load-dependent Sensor-driven Maintenance And Operations In
Power Systems
Murat Yildirim, Georgia Institute of Technology, Atlanta, GA,
30332, United States,
murat@gatech.edu,Andy Sun,
Nagi Gebraeel
The operational loads on the generating units have a significant impact on how
fast they degrade. For instance, the frequency of start-up and shut-down cycles
can change the lifetime of combined-cycle power plants by an order of
magnitude. In this talk, we use in-situ sensor based signals to provide i) an
accurate load-dependent degradation model for generating units, and ii) a flexible
framework whereby the scheduler gains some control on how fast the generating
units are degrading. The proposed framework achieves significant improvements
in cost and reliability.
3 - Impact Of Short-term Variability And Uncertainty On Long-term
Planning Problems
Henrik Bylling, University of Copenhagen, Universitetsparken 5,
Copenhagen, DK-2100, Denmark,
bylling@math.ku.dk,Salvador Pineda, Trine Krogh Boomsma
Considering a detailed representation of short-term system operations turns long-
term planning problems, such as generation expansion, computationally
intractable. Simplified models reduce the computational burden by focusing on a
particular aspect of the short-term operation. We compare existing simplified
models in terms of i) their ability to capture the impact of both short-term
variability and short-term uncertainty on long-term planning decisions and ii)
their computational complexity. We also propose a new procedure that
outperforms existing ones in these two aspects.
TA06
102A-MCC
Optimization Models in Data Mining
Sponsored: Data Mining
Sponsored Session
Chair: Petros Xanthopoulos, University of Central Florida,
4000 Central Florida Blvd., P.O. BOX 162993, Orlando, FL,
United States,
petrosx@ucf.edu1 - Relaxing Support Vector Machines
Orestis P. Panagopoulos, California State University, Stanislaus,
Turlock, CA, United States,
orepana@gmail.com, Talayeh Razzaghi,
Petros Xanthopoulos, Onur Seref
In this paper, we extend Relaxed Support Vector Machines (RSVM) to perform
regression as well as one-class classification tasks. Our models, Relaxed Support
Vector Regression (RSVR) and One-Class Relaxed Support Vector Machines
(ORSVM) are formulated using both linear and quadratic loss functions and are
solved with sequential minimal optimization. Their performance is measured on
several publicly available datasets and are compared to other state-of-the-art
regression and classification methods.
2 - Online Feature Importance Ranking Based On Sensitivity Analysis
Alaleh Razmjoo, University of Central Florida, Orlando, FL, 32765,
United States,
alaleh.razmjoo@Knights.ucf.edu,
Petros Xanthopoulos
In this paper, we present a fast and efficient incremental online feature ranking
and feature selection. We employ the concept of global sensitivity and rank
features based on their impact on the outcome of classification model. In the
feature selection part, we use a two stage filtering method to first eliminate highly
correlated and redundant features and then eliminating irrelevant features in the
second stage. It can be implemented along with any online classification method.
The proposed method is primarily developed for online tasks, however, significant
experimental results in comparison with popular feature selection methods
suggest that it can be also used in batch learning tasks.
3 - A Novel Weighting Policy For Unsupervised Ensemble Learning
Based On Mean-variance Portfolio Optimization Method.
Ramazan Unlu, UCF,
ramazanunlu@gmail.comUnsupervised ensemble learning is an optimal combination strategy of individual
clustering methods to create a model that fits to data better. Determining proper
weights for clustering methods is a crucial step to build a well-combined partition.
Recently, an approach was proposed based on concept of internal validity
measures that has profound advantages over traditional ensemble learning.
Despite its robust properties this approach consider only index values itself, but
not variation of them. In this paper, we propose a better weighting policy for this
problem that is based on mean-variance portfolio optimization method and
compare against other popular approaches.
4 - Nonlinear Dimensionality Reduction For Analysis
Ofelectroencephalography Records
Anton Kocheturov, University of Florida, Gainesville, FL,
United States,
antrubler@gmail.comWe suggest using nonlinear dimensionality reduction technique called the Local
Linear Embedding for analysis of EEG records. This approach enabled us to
distinguish between different states of the brain in a more efficient way
comparing to the existing machine learning techniques since it is faster and
doesn’t require training of the algorithm. We also detected evidence for local
linearity of the brain in the resting state and introduced a new model of the brain
based on it.
TA07
102B-MCC
Retail Analytics
Sponsored: Data Mining
Sponsored Session
Chair: Matthew Lanham, Virginia Tech, Pamplin 1007,
Blacksburg, VA, 24061, United States,
lanham@vt.edu1 - Analytics on The Edge Of Retail
Aaron Burciaga, Accenture,
adburciaga@gmail.comThe fervor of big data and business analytics have led to a bumper crop of
education, training, tools, and methods. It’s has become increasingly difficult to
detect the signal from the noise of those same people, processes, and tools that
purportedly exist to distinguish signals from noise. This presentation will review
several case studies of how commercial and national government programs are
developing (or stumbling) in their analytics programs. Emergent technologies and
methods, including the application of Machine Learning and Artificial Intelligence
on edge devices will be presented, showing how the last mile and last dollar can
be closed in both new and traditional challenges.
2 - An Investigation Of Cluster Analysis Of Retail Stores To Improve
Predictive Modeling Of Sales
Linda Schumacher, Merchandise Scientist, Raleigh, NC, 27604,
United States,
schumachers@bellsouth.netData mining clustering algorithms are used to identify similar groups of retail
stores for segmenting data to improve predictive modeling results. Clustering
methods including centroid-based, hierarchical, two-step and probabilistic
clustering are considered. The performance of these clustering methods is
evaluated and compared with calculated metrics. Using data from a national
retailer, the impact of segmenting the data to improve overall predictive
performance is reported.
3 - Investigating Sparse Demand Models To Support The Assortment
Planning Decision
Matthew Lanham, Clinical Assistant Professor, Purdue University,
West Lafayette, IN, United States,
malanham@gmail.comWe present research examining the performance of substitution-based multi-
classification models currently being researched and employed in practice by
major retailers, versus more naïve binary classification models to understand
purchase propensity. We discuss how these models would yield different
assortments for sparse demand products.
TA05