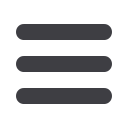

INFORMS Nashville – 2016
248
TA43
208A-MCC
Data-Driven Decision Making
Sponsored: Decision Analysis
Sponsored Session
Chair: Hiba Baroud, Assistant Professor, Vanderbilt University, 2301
Vanderbilt Place, PMB# 351831, Nashville, TN, 37212, United States,
hiba.baroud@vanderbilt.edu1 - Combining Data And Weakly Informative Priors To Make Better
Decisions Faster
Adam Jason Fleischhacker, University of Delaware,
ajf@udel.eduIn tackling decision problems, a decision maker must choose how to represent
uncertainty using techniques that may be classified on a spectrum; on one end
you have fully specified distributions which make strong assumptions, and on the
other end, completely non-parametric and robust approaches which minimize
assumptions. In this work, we develop and use an analytically tractable model of
uncertainty that can model mild assumptions and which can more rapidly extract
value from data than non-parametric approaches.
2 - Projection Of Drought Risk For Thermoelectric Power Plants
Using Downscaled Climate Scenarios
Royce Francis, Assistant Professor, George Washington University,
Washington, DC, 20052, United States,
seed@email.gwu.eduMany climate researchers have studied a number of climate forcing scenarios to
determine how the coupled oceanic-atmospheric systems will respond. At the
same time, these responses will be part of a complex feedback loop with
infrastructure systems. Thus, it is important to help infrastructure decision makers
incorporate climate scenarios into risk and reliability assessments. This
presentation demonstrates a Copula Bayesian Network for projecting
thermoelectric power plant drought risk over CMIP5 downscaled climate
scenarios.
3 - Data-driven Decision Analysis Model For Planning And
Management Of Multiple Purpose Reservoir Cascade Systems
Thushara De Silva, Vanderbilt University, 400 24th Avenue South,
267 Jacobs Hall, Nashville, TN, 37212, United States,
thushara.k.de.silva@vanderbilt.edu, George Hornberger,
Hiba Baroud
The objective of this study is to develop a decision analysis model for the planning
and management of water resources that maximizes multiple objectives such as
economic viability, environmental sustainability, and social development. The
model is deployed to the Mahaweli water resources development which is the
largest multipurpose project of Sri Lanka. A multicriteria decision analysis model
is considered and several data sources are used to assess the multiple attributes in
the model. The utility function incorporates the preferences of multiple decision
makers to assess the weights on the attributes.
4 - Using Data In Decision Making: Big Data, Little Data, No Data
Hiba Baroud, Vanderbilt University,
hiba.baroud@vanderbilt.eduThe role of data analytics in decision making has evolved as the volume of data
changed and the tools and technologies to handle such data improved. Are
decision makers overwhelmed with data or do they still lack the amount of data
they need to improve their decision models? This work is a review of the current
state of the art of the use of data-driven tools in decision analysis techniques in
practice and theory. The objective is to identify gaps between data and decisions
while highlighting opportunities and challenges in research.
TA44
208B-MCC
Investment Analysis and Financial Applications
Sponsored: Decision Analysis
Sponsored Session
Chair: Manel Baucells, University of Virginia Darden School of
Business, 100 Darden Blvd, Charlottesville, VA, 22903, United States,
baucellsm@darden.virginia.edu1 - Net Present Value Analysis And Individual Utility
Manel Baucells, University of Virginia Darden School of Business,
baucellsm@darden.virginia.edu,Sam Bodily
Standard investment analysis employs expected Net Present Value discounting at
a risk-adjusted market return. Such prescription takes the viewpoint of the capital
market, but neglects the risk aversion of the project owner or the individual
investor. We develop an approach that is consistent with expected utility, and
requires the integration of project and market returns. The approach recommends
the use of the certainty equivalent discount rate, which depends on both the
market and the risk aversion of the individual. We explore conditions in which
market returns can be omitted from the analysis; or in which our approach
particularizes into the standard analysis.
2 - An Expected Utility Approach For The Mean-variance
Portfolio Problem
Felipe Macedo de Morais Pinto, Universidade Federal de
Pernambuco, Caixa Postal 7471, Recife, 50630971, Brazil,
felipe_mmp94@hotmail.com, Adiel T de Almeida Filho
This paper presents an expected utility approach for decision makers with
exponential utility behavior as an alternative to the mean-variance approach
when considering a financial portfolio. The DA framework is used for modeling
the classical Markowitz’s portfolio decision problem incorporating a Bayesian
perspective, which allows to include aspects such as the evaluation of
macroeconomic environment and minimizing the Bayes Risk. A numerical
application is presented based on financial data for an investment decision
evaluating a portfolio of DOW 30, FTSE 100 and NASDAQ 100.
3 - A Bayesian Approach For Consumer Credit Debt
Collections Process
Adiel T de Almeida Filho, Assistant Professor, Universidade Federal
de Pernambuco, Caixa Postal 7471, Recife, 50630971, Brazil,
adieltaf@cdsid.org.br, Mee Chi So, Christophe Mues,
Lyn C Thomas
After a borrower defaults on their repayment obligations, collectors of unsecured
consumer credit debt have a number of actions they can take to secure some
repayment of the debt. The operations management challenge in this setting is to
decide which of these actions to take, how long to take them, and in what
sequence to take them. In this paper, a Bayesian Markov Decision Process (MDP)
model is used to find an optimal policy of what action to undertake in the next
period given the current information on the individual debtor’s repayment
performance thus far.
4 - An Analytic Method For Investment Analysis In
Mulichannel Retailing
Somayeh Yasamin Salmani, Drexel University, 2007 Chestnut
Street, Apt D2, Philadelphia, PA, 19103, United States,
ss3858@drexel.edu, Fariborz Partovi
We propose a two-stage stylized model to help firms in making a major strategic
decision in distribution channels investment. Our study is motivated by firms that
provide multiple channels for customers. We develop an analytic model using
customer input and operating costs for specific channel structures to find optimal
investing allocation across different distribution channels.
TA45
209A-MCC
Efficient Learning in Stochastic Optimization
Sponsored: Simulation
Sponsored Session
Chair: Ilya O. Ryzhov, University of Maryland, 4322 Van Munching
Hall, College Park, MD, 20742-1815, United States,
iryzhov@rhsmith.umd.edu1 - Continuous Learning For Contextual Bandits With
Nonstationary Rewards
John G Turner, University of California Irvine, Irvine, CA, United
States,
john.turner@uci.edu, Amelia C Regan, Tianbing Xu,
Yaming Yu
We study how best to match ads to viewers using high-dimensional contextual
features (demographic, browsing behavior) to predict click-through probability.
Using Thompson Sampling in a Bayesian framework, our model learns the
importance of contextual features while adapting/forgetting over time, capturing
changing individuals’ tastes and shifts in the viewing population’s composition.
2 - Bayesian Bandits For Sequential Clinical Trials Of
Multiple Technologies
Ozge Yapar, University of Pennsylvania, Philadelphia, PA, United
States,
yapar@wharton.upenn.edu, Stephen E Chick, Noah Gans
We extend recent work on fully sequential trials for health technologies that
explore the potential benefits of linking Phase III trials with health technology
assessments for market access. We take a bandit perspective that uses Bayesian
learning about multiple health technologies.
TA43