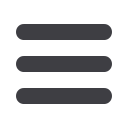

INFORMS Nashville – 2016
249
3 - Vssa – A Variable Sample-size Stochastic Approximation
Schemes For Stochastic Convex Optimization
Uday V. Shanbhag, Pennsylvania State University,
udaybag@engr.psu.edu, Afrooz Jalilzadeh, Jose Blanchet,
Peter W Glynn
Traditional stochastic approximation (SA) schemes employ a single gradient or a
fixed batch of noisy gradients in computing a new iterate. We consider SA
schemes in which Nk samples are utilized at step k and the total simulation
budget is M. This paper derives error bounds in this budget-constrained regime in
both strongly convex and convex regimes with constant and increasing sample-
sizes. Notably, trade-offs between sample-complexity and computational
complexity are examined. Preliminary numerics suggest that such avenues
provide approximate solutions in less than a hundredth of the time taken by
standard SA schemes with modest drops in accuracy.
4 - A New Consistency Theory For Approximate Bayesian Inference
Ye Chen, University of Maryland,
yechen@math.umd.edu,Ilya O Ryzhov
Approximate Bayesian inference is a powerful methodology for constructing
statistical learning mechanisms in problems where incomplete information is
collected sequentially. Approximate Bayesian models have been widely applied,
but the convergence or consistency results for approximate Bayesian estimators
are largely unavailable. We develop a new consistency theory for these learning
schemes by interpreting them as stochastic approximation (SA) algorithms with
additional “bias” terms. We prove the convergence of a general SA algorithm of
this type, and through this, for the first time, show the consistency of several
approximate Bayesian methods from the recent literature.
TA46
209B-MCC
Dynamic Games and Applications to Revenue
Management
Sponsored: Revenue Management & Pricing
Sponsored Session
Chair: Konstantinos Bimpikis, Stanford, Stanford, Palo Alto, CA, 94305,
United States,
kostasb@stanford.edu1 - Dynamic Selling Mechanisms For Product Differentiation
And Learning
N. Bora Keskin, Duke University, Durham, NC, United States,
bora.keskin@duke.edu,John R Birge
We consider a firm that designs a menu of vertically differentiated products for a
population of customers with heterogeneous quality sensitivities. The firm faces
an uncertainty about production costs. We characterize the structure of the firm’s
optimal dynamic learning policy and construct simple and practically
implementable policies that are near-optimal.
2 - When Fixed Price Meets Priority Auctions: Service Systems With
Dual Modes
Krishnamurthy Iyer, Cornell University,
kriyer@cornell.edu,
Jiayang Gao, Huseyin Topaloglu
We consider a service system where service is offered via two modes. The first
mode charges a fixed price, and the service discipline is FIFO. In the second mode,
called the bid-based priority mode, customers submit a bid, obtain service in the
descending order of their bids, and make payments equal to their bids. We
assume the customers have heterogeneous waiting costs, and choose the service
mode strategically on arrival. We establish the existence and uniqueness of a
symmetric equilibrium, which has a simple threshold structure: customers with
either high or low waiting cost obtain service from the bid-based priority mode,
whereas those with moderate waiting cost obtain service from the FIFO mode.
3 - Customizing Marketing Decisions Using Field Experiments
Spyros Zoumpoulis, INSEAD,
spyros.zoumpoulis@insead.edu,
Theodoros Evgeniou, Duncan I Simester, Artem Timoshenko
We investigate how firms can use the results of field experiments to optimize
marketing decisions, and in particular allocating different promotional offers to
different customer segments for the customer acquisition problem of a large
retailer. In the first stand of the work, we solve the problem of finding the optimal
one-shot promotion policy: what promotional offer should be sent to what
customer segment? In the second strand of the work, we solve the problem of
optimally retargeting nonrespondents through promotions in multiple waves:
what customer segment should we stop mailing to, and for what segment would
we benefit from repeated promotions?
TA47
209C-MCC
Personalized E-commerce
Sponsored: Revenue Management & Pricing
Sponsored Session
Chair: Van Anh Truong, Cornell University, Ithaca, NY, United States,
vat3@cornell.edu1 - Distribution-free Pricing
Ming Hu, University of Toronto, Toronto, ON, Canada,
Ming.Hu@rotman.utoronto.ca, Hongqiao Chen
We study a monopoly robust pricing problem in which the seller does not know
the customers’ valuation distribution but knows its mean and variance. Such
minimum requirement of information is nothing but asking two questions: How
much your targeted customers are going to pay on average? And how sure are
you? We obtain the best robust price heuristic in closed form and provide its
distribution-free, worst-case performance bound. We then provide easily
verifiable distribution-free sufficient conditions to guarantee the pure bundle to
be more profitable than separately sales. We illustrate the benefit of bundling by a
couple of practical examples such as subscription services of digital music.
2 - Revenue Management With Consumer Search Cost
Zizhuo Wang, University of Minnesota, Minneapolis, MN,
United States,
zwang@umn.edu, Yan Liu, William L Cooper
We consider a pricing problem in which the product valuations are uncertain to
the consumers. The consumers can find the valuation of the product by incurring
a search cost. We study the seller’s problems of whether it should lower the
search costs of the products, and what prices it should charge. We find that when
there are two products, lowering the search cost of one product while
maintaining a high search cost for the other product may be optimal. We also
show how our results vary depending on the correlation between the uncertainty
of the products.
3 - Approximation Algorithms For Product Framing And Pricing
Anran Li, Columbia University,
al2942@columbia.eduWe propose one of the first models of “product framing” and pricing. Product
framing refers to the way consumer choice is influenced by how the products are
displayed. We present a model where consumers consider only products in a
random number of top web pages. Consumers select a product from these pages
following a general choice model. We show that the product framing problem is
NP-hard. We derive algorithms with guaranteed performance relative to an
optimal algorithm under reasonable assumptions. We also present structural
results for pricing under framing effects. At optimality, products are sorted in
descending order of quality, and prices are shown to be page dependent.
TA48
210-MCC
Social Media Analysis II
Invited: Social Media Analytics
Invited Session
Chair: Yen-Yao Wang, Michigan State University, 5211 Madison
Avenue, Apartment A5, Okemos, MI, 48864, United States,
wangyen@broad.msu.edu1 - A Unified Framework For Credit Evaluation For Internet
Finance Companies
Meheli Basu, Graduate Research Assistant, University of
Pittsburgh, 5820 Elwood Street, APT 33, Pittsburgh, PA, 15232,
United States,
meb209@pitt.eduWe developed and detailed a multi-criteria decision-making framework based on
interface of the subjective approach of analytical hierarchical process (AHP) and
validated by comparative analysis using the objective approach of data
envelopment analysis (DEA) to evaluate credit index. Our framework identifies
and weighs the most important characteristics of SMEs and start-ups which
contribute to overall credit rating. Although our target implementation group is
the internet finance industry, our framework for credit evaluation will also give
start-ups and SMEs an insight into favorable criteria for a good credit standing.
TA48