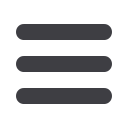

INFORMS Nashville – 2016
313
3 - Evaluating The Impact Of Adopting 3d Printing Services
On The Retailers
Sharareh Rajaei dehkordi, PhD Student of industrial engineering,
New Jersey Institute of Technology, 10 Hill Street, Apt 2N,
Newark, NJ, 07102, United States,
sr552@njit.edu,
Wenbo (Selina) Cai
As 3D printing technology becomes more agile to react to customers’ demands,
one important question for the retailers is whether they should provide 3D
printing services in their brick-and-mortar store in addition to the traditional off-
the-shelf product? If so, what is the pricing scheme that achieves the optimal
profit? What is the optimal capacity of the 3D printers? In this study we answer
these questions by examining retailer’s optimal joint decisions on pricing scheme
and capacity while considering consumers preferences for self-designed, 3D
printed products versus off-the-shelf products, using queueing systems and
stochastic optimization models.
4 - Choice-based Revenue Management Under Online Reviews
Dirk Daniel Sierag, CWI, Science Park 123, Amsterdam,
1098 XG, Netherlands,
dirk@cwi.nlThis article proposes a choice-based network revenue management model that
integrates the effect of reviews. Application areas include airlines, hotels, and
rental cars. The dependency between reviews and revenue is two-fold: the
content of a review depends on the product the customer purchases, and reviews
impact the demand. A complicating factor in this model is that the effects of
reviews are delayed, i.e., by sacrificing revenue now in order to get better
reviews, long-term revenue can be increased. Novel solution methods are
proposed that exploit the presence of reviews in order to optimise revenue.
5 - Customer’S Strategic Behavior Using Thompson Sampling
Sareh Nabi Abdolyousefi, University of Washington, 2727 NE 55th
Street, Seattle, WA, 98105, United States,
snabi@uw.eduRetailer is pricing dynamically in order to maximize his cumulative expected
revenue in a multi-armed bandit setting. Retailer has no information regarding
expected demand and type of customers he is facing, myopic or strategic. He is
applying a machine learning technique, updated Thompson Sampling, to learn
expected demand and customer’s type in an exploration vs exploitation fashion.
We have proved analytically that retailer’s long run price is lower for strategic
customers compared to myopic ones. We have also shown numerically that
retailer can be better off with strategic customers.
TC33
203B-MCC
Queueing Models II
Contributed Session
Chair: Iqra Ejaz, Texas A&M University, College Station, TX,
United States,
iejaz@tamu.edu1 - Multiple Server Preemptive Scheduling With Impatience
Yang Cao, University of Southern California, Los Angeles, CA,
90089, United States,
cao573@usc.eduWe study a scheduling problem with n impatient customers to be served by m
parallel servers (n>m). We assume that the impatience time of customers in
queue and the service time on servers are all exponentially distributed, and the
system earns a positive reward upon each service completion. We consider both
the case of non-preemptive servers and the case of preemptive servers. The
objective is to maximize the expected total return for both cases. We give
conditions under which a list policy is optimal.
2 - Optimal Control Of General Dynamic Matching System
Mohammadreza Nazari, PhD Student, Lehigh University, Lehigh
University, Murray H Goodman Campus, Bethlehem, PA, 18015,
United States,
mon314@lehigh.edu, Aleksandr Stolyar
Consider a system with random arrivals of items of multiple types. There is a
finite number of possible matchings, each being a subset of item types. Each
matching has associated fixed reward, and matched items leave the system. We
propose a matching algorithm and prove its asymptotic optimality in the sense of
maximizing the long-term average reward, while keeping the item queues stable.
This algorithm applies an extended version of the greedy primal-dual (GPD)
algorithm to a virtual system, which allows negative item queues.
3 - Acuity-based Nurse-staffing Strategies For Inpatient Settings
Using A Stochastic Modeling Approach
Parisa Eimanzadeh, PhD Student, Wichita State University, 6000 E
Mainsgate Street, Apt 108, Wichita, KS, 67220, United States,
pxeimanzadeh@wichita.edu, Ehsan Salari
Minimum nurse-to-patient ratios have been traditionally used to guide staffing
decisions in inpatient units. However, the severity of nursing care may vary across
inpatients, rendering those ratios ineffective. We develop a stochastic modeling
framework to quantify the impact of different staff levels on the performance of
inpatient units while accounting for heterogeneity in patient acuity and staff
nursing skill levels.
TC34
4 - Condition-based Maintenance For Queues With
Degrading Servers
Iqra Ejaz, Texas A&M University, College Station, TX, United
States,
iejaz@tamu.edu, Michelle M. Alvarado, Nagi Gebraeel,
Natarajan Gautam, Mark Alan Lawley
We derive an analytical model for condition monitoring of a single server queue
with Markovian degradation, Poisson arrivals, and general service and repair
times. Stability conditions and performance measures (e.g., average queue length,
average degradation.) are derived through steady state analysis. An optimal repair
decision model is presented that minimizes an objective function with four costs:
repair, catastrophic failure, quality and holding. We develop and verify a
simulation model, perform a sensitivity analysis, and show insights learned from
relaxing underlying assumptions.
TC34
204-MCC
Public Policy and Healthcare Operations
Sponsored: Manufacturing & Service Oper Mgmt, Healthcare
Operations
Sponsored Session
Chair: Susan F Lu, Purdue University, West Lafayette, IN,
United States,
lu428@purdue.eduCo-Chair: Lauren Xiaoyuan Lu, University of North Carolina at Chapel
Hill, Chapel Hill, NC, United States,
lauren_lu@unc.edu1 - Do Mandatory Overtime Laws Improve Quality? Staffing Decisions
And Operational Flexibility Of Nursing Homes
Lauren Xiaoyuan Lu, University of North Carolina at Chapel Hill,
Chapel Hill, NC, United States,
lauren_lu@unc.edu, Susan F Lu
During the 2000s, over a dozen U.S. states passed laws that prohibit health care
employers from mandating overtime for nurses. Using a nationwide panel dataset
from 2004 to 2012, we find that these mandatory overtime laws reduced the
service quality of nursing homes, as measured by an increase in deficiency
citations. This outcome can be explained by two undesirable changes in the
staffing hours of registered nurses: decreased hours of permanent nurses and
increased hours of contract nurses per resident day.
2 - Predicting Nurse Turnover And Its Impact On Staffing Decisions
Eric Webb, Indiana University, Bloomington, IN, United States,
ermwebb@indiana.edu,Kurt Bretthauer
Nurse turnover remains a significant problem in skilled nursing facilities across
the United States. High turnover leads to two important questions: (1) Hiring
decisions - What applicant attributes should be valued when hiring nurses, in
order to hire nurses that are effective at their jobs and likely to stay for a long
duration? (2) Staffing decisions - How should nurse workload be managed in
order to prevent burnout and decrease turnover? Based on a large dataset from
skilled nursing facilities in the United States, we first use a survival model to
predict nurse turnover. For this talk we then focus on staffing and incorporate
these empirical results into analytical models for nurse staffing decisions.
3 - Hospital Readmissions Reduction Program: An Economic And
Operational Analysis
Dennis Zhang, Washington University in St. Louis, St. Louis, MO,
90024, United States,
zjj1990228@gmail.comThe Hospital Readmissions Reduction Program (HRRP) requires the Centers for
Medicare and Medicaid Services to penalize hospitals with excess readmissions.
We take an economic and operational (patient flow) perspective to analyze the
effectiveness of this policy in encouraging hospitals to reduce readmissions. We
develop a game-theoretic model to show that the competition among hospitals
can be counterproductive: it increases the number of nonincentivized hospitals.
We calibrate our model with a data set of more than 3,000 hospitals and draw
several policy recommendations to improve this policy’s outcome.