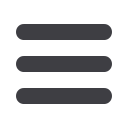

INFORMS Nashville – 2016
318
TC46
209B-MCC
Empirical and Data-Driven Studies in Revenue
Management and Pricing
Sponsored: Revenue Management & Pricing
Sponsored Session
Chair: Jun Li, Ross School of Business, University of Michigan, Ann,
MI, United States,
junwli@umich.eduCo-Chair: Serguei Netessine, INSEAD, Singapore, Singapore,
serguei.netessine@insead.edu1 - Compete With Many: Price Competition In High
Dimensional Space
Jun Li, Ross School of Business, University of Michigan,
junwli@umich.edu, Serguei Netessine, Sergei Koulayev
We study price competition in markets with a large number (in the magnitude of
hundreds or thousands) of potential competitors. We propose a new instrument
variable approach to address simultaneity bias in high dimensional variable
selection problems. The novelty of the idea is to exploit online search and
clickstream data to uncover demand shocks at a granular level, with sufficient
variations both over time and across competitors. We apply this data-driven
approach to study the New York City hotel market.
2 - Randomized Markdowns And Online Monitoring
Ken Moon, The Wharton School, Philadelphia, PA, United States,
kenmoon@wharton.upenn.edu, Kostas Bimpikis, Haim Mendelson
Using data tracking customers of a North American retailer, we present empirical
evidence that monitoring products online associates with successfully obtaining
discounts. We develop a structural model of consumers’ dynamic monitoring to
find substantial heterogeneity, with consumers’ opportunity costs for an online
visit ranging from $2 to $25 in inverse relation to their price elasticities. We show
implications for retail operations and also discuss targeting customers with price
promotions using their online histories.
3 - A Nonparametric Approach To Learning Mixture Models
Srikanth Jagabathula, NYU Stern,
sjagabat@stern.nyu.edu,Lakshminarayanan Subramanian, Ashwin Venkataraman
We consider the problem of learning a mixture model, where the number of
mixture components is learned directly from the data. Our framework applies to
any mixture model, but we specialize our techniques to learn the mixture of
multinomial (MNL) models. We pose the learning problem as that of minimizing
a loss function (likelihood, squared-loss, etc.) over the data and model
parameters. Common formulations result in a non-convex problems. We
overcome this through a novel reformulation that converts the problem into a
semi-infinite convex program. We then apply conditional-gradient techniques to
solve the convex program. We validate our methods theoretically and empirically.
4 - Leveraging Inventory In Profit Maximization For Personalized
Online Bundle Pricing Recommendation
Anna M Papush, Massachusetts Institute of Technology, 1 Amherst
Street, Cambridge, MA, 02139, United States,
apapush@mit.edu,Pavithra Harsha, Georgia Perakis
E-commerce has been vastly growing in popularity over the past decade. It has
been capturing increasing proportions of the retail market. Thus gaining the
competitive edge in this sector is of utmost importance to any firm’s success. This
work presents a model for providing relevant product recommendations at
personalized prices while leveraging knowledge of inventory at-risk for
markdowns and maximizing retailer profits. We demonstrate practical
applications through implementation on actual e-tailer data, as well as
establishing performance guarantees relative to an optimal offline approach.
TC47
209C-MCC
Choice-based Demand and Strategic Consumers
Sponsored: Revenue Management & Pricing
Sponsored Session
Chair: Gustavo Vulcano, New York University, 44 West Fourth St, Suite
8-76, New York, NY, 10012, United States,
gvulcano@stern.nyu.edu1 - Predicting Individual Customer Responses To Product
Promotions
Dmitry Mitrofanov, NYU,
dm3537@stern.nyu.edu,Srikanth
Jagabathula, Gustavo Jose Vulcano
We consider the problem of predicting an individual customer’s response to a
product promotion using historical purchase transactions data, tagged by the
customer id. The problem is challenging because of the limited number of
observations available for each individual. To extract the signal from the limited
data most efficiently, we model each individual through a partial-order consisting
of weak and strong preferences (A is strongly preferred to B if non-promoted A is
purchased over promoted B). We calibrate an MNL model over the partial orders
and quantify its prediction power on out-of-sample transactions. Then, we use
this information to optimize personalized promotions.
2 - The Heteroscedastic Exponomial Choice Model
Aydin Alptekinoglu, Penn State,
aydin@psu.edu,John H Semple
We develop analytical properties of the Heteroscedastic Exponomial Choice (HEC)
model and demonstrate its estimation using a household panel data of grocery
purchases. The HEC model compares quite favorably to MNL in out-of-sample
prediction.
3 - Assortment Optimization With Product Costs And Constraints
Sumit Kunnumkal, ISB,
sumit_kunnumkal@isb.edu,Victor
Martinez de Albeniz
We consider the assortment optimization problem under the MNL model with
product fixed costs and constraints. We propose a new method to obtain an upper
bound on the optimal expected profit. We show that our method is tractable and
has provable performance guarantees for some common types of assortment
constraints.
4 - Optimal Pricing In Continuous Time
José Correa, University of Chile, Santiago, Chile,
correa@uchile.clWe consider continuous time pricing problems with strategicconsumers that
arrive over time. By combining ideas from auctiontheory and recent work on
pricing with strategic consumers we derivethe optimal continuous time pricing
scheme in some situations. Ournovel approach is based on optimal control theory
and is well suitedfor numerical computations.
TC48
210-MCC
Social Media Analysis IV
Invited: Social Media Analytics
Invited Session
Chair: Anandasivam Gopal, University of Maryland, Smith School of
Business, College Park, MD, 11111, United States,
agopal@rhsmith.umd.edu1 - Extraction Of Adverse Events From Social Media
Lina Zhou, University of Maryland-Baltimore County, 1000 Hilltop
Circle, Baltimore, MD, 21250, United States,
zhoul@umbc.edu, Yin
Kang
Adverse events have significant impacts on patients’ safety. Understanding patient
reports of adverse events in social media remains a significant research challenge.
We proposed new methods based on syntactic dependency relations to extract
adverse events. The experiment results demonstrate that our proposed methods
improve the extraction performance across all data sets in terms of both precision
and recall.
2 - When It Rains, It Pours: Effect Of Social Media On Stock Price
Behavior During Firm Crises
Soo Jeong Hong, Michigan State University, 404 Wilson Rd, Room
311, East Lansing, MI, 48824, United States,
hongsoo3@msu.edu,Kwangjin Lee
We examine the effect of social media usage on the capital market under a firm
crisis situation. Focusing on consumer product recalls, we investigate the factors
which determine individuals’ information sharing decisions in social media. We
also analyze when recall information shared via social media can exacerbate
negative market reactions.
3 - Networked Pattern Recognition Frameworks For Understanding
And Detecting Future Terrorism Threats
Salih Tutun, Turkish Military Academy, and Binghamton
University, 4400 Vestal Pkwy E, Binghamton, NY, 13850, United
States,
slh.tutun@gmail.com, Mohammad Khasawneh
The challenge of governments are how to track threats, since terrorists have
learned how to avoid unsecured communications, such as social media. This
research is proposed as a new framework that will better understand the
characteristics of future suicide attacks by analyzing the relationship among the
attacks. It is also proposed as a new unified detection framework that applies
pattern classification techniques to network topology to detect terrorist activity.
The finding results can potentially use to propose reactive strategies thus enabling
precautions to be taken against future attacks.
TC46