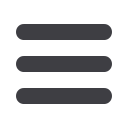

INFORMS Nashville – 2016
315
TC38
206A-MCC
Service Science II
Contributed Session
Chair: Do-Hyeon Ryu, Pohang University of Science and Technology,
Pohang, Kyungbuk, Korea, Republic of,
dhryu@postech.ac.kr1 - Financial Valuation Of Wellness Centered Operations
Min Kyung Lee, Clemson University, 100 Sirrine Hall, Clemson,
SC, 29634, United States,
minl@g.clemson.edu,Aleda Roth,
Rohit Verma, Bernardo F. Quiroga
The importance of individual health and their wellbeing sheds light on the
evolution of building design to achieve human sustainability. This study
contributes the unstudied area of the financial and market impact of hotel guest
rooms with wellness inspired features by comparing the Revenue Per Available
Room (RevPAR) and customer satisfaction score.
2 - Extended Warranty Information Availability Formats: Impact On
Consumer Purchase Decisions
Paul R Messinger, University of Alberta, Faculty of Business, 3-20e
Faculty Of Business Bldg, Edmonton, AB, T6G 2R6, Canada,
paul.messinger@ualberta.ca, Moein Khanlari Larimi
Information about extended service contracts, mainly price, is generally offered to
buyers subsequent to their product purchase decision during the checkout.
However, extended service contract (ESC) information has also been made
available alongside product attribute information. In this research, we ask
whether and how the mere availability of ESC information during the product
choice phase might affect consumers’ product and ESC purchase decisions. To
answer this question, we pit the simultaneous vs. delayed ESC information
availability strategies against one another in choice experiments.
3 - Technology In Service – From Dumb To Thinking To Feeling
Ming-Hui Huang, Distinguished Professor, National Taiwan
University, 1 Sec 4, Roosevelt Road, Taipei, 10617, Taiwan,
huangmh@ntu.edu.twIn this paper a three-generational technology evolution (from automated to
thinking to feeling technology) is presented and the way they can be used in
service is illustrated. Automated technologies are mainly developed for
productivity, which achieves greater output with less input by standardization.
Thinking technologies are designed to handle cognition-based personalization for
customer satisfaction. Feeling technologies are to handle emotion-based
personalization that enriches interactions. Given the multiplicity of technology,
we should explore how to use the right technology for the right purpose in the
right context by the right employees for the right customers.
4 - Development Of An Online To Offline Service Blueprint
Do-Hyeon Ryu, Pohang University of Science and Technology,
Pohang, Kyungbuk, Korea, Republic of,
dhryu@postech.ac.kr,
Chie-Hyeon Lim, Kwang-Jae Kim
The online to offline(O2O) service is to find and attract users online and direct
them to offline stores. Examples include Uber, Zipcar, Groupon, and so on.
Although the term, O2O, is frequently used in academia and industries, research
on systematic methods for developing O2O services has been scarce. This research
aims to develop a new type of service blueprint specially designed for O2O
services. This blueprint is expected to help O2O service providers visualize their
services from the customer perspective.
TC39
207A-MCC
Dynamic Learning Applications
Sponsored: Applied Probability
Sponsored Session
Chair: N. Bora Keskin, Duke University, Durham, Durham, NC, 27708,
United States,
bora.keskin@duke.edu1 - Dynamic Pricing In Unknown Environments With Memory
Abbas Kazerouni, Stanford University, Stanford, CA, 94305,
United States,
abbask@stanford.edu, Benjamin Van Roy
We consider the problem of dynamic pricing in an unknown environment where
the demand at any time is governed by the prices at that time as well as the
previous time steps. The delayed consequences of the prices introduce new
challenges to the problem and require more sophisticated pricing strategies. To
deal with this problem, we propose a pricing strategy based on Reinforcement
Learning techniques and derive bounds on its performance. We show the
efficiency of the proposed strategy by comparing its performance against other
strategies as well as the lower bound through some examples.
2 - Online Active Linear Regression
Carlos Riquelme, Stanford University,
rikel@stanford.edu,
Ramesh Johari, Baosen Zhang
We study the problem of online active learning to collect data for regression
modeling; a decision maker that faces a limited experimentation budget but must
efficiently learn an underlying linear model. Our main contribution is a novel
threshold-based algorithm for selection of most informative observations; we
characterize its performance and fundamental lower bounds. We extend the
algorithm and its guarantees to sparse linear regression in high-dimensional
settings. Simulations show significant benefits over random sampling in several
real-world datasets that exhibit high nonlinearity and high dimensionality -
strongly reducing the mean and variance of the squared error.
3 - Learning Preferences With Side-information: Near Optimal
Recovery Of Tensors
Andrew A Li, MIT, Cambridge, MA, United States,
aali@mit.edu,Vivek Farias
Many recent problems of great interest in e-commerce can be cast as large-scale
problems of tensor recovery in three dimensions. Thus motivated, we study the
problem of recovering ‘simple,’ 3D tensors from their noisy observations. We
provide an efficient algorithm to recover structurally simple tensors given noisy
(or missing) observations of their entries; our version of simplicity subsumes low-
rank tensors for various definitions of tensor rank. Our algorithm is practical for
large datasets and provides a significant performance improvement over
incumbent approaches to Tensor completion. Further, we show theoretical
recovery guarantees that under certain assumptions are order optimal.
4 - On Incomplete Learning And Certainty-equivalence Control
N. Bora Keskin, Duke University, Durham, NC, United States,
bora.keskin@duke.edu,Assaf Zeevi
Motivated by dynamic pricing applications, we consider a dynamic control-and-
estimation problem. The decision-maker sequentially chooses controls and
observes responses that depend on both the chosen controls and an unknown
parameter. The decision-maker uses a certainty-equivalence policy, and we
characterize the asymptotic accuracy performance of this policy.
TC40
207B-MCC
Applied Probability and Machine Learning I
Sponsored: Applied Probability
Sponsored Session
Chair: Guy Bresler, Massachusetts Institute of Technology, 32 Vassar St,
32-D672, Cambridge, MA, 02139, United States,
guy@mit.edu1 - Controlling Bias From Data Exploration Using Information Theory
Daniel Russo, Northwestern University, Evanston, IL, United
States,
dan.joseph.russo@gmail.com,James Zou
Modern data is messy and high-dimensional, and it is often not clear a priori
which questions to ask. Instead, the analyst typically needs to use the data to
search for interesting analyses to perform and hypotheses to test. It’s widely
recognized that this process, even if well-intentioned, can lead to biases and false
discoveries, contributing to the reproducibility crisis in science. We propose a
general information-theoretic framework to quantify and provably bound the bias
of an arbitrary adaptive analysis process. We prove that our bound is tight in
natural models, and then use it to give rigorous insights into when common
procedures do or do not lead to substantially biased estimation.
2 - K-nearest Neighbor Methods For Information Estimation
Sewoong Oh, University of Illinois, Urbana, IL, United States,
swoh@illinois.eduEstimators of information theoretic measures such as entropy and mutual
information from samples are a basic workhorse for many downstream
applications in modern data science. State of the art approaches have been either
geometric (nearest neighbor (NN) based) or kernel based. In this paper we
combine both these approaches to design new estimators of entropy and mutual
information. Our estimator uses bandwidth choice of fixed k-NN distances; such a
choice is both data dependent and linearly vanishing in the sample size and
necessitates a bias cancellation term that is universal and independent of the
underlying distribution.
3 - Semidefinite Programming Relaxations For Exact Recovery Of
Hidden Communities
Jiaming Xu, Purdue University, West Lafayette, IN, United States,
xu972@purdue.edu,Bruce Hajek, Yihong Wu
We study a semidefinite programming (SDP) relaxation of the maximum
likelihood for exactly recovering hidden communities under the stochastic block
model. It is shown that when the community size is large comparing to the
network size, the SDP relaxation achieves the information-theoretic recovery
threshold with sharp constants; when the community size is small, the SDP
becomes strictly suboptimal comparing to the maximum likelihood estimator.
TC40