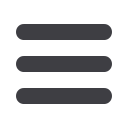

INFORMS Nashville – 2016
356
3 - Facilitate Fit Revelation In a DistributionChannel
Lin Hao, University of Notre Dame, 351 Mendoza College Of
Business, Notre Dame, IN, 46556, United States,
lhao@nd.edu,
Yong Tan
We investigate a retailer’s and a supplier’s incentive to facilitate fit revelation, i.e.,
facilitate consumer learning of their true product fit, under two popular channel
pricing models, agency pricing model and wholesale pricing model.
TD66
Mockingbird 2- Omni
Technometrics Invited Session: Recent Statistical
Methods for Analyzing Big Data
Sponsored: Quality, Statistics and Reliability
Sponsored Session
Chair: Peihua Qiu, Professor and Chair, University of Florida, 2004
Mowry Road, Gainesville, FL, 32611, United States,
pqiu@phhp.ufl.edu1 - Discovering The Nature Of Variation In Nonlinear Profile Data
Daniel Apley, Northwestern University,
apley@northwestern.eduMost prior work on profile data in the quality control literature has focused on
monitoring to detect sudden changes in the characteristics of the profiles, relative
to an in-control sample of profiles. In contrast, we develop an approach for
exploratory analysis of a sample of profiles to discover the nature of any profile-
to-profile variation present over the sample via manifold learning. Instead of
analyzing parameter variation in some prespecified parametric profile model, our
focus is on discovering and visualizing an appropriate characterization or
parameterization of the variation, as a tool to facilitate discovery (and ultimately
elimination) of its root causes.
2 - A Bootstrap Metropolis-hastings Algorithm For Bayesian Analysis
Of Big Data
Faming Liang, University of Florida,
faliang@ufl.eduMCMC methods have proven to be a powerful tool for analyzing data of complex
structures. However, their computer-intensive nature precludes their use for big
data analysis. We propose the bootstrap Metropolis-Hastings (BMH) algorithm,
which provides a general framework for how to tame powerful MCMC methods
to be used for big data analysis; that is to replace the full data log-likelihood by a
Monte Carlo average of the log-likelihoods that are calculated in parallel from
multiple bootstrap samples. The BMH algorithm possesses an embarrassingly
parallel structure and avoids repeated scans of the full dataset in iterations, and is
thus feasible for big data problems.
3 - Online Updating Of Statistical Inference In The Big Data Setting
Elizabeth D. Schifano, University of Connecticut, Storrs, CT,
United States,
elizabeth.schifano@uconn.edu,Ming-Hui Chen,
Chun Wang, Jing Wu, Jun Yan, Yuping Zhang
We present statistical methods for big data arising from online analytical
processing, where data arrive in streams and require fast analysis without
storage/access to historical data. In particular, we develop computationally
efficient, minimally storage-intensive iterative estimating algorithms and
statistical inferences for linear models and estimating equations that update as
new data arrive. We propose goodness of fit tests, a new estimator within the
estimating equation setting, and a modification to incorporate new variables
midway through the data stream. We demonstrate the effectiveness of our
procedures through theoretical and empirical analysis, as well as in application.
TD67
Mockingbird 3- Omni
QTQM Invited Session
Sponsored: Quality, Statistics and Reliability
Sponsored Session
Chair: Jing Li, Arizona State University, Arizona State University,
Tempe, AZ, 85287, United States,
jing.li.8@asu.edu1 - A Mixed-effect Model For Analyzing Experiments With
Multistage Processes
Kaibo Wang, Tsinghua University,
kbwang@tsinghua.edu.cnIn industrial practice, most products are produced by processes that involve
multiple stages. In this paper, through an analysis of the error transmission
mechanism, we propose a mixed-effect model for analyzing experiments with
multistage processes. Based on an analysis of simulated and real experimental
data, we find that different conclusions about factor significance may be drawn if
the data are analyzed differently. In addition, the mixed-effect model can help
separate errors at different stages and hence provide more information on process
improvement.
2 - Setup Adjustment For Asymmetric Cost Functions Under
Unknown Process Parameters
Arda Vanli, Florida State University, 2525 Pottsdamer St,
Tallahassee, FL, FL, 32310, United States,
oavanli@eng.fsu.edu,
Zilong Lian, Enrique Del Castillo”
We present a bayesian approach for the optimal control of a machine that can
experience setup errors assuming an asymmetric off-target cost function. It is
assumed that the setup error cannot be observed directly due to presence of
measurement and part-to-part errors and the variance of this error is not known
a priori. The setup error can be compensated by performing sequential
adjustments of the process mean based on observations of the parts produced. We
show how the proposed method converges to the optimal (known variance)
trajectory, recovering from a possibly biased initial variance estimate. Simulations
results are presented for constant asymmetric and quadratic asymmetric cost
functions.
3 - Bounded Loss Functions And The Characteristic Function
Inversion Method For Computing Expected Loss
Matthias Tan, City University of Hong Kong,
matthtan@cityu.edu.hkIn robust parameter design, the quadratic loss function is commonly used.
However, this loss function is not always realistic. We propose a general class of
bounded loss functions that are cumulative distribution functions and probability
density functions. New loss functions are investigated and they are shown to
behave differently from the quadratic loss. For models linear in the noise factors,
we give a numerical method based on characteristic functions inversion for
computing expected loss. The method is very quick and accurate. It is applicable
as long as the distributions chosen to represent the loss function and variation in
the noise factors have tractable characteristic functions.
4 - Quasi-feedforward And Feedback Control For Random Step Shift
Disturbance Models
Lihui Shi, Senior Data Scientist, Centerfield Corporation, El
Segundo, CA, 90245, United States,
shilihui@uw.eduLihui Shi, Senior Data Scientist, University of Washington,
Seattle, WA, 98195, United States,
shilihui@uw.eduProcess monitoring and process adjustment strategies are two important parts of
the process improvement methods, and they should be integrated together in
stead of separated. Integrated moving average (IMA) model is the most common
disturbance model, and step shift model is one type of more complicated one that
often exists in real applications. We investigate the IMA background disturbance
subject to random step shifts with a certain probability. We propose a feedback
control with a quasi-feedforward control by monitoring the output errors. It is
very robust against parameter misspecifications. We also investigate the type I and
type II errors in process adjustment on this disturbance model.
TD68
Mockingbird 4- Omni
Reliability Modeling and Optimization in Early Product
Design Stages
Sponsored: Quality, Statistics and Reliability
Sponsored Session
Chair: Zhaojun Li, Western New England University, Springfield, MA,
United States,
zhaojun.li@wne.edu1 - Assessing Failure Dependency In A Complex System
Rong Pan, Arizona State University,
Rong.Pan@asu.edu,
Petek Yontay
In this talk we will discuss a Bayesian network model for assessing system
reliability of a complex system. Coupling with Bayesian inference methods , the
posterior distributions of the conditional probabilities in a BN model can be
estimated by combining failure information and expert opinions at both system
and component levels.
2 - A Multi-objective Approach For Multi-stage Reliability
Growth Planning By Considering The Timing Of New
Technologies Introduction
Steven LI, Western New England University,
zhaojun.li@wne.edu,Mohammad Sadegh Mobin, Hossein Cheraghi
This paper proposes a new multi-stage reliability growth planning model which
optimizes and balances the product development cost, time, and the product
reliability. The number of test units, test time, and the percentage of introduced
new technologies are major decision variables. Different reliability growth rates
are considered for each sub-system in each stage. An integrated approach is
developed to optimize the problem, which starts with a multi-objective
evolutionary algorithm to find a set of Pareto optimal solutions followed by the
application of clustering tools to cluster the solutions. The clustered solutions are
further ranked using a multiple criteria decision making tool.
TD66