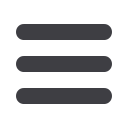

INFORMS Nashville – 2016
359
5 - An Experimental Study Of Customer’s Risky And Egalitarian
Behaviors In a Clearance Sales Problem
Junlin Chen, Associate Professor, Central University of Finance
and Economics, 39 South College Road, Haidian District, Beijing,
100081, China,
chenjunlin@cufe.edu.cn, Yingshuai Zhao
We consider a monopolist set prices in a two-period selling season such that low-
value customers postpone the purchase for a lower price but subject to rationing
risk, whereas high-value customers buy regularly. Traditionally, the optimal
regular price is usually set with making high-value customers indifferent between
buying early and late, and then basically all high-value customers are assumed to
buy regularly. By conducting laboratory experiments, we provide evidence
against this basic assumption. We demonstrate that the behavior of subjects can
be explained by risky and egalitarian behaviors. We also find evidence about
irrational waiting and myopic buying strategic customers.
TD76
Legends D- Omni
Decision Analysis II
Contributed Session
Chair: Nikita Korolko, PhD Candidate, Massachusetts Institute of
Technology, 1 Amherst st, E40-106 ORC, Cambridge, MA, 2139, United
States,
korolko@mit.edu1 - Impact Of Service Payment On Product And Service Supply Chain
Considering Time Value
Jiayuan Liu, Tsinghua University, Tsinghua University, 14 Zijing
Department, Beijing, 100084, China,
liujiayuan46@163.com,Wanshan Zhu
We investigate a supply chain consisting of a service provider (e.g. AT&T) and a
product maker (e.g. Apple), where the payment for service is in installments
through a contract time frame. By modeling the installment payment and time
value and solving the equilibrium strategies of pricing and inventory decisions,
we analyze the impact of the service payment on the structure of the supply
chain.
2 - Almost Stochastic Dominance When Utility Is Action-dependent
Chunling Luo, National University of Singapore, Singapore,
Singapore,
c_luo@u.nus.edu, Chin Hon Tan
Current stochastic dominance rules assume that utility function is identical across
all actions. This assumption makes stochastic dominance rules not applicable
under some practical settings. To help reveal decision makers’ preferences under
these settings, we generalize almost stochastic dominance by allowing utility
functions to differ among actions.
3 - Decision Analysis For Locating Partial Building Renovations
Regarding Adaptive Reuse
Kristopher Harbin, Doctoral Candidate, The University of Alabama,
Tuscaloosa, AL, 35487-0205, United States,
kbharbin@ua.eduWhen considering a building renovation for an adaptive reuse there are
numerous building attributes and systems that should be considered. These
building attributes should be compared to the proposed reuse and any alterations
needed should be noted. The impact of these alterations should be noted and
assigned an appropriate weight reflecting the level of impact. This is done for
multiple areas of the building which helps ensures a complete listing of the
renovation options are seen.
4 - Covariate-adaptive Optimization In Online Clinical Trials
Nikita Korolko, PhD Candidate, Massachusetts Institute of
Technology, 1 Amherst st, E40-106 ORC, Cambridge, MA, 02139,
United States,
korolko@mit.edu, Dimitris Bertsimas,
Alexander M Weinstein
Pharmaceutical companies spend tens of billions of dollars each year to operate
clinical trials needed for the approval of new drugs. We present an online
allocation algorithm for clinical trials that leverages robust mixed-integer
optimization. In simulated experiments involving both single and multiple
controlled covariates, our method reduces the number of subjects needed to
achieve a desired level of statistical power by at least 35% relative to state-of-the-
art allocation algorithms. Correspondingly, we expect that our computationally
tractable approach could significantly reduce both the duration and operating
costs of a clinical trial.
TD77
Legends E- Omni
Opt, Integer Programing IV
Contributed Session
Chair: Joseph B Mazzola, Cleveland State University,
1860 East 18th Street, BU 530, Cleveland, OH, 44115, United States,
j.b.mazzola@csuohio.edu2 - Using Odheuristics To Solve Hard Mixed Integer
Programming Problems
Alkis Vazacopoulos, Optimization Direct, Inc.,
202 Parkway, Harrington Park, NJ, 07640, United States,
alkis@optimizationdirect.com, Robert Ashford
It is not practical to prove optimality for most large scale MIP models. Indeed,
many are so computationally onerous that it is not possible to raise the best
bound at all beyond the root solve. ODHeuristics is a general purpose program
built on CPLEX for obtaining good feasible solutions to such MIPs. It is designed
for scheduling problems but works for any MIP which has a reasonable number
of integer feasible solutions. It has been deployed effectively on packing problems,
supply chain and telecoms as well as scheduling applications. This talk looks at
what ODHeuristics does and how - in general terms - it goes about it with
reference to some simple examples.
3 - Objective Scaling Ensemble Approach For Integer
Linear Programming
Weili Zhang, University of Oklahoma, 202 W. Boyd St., Room 116,
Norman, OK, 73019, United States,
weili.zhang-1@ou.edu,
Charles D. Nicholson
The objective scaling ensemble approach is a novel, approximate solution
procedure for integer linear programming problems\deleted[id=WZ]{in general}
\added[id=WZ]{shown to be effective on a wide variety of ILP problems}. The
technique identifies and aggregates multiple partial solutions to modify the
problem formulation and significantly reduce the search space. An empirical
analysis on widely available difficult problem instances demonstrate the efficacy
of our approach by outperforming the existing advanced solution strategies
implemented in modern optimization software.
4 - Preventive Maintenance And Replacement Scheduling
Farzad Pargar, University of Oulu, Oulu, Finland,
farzad.pargar@oulu.fi,Jaakko Kujala
In this paper, a pure integer linear programming model is developed to determine
the optimal preventive maintenance and replacement schedules for a series of
multi-component systems. In this model, we have considered a finite and
discretized planning horizon in which three possible actions must be planned for
each component in each system, namely maintenance, replacement, or do
nothing. The objective is to minimize the total cost of projects by grouping
maintenance and replacement operations. Because of the complexity of the
model, several heuristic methods are applied to tackle the problem.
5 - Non Monotone Submodular Knapsacks And Applications
Avinash Bhardwaj, Postdoctoral Fellow, Georgia Institute of
Technology, Room 336, 755 Ferst Drive, NW, Atlanta, GA, 30332,
United States,
abhardwaj@gatech.edu, Alper Atamturk
We study the facial structure of the convex hull of the level sets of a given
submodular set function. In particular we derive valid inequalities and their
extensions for the general lower level sets of submodular set functions, and
propose the facet defining conditions for the same. We relax the monotonicity
assumptions on the underlying set function and thus offering a generalization to
earlier studies on this subject matter. We derive the appropriate valid inequalities
and their extensions from the aggregation of the linear knapsack inequalities
corresponding to the extended polymatroid of the set function in context.
1 - Generalizations And Applications Of The Multiperiod
Assigment Problem
Joseph B Mazzola, Professor and Endowed Chair, Cleveland State
University, 1860 East 18th Street, BU 530, Cleveland, OH, 44115,
United States,
j.b.mazzola@csuohio.eduThe Multiperiod Assignment Problem (MultiAP) involves the cost-minimizing
assignment of a set of tasks to a set of agents within each period of a finite
planning horizon when, in addition, there are transition costs associated with
changing agent-task assignments from one period to the next. We review the
literature on MultiAP and consider generalizations of the MultiAP including, for
example, a model in which task learning occurs when an agent is able to work
repeatedly on the same task. We also discuss applications of MultiAP.
TD77