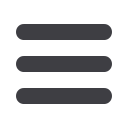

WC42INFORMS Charlotte – 2011
363
Wednesday, 8:00AM - 9:30AM
WA01
101A-MCC
Forecasting
Sponsored: Data Mining
Sponsored Session
Chair: Ivan G Guardiola, Missouri S&T, 600 W. 14th St,
Rolla, MO, 65409, United States,
guardiolai@mst.edu1 - Ensemble Methods With Disparate Data Sources For Stock
Market Prediction
Lin Lu, Auburn University, Auburn, AL, United States,
lzl0032@auburn.edu,Bin Weng, Fadel Mounir Megahed
Stock market has time critical characteristics which draws attentions from both
investors and researchers. The objective of this study is to develop a prediction
model for stock’s short-term movement forecasting. We assume more related data
sources used will increase the prediction performance. In this study, we consider
data from Wikipedia, Financial news, Market sentiment and Stock market history
data. Different features are generated from these data sources and data mining
methods are applied to select the most important ones. Next, ensemble methods
are used to develop the model. As a result, our prediction model dominates
related studies for the stock market forecasting.
2 - Occupancy Level Analysis At A VA Hospital That Considers
Discharge Of Patient Medical Decisions
Ivan G Guardiola, Associate Professor, Missouri S&T, 600 W. 14th
St, Rolla, MO, 65409, United States,
guardiolai@mst.edu,Tatiana Cardona
The improvement of short-term information is vital to obtain positive gains in
various hospital operational and business processes. To this end, the prediction or
forecasting of hospital census gives insight into hospital resource use that results
in better planning. This paper presents a combination of nonparametric and
parametric models to deal with the intra-week seasonality from the daily
discharge distribution.
3 - Neural Networks Based Linear Ensemble Framework for Time
Series Forecasting
Lin Wang, Huazhong University of Science and Technology,
Wuhan, China, Zhigang Wang
In this study, a combination forecasting model resulting from a novel ensemble
framework of four neural networks is proposed for time series forecasting. The
proposed framework has two primary advantages: (a) a heuristic to determine
the number of input and hidden neurons for each neural network, and (b) a
BPNN-BSA based mechanism for the associated combining weights. Both of the
advantages will improve the accuracy of each individual model and the final lin-
ear combination modle. Experimental results performed on nine time series
datasets show that the ensemble framework outperforms the component neural
network models and other well recognized models.
WA02
101B-MCC
Data Mining in Healthcare 1
Sponsored: Data Mining
Sponsored Session
Chair: Adel Alaeddini, University of Texas at San Antonio, Department
of Mechanical Engineering, One UTSA Circle, San Antonio, TX, 78249,
United States,
adel.alaeddini@utsa.eduCo-Chair: Anh Pham, University of Arkansas, 1411 S Washington
Avenue, Fayetteville, AR, 72701, United States,
anh.pham1234@gmail.com1 - Using Data Mining To Detect Fraud And Abuse Under National
Health Insurance System In China
Chong Li, Beijing Institute of Technology, Beijing, 100081, China,
lichongbit@163.com, Zihao Jiao, Huijuan Cao
Health care fraud and abuse are pressing problems, causing an important fraction
of total health expenditure wasted. Data mining methods can be used to
automatically detect fraud in billions insurance claim data, superior to the time-
consuming and practically efficient traditional auditing methods. Nevertheless,
few studies have been dedicated to this field in China. This paper presents how to
apply unsupervised methods to extract useful information and identify a smaller
subset from the claims for further assessment under China National Health
Insurance system. Our approach will help in streamlining auditing approaches
towards the suspect groups rather than routine auditing of all claims.
2 - Understanding The Association Of Clinical Characteristics Of Low
Grade Gliomas With Disease Outcomes
Anh Pham, Student, University of Arkansas, 1 University Avenue,
Fayetteville, AR, 72701, United States,
anh.pham1234@gmail.com,
Shengfan Zhang
Glioma is among the most prevalent and most devastating primary brain tumor.
Gliomas represent 28% of all brain tumors and 80% of malignant brain tumors.
70% of Low Grade Glioma patients eventually die from cancerous tumor
transformation. This study uses The Cancer Genome Atlas (TCGA) data to
understand relationships between different clinical characteristics of Low Grade
Glioma, such as tumor grades, tumor status, vital status and first presented
symptoms. Two data mining methods, association rules and decision trees, are
used.
3 - Modeling The Accumulation Of Comorbidities In Patients With
Multiple Chronic Conditions
Adel Alaeddini, University of Texas at San Antonio, Department of
Mechanical Engineering, One UTSA Circle, San Antonio, TX,
78249, United States,
adel.alaeddini@utsa.eduLong-lasting diseases known as chronic conditions can be considered as a staple
example of degradation processes that can progress and accumulate over time.
Approximately a quarter of all Americans and 75% of citizens aged 65 years and
older are burdened with two or more (multiple) chronic conditions (MCC). Here,
we introduce a latent regression Markov mixture (LRMM) model to explore
major patterns of disease accumulation in MCC patients and identify the risk
factors affecting the accumulation process. The new methodology will be
validated through a national healthcare dataset.
WA03
101C-MCC
Big Data
Contributed Session
1 - Discriminant Analysis And The Baseball Hall Of Fame
Tom Brady, Purdue University, 1401 S US Hwy 421, Westville, IN,
46391, United States,
tbradyjr@pnc.edu, Tom Brady
Baseball has long been referred to as the national past time in America. The most
fundamental discussions center around the inclusion, or exclusion of individual
players in the Baseball Hall of Fame. Election to this esteemed organization is
done on a purely subjective basis. The movie “MoneyBall” has highlighted the
recent trend towards using a more quantitative approach to managing and
operating a professional baseball team. The term Sabermetrics refers to the
application of quantitative techniques in all areas of baseball. In this paper, we
apply Discriminant Analysis to the selection problem faced by the Baseball Hall of
Fame members and analyze the performance of the process since its inception.
2 - Graphical Lasso And Thresholding: Conditions For Equivalence
Somayeh Sojoudi, University of California, Berkeley,
1543 Delaware Street, Berkeley, CA, 94703, United States,
somayeh.sojoudi@gmail.comGraphical lasso is a popular technique for finding a sparse inverse covariance
matrix from a small number of samples. Graphical lasso is computationally
expensive for large-scale problems due to a positive semidefinite constraint. A
cheap heuristic method for finding a graphical model is to simply threshold the
sample correlation matrix. By introducing the notions of sign-consistent and
inverse-consistent matrices, we derive sufficient conditions under which graphical
lasso and thresholding produce the same solution. These conditions are expected
to be satisfied for sufficiently sparse graphical models. We test the conditions on
electrical circuits and functional MRI data.
3 - Quantitative Compliance As A Driver For Automation
Leif Meier, Professor, University of Applied Sciences Bremerhaven,
An der Karlstadt 8, Bremerhaven, 27568, Germany,
lmeier@hs-bremerhaven.deCompliance management covers all efforts to comply with regulations such as
laws and rules, policies and standards. Automated processes are dealing with a
huge number of (trans-) actions to be executed in short term, depending on big
data sets. Each single transaction that is executed must comply with regulations
and must be transparent to auditors. Quantitative Compliance provides methods
to manage processes and risks in complex systems considering regulations to
improve decisions from available information. We provide an example to identify
risks from Anti-Money-Laundering (AML) in Financial Transactions and show
applications of this approach to data-driven systems in new areas.
WA03