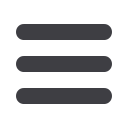

INFORMS Nashville – 2016
377
WA41
207C-MCC
Machine Learning for Finance
Sponsored: Financial Services
Sponsored Session
Chair: Justin Sirignano, University of Illinois at Urbana-Champaign,
Champaign, Champaign, IL, 61801, United States,
jasirign@illinois.edu1 - Recurrent Neural Networks For Modeling Financial Data
Justin Sirignano, University of Illinois at Urbana-Champaign,
Champaign, IL,
jasirign@illinois.eduWe explore using recurrent neural networks for modeling financial time series.
Recurrent neural networks depend upon the full history of the time series,
allowing for modeling long-term correlations. In out-of-sample tests on financial
data, we show recurrent neural networks can outperform standard feedforward
neural networks.
2 - Deep Learning For Mortgage Risk
Apaar Sadhwani, Stanford University,
apaars@gmail.comWe analyze mortgage risk at loan and pool levels using an unprecedented data set
of over 120 million mortgages originated in United States, which includes the
origination data, monthly updates on loan performance, and several time-varying
economic variables. We develop, estimate, and test dynamic models for mortgage
prepayment, delinquency, and foreclosure that capture loan-to-loan correlation.
At heart of our model is a deep neural network trained using GPU-accelerated
clusters. We develop several metrics to test model performance, which is a major
improvement over existing models and highlights the importance of local factors.
This is joint work with Justin Sirginano and Kay Giesecke.
3 - Background Subtraction For Pattern Recognition In High
Frequency Financial Data
Alex Papanicolaou, Integral Development Corporation,
alex.papanic@gmail.comFinancial markets produce massive amounts of complex data from multiple
agents, and analyzing these data is important for building an understanding of
markets, their formation, and the influence of different trading strategies. We
apply low-rank plus sparse background subtraction methods to high frequency FX
quote data. For prices in a single currency pair from many sources, we model the
market as a low-rank structure with an additive sparse component representing
transient market making behavior. We show case studies with real market data,
showing both in-sample and online results, for how the model reveals pricing
reactions that deviate from prevailing patterns.
WA42
207D-MCC
RM in Practice I
Sponsored: Revenue Management & Pricing
Sponsored Session
Chair: Wei Wang, Pros, Inc, 3100 Main St, Ste 900, Houston, TX,
77002, United States,
weiwang@pros.com1 - Dynamic Pricing And Learning In Airline Revenue Management
Ravi Kumar, PROS Inc,
rkumar04@pros.com,Wei Wang
Many airlines have been actively looking into class-free demand control
structures, which requires demand models where price varies over a continuous
interval. As evidenced both in literature and in practice one of the big challenges
in this setting is the trade-off between policies that learn quickly and those that
maximize expected revenue. We investigate applicability of recent advances in the
area of optimal control with learning. We examine a demand model where
customers maximum WTP is modeled as Gaussian and study approaches that
generate sufficient variability in pricing to ensure discovery of the underlying
customer behavior while providing appropriate level of expected revenue.
2 - Risk Management In Price And Revenue Optimization
Yanqi Xu, Princess Cruises,
yanqi6@yahoo.comPrice and revenue optimization has been instrumental in delivering profit lift for
companies across industries. Typical analytical models in this area involve
providing a forecast, and then use price and revenue optimization model to
balance supply and demand to extract the maximum profit for company’s assets.
In many cases, critical factors in the model are treated as deterministic and their
stochastic nature is frequently ignored, a trade-off for simplicity in models. The
utility of such solutions may be doubtful at best in situations where the modeled
factors have large variances. In this talk, we will discuss models that account for
risks in optimization, and show why it can be productive to do so.
3 - Implementing Optimal Decisions In Business Processes Using
4Ps: Proof Of Concept, Prototyping, Production, Performance
Sachin Sumant, Hertz Car Rental,
sumantsachin@yahoo.comIn the new era of analytics, there is abundance of data, analytical models,
visualization tools and integration technologies. Corporations are spending
millions of dollars in building analytical infrastructure in the hope of significant
ROIs. Large teams are getting formed and everybody is talking about “Next
Generation Systems” within analytics team and “Change Management” among
business users. This paper discusses how 4Ps can be utilized to unify the analytics
team and business users to achieve the optimal benefit from decision making
systems and processes by reducing rework, providing well-calibrated solutions,
increasing acceptability and guaranteeing positive results.
4 - Deriving Price Elasticity Estimates In The UK Cruise Market
John Harvey, Carnival UK,
johnandrewharvey@googlemail.comI shall outline the data-driven approach used in deriving a first set of price
elasticities for the UK Cruise Market, using purely observations from booking
history. By segmenting UK cruises based on their demand and price behaviour, I
will show how we approximated elasticity estimates through constructing
willingness-to-pay models and including a weighting factor based on the average
expected demand in different time intervals; we can estimate elasticities that
represent the impact of price at points in the booking curve versus an average
assumed weekly demand across the booking horizon.
WA43
208A-MCC
Spreading Decision Competencies
Sponsored: Decision Analysis
Sponsored Session
Chair: Chris Spetzler, Decision Education Foundation, DEF,
Palo Alto, CA, 00000, United States,
chris.spetzler1@gmail.com1 - Adding Social Impact To Research Efforts And Grants.
Ali Abbas, University of Southern California,
aliabbas@price.usc.edu2 - Teaching Decision Skills In College And Career Readiness
Frank Koch, Koch Decisions,
frank@kochdecision.comDuring the 2015-2016 school year, Thurston High School in Springfield Oregon
offered a College and Career Readiness class to juniors and seniors. The basic
principles of decision quality were taught as well as how to write effective essays
for college applications and how to plan to improve their college and career
decisions during the rest of the school year. We learned that many of the same
approaches used in business decision analysis are very effective with teenagers.
The approach that has been used at Thurston should be easily adaptable to other
schools as well as other organizations where the youth are starting to face
significant life decisions.
3 - Recent Advances In Spreading Decision Skills
Chris Spetzler, Decision Education Foundation,
chris@decisioneducation.orgThe Decision Education Foundation has been working on spreading decision skills
for more than a decade. Numerous opportunities exist for practitioners and
academics to contribute and help spread the word. This talk will discuss possible
collaboration scenarios.
WA44
208B-MCC
Environmental and Water Resources
Decision Analysis
Sponsored: Decision Analysis
Sponsored Session
Chair: Fengwei Hung, Johns Hopkins University, Baltimore, MD,
United States,
hfengwe1@jhu.eduCo-Chair: Liang Chen, Johns Hopkins University, Baltimore, MD,
United States,
chenliang1468@gmail.com1 - Case Studies In Water Resources Management For Sustainability
And Resilience
Cate Fox-Lent, US Army Corps of Engineers,
696 Virginia Rd, Concord, MA, 01742, United States,
Catherine.Fox-Lent@usace.army.mil,Igor Linkov
The US Army Corps of Engineers has several missions related to Environmental
Restoration, Water Quality, and goals for Sustainability and Resilience. Meeting
those goals requires life-cycle planning and decision making beyond the usual
project time horizon. This presentation will present 3 case studies of the various
ways in which formal decision analytics are integrated in to water resources
management in a Federal agency context.
WA44