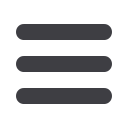

INFORMS Nashville – 2016
376
2 - Performance Of Affine Policies In Dynamic Robust Optimization
Omar El Housni, Columbia University, NYC, NY, United States,
omarhousni@hotmail.com,Vineet Goyal, Chaithanya Bandi
We study the performance of affine policy approximation for multi-stage robust
linear optimization problem under right hand-side uncertainty. Such problems
arise in many applications where right hand side models demand uncertainty.
Surprisingly, affine policies are not necessarily optimal even for simplex
uncertainty sets in multi-stage unlike two-stage. We show that affine policies give
a $O(\sqrt{ \lot T m})$-approximation if the uncertainty is a Cartesian product of
identical (or nested) uncertainty sets in each period and $O(\sqrt {Tm})$-
approximation for general multi-stage uncertainty.
3 - Robust And Reliable Solutions Bilevel Optimization Problems
Under Uncertainties
Zhichao Lu, Michigan State University, 16789 Chandler Rd
Apt 0436D, East Lansing, MI, 48823, United States,
luzhicha@msu.edu,Kalyanmoy Deb
Bilevel optimization problems have received a growing attention in the recent
past due to their relevance in practice. A number of studies on bilevel applications
and solution methodologies are available for deterministic set-up, but studies on
uncertainties in bilevel optimization are rare. In this paper, we suggest
methodologies for handling uncertainty in both lower and upper level decision
variables that may occur from different practicalities. For the first time, we discuss
and demonstrate the effect of uncertainties in each level on the overall definition
of a robust and reliable bilevel solution and present simulation results on a
number of problems.
4 - Uncertainty Quantification For Robust Optimization
Abhilasha Aswal, Phd Candidate, International Institute of
Information Technology, Bangalore, 26 Willow drive, Apt 8B,
Ocean, 07712, India,
abhilasha.aswal@iiitb.ac.in,Srinivasa Prasanna
We present a Hartley-like measure for quantification of information driving the
optimization using robust uncertainty sets. Based on this, we present
transformation based uncertainty equivalent materializations of alternative
uncertainty sets from a given uncertainty set. We show that using polyhedral
models for uncertainty leads to optimization models that are computationally
simpler than probabilistic alternatives, with potentially mixed continuous and
discrete variables.
WA39
207A-MCC
Joint Learning and Optimization in Supply Chain
Systems
Sponsored: Applied Probability
Sponsored Session
Chair: Cong Shi, University of Michigan, Ann Arbor, Ann Arbor, MI,
48105, United States,
shicong@umich.edu1 - Nonparametric Learning Algorithms For Optimal Base-stock
Policy In Perishable Inventory Systems
Huanan Zhang, University of Michigan,
zhanghn@umich.eduWe develop the first nonparametric learning algorithm for periodic-review
perishable inventory systems, where the firm does not know the demand
distribution a priori but makes the replenishment decision in each period based
only on the past sales (censored demand) data. It is well-known that even with
complete information about the demand distribution a priori, the optimal policy
for this problem does not possess a simple structure. Hence in this paper we focus
on finding the best base-stock policy, which performs near-optimal in these
systems. We establish a square-root convergence rate of the proposed algorithm,
which is the best possible for this class of problems.
2 - Dynamic Inventory Control With Stockout Substitution And
Demand Learning
Beryl Boxiao Chen, University of Illinois at Chicago, Chicago, IL,
United States,
boxchen@umich.edu, Xiuli Chao
Stock-out substitution is the phenomenon that if the primary choice of a
customer is out of stock, besides leaving the market immediately, the customer
may also substitute for other products. In this paper, we study a data-driven
inventory management problem and infer the customer substitution behavior
from historical sales data.
3 - Demand Estimation And Price Optimization With
Endogeneity Effect
He Wang, Massachusetts Institute of Technology, Cambridge, MA,
United States,
wanghe@mit.edu, Milashini Nambiar,
David Simchi-Levi
Inspired by many applications, we consider a revenue management setting
where heterogeneous products are offered sequentially over a finite horizon.
Demand for each product is modeled as a function of price, a product feature
vector, and a random noise. Our model allows for price endogeneity — a
common problem in the presence of heterogeneous product features — where
price is correlated with demand noise. We propose an online pricing algorithm
which uses randomized price shocks to estimate parameters via a two-step
regression. Theoretical and numerical evidence is provided to show the
performance of this algorithm.
WA40
207B-MCC
Probabilistic Combinatorial Optimization
Sponsored: Applied Probability
Sponsored Session
Chair: Alessandro Arlotto, Duke University, 100 Fuqua Drive,
Durham, NC, 27708, United States,
aa249@duke.edu1 - Limiting Theorems For The Optimal Alignments Score In Multiple
Random Words
Ruoting Gong, Illinois Institute of Technology,
rgong2@iit.eduLet X_{1}, ..., X_{m} be m independent sequences of i.i.d. random variables taking
values in a finite alphabet A. Let the score function S, defined on A^{m}, be non-
negative, bounded, permutation-invariant, and satisfy a bounded differences
condition. Under a variance lower-bound assumption, a central limit theorem is
proved for the optimal alignments score of the m random words. This is in
contrast to the Bernoulli matching problem or to the random permutations case,
where the limiting law is the Tracy-Widom distribution. In particular, when
S(x)=1_{x_{1}=x_{2}= ... =x_{m}}, a central limit theorem is obtained for the
length of the longest common subsequence of random words X_{1}, ..., X_{m}.
2 - Changing Graph Structure For Performing Fast, Approximate
Inference In Probabilistic Graphical Models
Areesh Mittal, The University of Texas at Austin,
areeshmittal@utexas.eduComplexity of exact marginal inference algorithms in probabilistic graphical
models is exponential in the treewidth of the underlying graph. We develop a
method to perform approximate inference on discrete graphical models by
modifying the graph to a new graph of lower treewidth. We prove error bounds
on the approximate inference solution compared to the exact solution. We
formulate the problem of finding parameters of the new graph which gives the
tightest error bounds as a linear program (LP). The number of constraints in the
LP grow exponentially with the number of nodes. To solve this issue, we develop
a row generation algorithm to solve the LP. We discuss heuristics for choosing the
new graph.
3 - Tight Adaptive Policies For The Dynamic And Stochastic
Knapsack Problem
Xinchang Xie, Duke University, Durham, NC, United States,
xinchang.xie@duke.edu, Alessandro Arlotto, Yehua Wei
In this talk, we consider a dynamic and stochastic knapsack problem in which
items have unitary values and the knapsack has finite capacity. A decision maker
is sequentially presented with n items with independent and identically
distributed sizes, and the goal of the decision maker is to maximize the expected
number of items that are included in a knapsack subject to the capacity
constraint. We propose adaptive selection policies that are tight. That is, such
adaptive policies have an expected number of selected items that is close to that of
the optimal policy.
4 - A Central Limit Theorem For Temporally Non-homogenous
Markov Chains With Applications To Dynamic Programming
Alessandro Arlotto, Duke University, Fuqua Drive, Durham, NC,
27708, United States,
aa249@duke.edu, J. Michael Steele
We prove a central limit theorem for a class of additive processes that arise
naturally in the theory of finite-horizon Markov decision problems. The main
theorem generalizes a classic result of Dobrushin (1956) for temporally non-
homogeneous Markov chains, and principal innovation is the summands are
permitted to depend on both the current state and a bounded number of future
states of the chain. We show through examples that this added flexibility gives
one a direct path to asymptotic normality of the optimal total reward of finite-
horizon Markov decision problems. The examples explain why such results are
not easily obtained by alternative Markovian techniques such as enlargement of
state space.
WA39