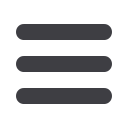

INFORMS Nashville – 2016
386
3 - Data-driven Diagnosis For Asthma Control Status In Smart
Asthma Management System Based On Correlated
Gamma-based Hidden Markov Model
Junbo Son, Assistant Professor, University of Delaware, Newark,
DE, United States,
sonjunbo@gmail.com,Shiyu Zhou,
Patricia Brennan
Driven by the IoT, a smart asthma management (SAM) system has been
implemented in practice. The SAM system includes rescue inhalers with a
wireless connection and the system records the inhaler usage and transmits the
data to a centralized server. To effectively manage the asthma, a statistical model
based on the patient monitoring data from the SAM system is crucial. In this
research, we propose a data-driven diagnostic tool for assessing underlying
asthma control status of a patient based on hidden Markov model (HMM). The
proposed correlated gamma-based HMM can visualize the asthma progression to
aid therapeutic decision making and its promising features are shown in both
simulation and case study.
4 - Reliability Analysis Considering Dynamic Material
Local Deformation
Wujun Si, Wayne State University,
fk9456@wayne.edu,Qingyu Yang, Xin Wu
We conduct reliability analysis utilizing dynamic material local deformation
information. A novel multivariate general path model with a new variance-based
failure criterion is proposed. A two-stage parameter estimation method is
developed to overcome the computational complexity. Both simulation studies
and physical experiments are conducted for verification and illustration.
WA67
Mockingbird 3- Omni
Data Analytics for System Improvement II
Sponsored: Quality, Statistics and Reliability
Sponsored Session
Chair: Xi Zhang, Peking University, Beijing, China,
xi.zhang@pku.edu.cnCo-Chair: Kaibo Liu, Universityof Wisconsin, Madison, 1513 University
Avenue, Madison, WI, 53706, United States,
kliu8@wisc.edu1 - Statistical Process Control Of Stochastic Textured Surfaces
Anh T Bui, Northwestern University, Evanston, IL, United States,
atbui@u.northwestern.edu,Daniel Apley
We develop a defect monitoring and diagnostic approach for manufactured
products that have stochastic textured surfaces (e.g., textiles or material
microstructures). We first use generic supervised learning methods to characterize
the stochastic behavior of “normal” in-control samples of the textured surfaces.
Based on the residuals of the supervised learning model applied to new samples
in a statistical process control context, we propose two spatial moving statistics for
detecting local aberrations in the textured surfaces. We illustrate the approach
using simulated and real examples.
2 - Causation-based Process Monitoring And Diagnosis For
Multivariate Categorical Processes
Xiaochen Xian, the University of Wisconsin, Madison, WI,
xxian@wisc.eduStatistical surveillance for multivariate categorical processes have attracted more
and more attentions. In many applications, causal relationships may exist among
categorical variables, where the shifts at upstream variables will propagate to their
downstream variables. We employ Bayesian network to characterize such causal
relationships and integrate it with the statistical process control technique. We
propose two control charts for detecting shifts in the conditional probabilities of
the multiple categorical variables that are embedded in the Bayesian network.
Both simulation and real case studies are used to demonstrate the effectiveness of
the proposed schemes.
3 - A Thermal Field Estimation Method Based On Spatial-temporal
Dynamics Using Multi-channel Sensor Data
Xi Zhang, Peking University, Beijing, China,
xi.zhang@pku.edu.cn,
Di Wang, Kaibo Liu
Thermal field profile is one of the critical issues for the quality assurance of the
grain warehouse. However, only limited sensors are afforded to characterize the
dynamics in the grainhouse, leading to an inappropriate decision for grain
maintenance. This article presents a field estimation approach to model spatio-
temporal dynamics of warehouse temperature through integrating
thermodynamics model and spatiotemporal stochastic processes. Specifically, we
integrate a 3-D unsteady heat transfer model into a Gaussian Markov random
field to achieve a parsimonious representation of spatial patterns. Simulation and
real case are conducted to show the effectiveness of the developed method.
4 - Multivariate Ordinal Categorical Process Control Based On
Log-linear Modeling
Jian Li, Xi’an Jiaotong University, Xi’an, China,
jianli@mail.xjtu.edu.cn,Junjie Wang, Qin Su
The quality of products or services is sometimes measured by multiple categorical
characteristics, each of which is classified into attribute levels such as good,
marginal, and bad. There is usually natural order among these attribute levels. By
assuming that each ordinal categorical quality characteristic is determined by a
latent continuous variable, this work incorporates the ordinal information into an
extended log-linear model and proposes a multivariate ordinal categorical control
chart. Simulations show that the proposed chart is efficient in detecting location
shifts and dependence shifts in the corresponding latent continuous variables of
ordinal categorical characteristics.
WA68
Mockingbird 4- Omni
Statistical Models for Computer Experiments
Sponsored: Quality, Statistics and Reliability
Sponsored Session
Chair: Qiong Zhang, Richmond, VA, United States,
qzhang4@vcu.edu1 - Efficient Gaussian Process Modeling For Computer Experiments
Yibo Zhao, Rutgers State University of New Jersey, Piscataway, NJ,
United States,
yz346@scarletmail.rutgers.eduWe study the problem of simultaneous variable selection and parameter
estimation in Gaussian process models. Conventional penalized likelihood
approaches are attractive but the computational cost of the penalized likelihood
estimation (PMLE) or the corresponding one-step sparse estimation (OSE) can be
prohibitively high as the sample size becomes large. This is because the likelihood
function heavily involves operations of a covariance matrix of the same size as the
number of observations. To address this issue, this article proposes an efficient
subsample aggregating (subagging) approach with an experimental design-based
subsampling scheme. The proposed method is computationally cheaper, yet it can
be shown that the resulting subagging estimators achieve the same efficiency as
the original PMLE and OSE asymptotically. The finite-sample performance is
examined through simulation studies. Application of the proposed methodology
to a data center thermal study reveals some interesting information, including
identifying an efficient cooling mechanism.
2 - Change-point Detection For Spatial-temporal Organ Image Data
Shuyu Chu, Virginia Tech,
cshuyu@vt.edu, Xinwei Deng, Ran Jin
The demand for organ transplantation increases rapidly, but only a limited
number of viable organs is available. Poor preservation and evaluation cause
many organs to be discarded. Current evaluation methods are often inaccurate or
result in organ damage. There is a great need for accurate non-invasive
evaluation methods. In this work, we focus on detecting quality changes in
organs under preservation by only using biomedical thermal image data. Scalable
Gaussian processes with expressive spectral mixture kernels is applied on the
large multidimensional image data to conduct model fitting and inference. A real
case study will be used to elaborate the performance of the proposed method.
3 - Asymmetric Process For Stochastic Simulation
Qiong Zhang, Virginia Commonwealth University,
qzhang4@vcu.eduQuantiles serve as important measurements in stochastic simulation. In
simulation practice, we need statistical methods to model these quantiles for
optimization or calibration. However, the traditional Gaussian process model
often fails to capture the behavior of quantiles if the sample path is not long
enough. To resolve this issue, we will introduce the asymmetric process for
modeling the quantiles in stochastic simulation. Numerical results will be
provided to show the effectiveness of this new approach.
WA67