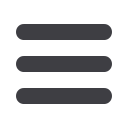

INFORMS Nashville – 2016
467
WD38
206A-MCC
General Session II
Contributed Session
Chair: Gang Wang, University of Massachusetts Dartmouth, 285 Old
Westport Rd, Room 214, North Dartmouth, MA, 2747, United States,
gwang1@umassd.edu1 - Pricing Decision Model For New, Upgraded And Remanufactured
Short-life Cycle Products
Che-Wei Yeh, PhD Student, National Taiwan University, Floor 9,
No.1, Sec. 4, Roosevelt Road, Taipei, Taiwan,
d99741008@ntu.edu.twDespite remanufacturing short life cycle products is rewarding economically as
well as environmentally, very little is known about modeling upgraded decisions
for products with short life cycle. In this paper, we develop a closed loop supply
chain model that optimizes the price for new, upgraded and remanufactured
products where demands are time-dependent and price sensitive. Using numerical
analysis, the findings give reasonable results and have important implications for
the impact of demand’s speed of change to the optimal prices.
2 - Effects Of The Adopting Distillers Grain In The Feed Ration For
Swine Industry In Argentina
Maria Celeste De Matteis, Graduate Assistant, University of
Tennessee, Knoxville, TN, United States,
mdematt1@vols.utk.edu,Tun-Hsiang Edward Yu
Driven by the Biofuels Law enacted in 2006, the production of corn-based
ethanol in Argentina has surged over the past decade. Distillers grain, a co-
product of corn ethanol, is expected to become an important feedstuff in
Argentine livestock feed ration because of its high content of protein and other
nutrition. Our study aims to evaluate the potential impact of adopting this
emerging feedstuff in the feed ration for Argentine hog industry. A multi-
objective model will be developed incorporating both feedstuff cost and animal
performance in the decision criteria.
3 - Integrated Operations Scheduling Under Different Penalty Terms
Gang Wang, University of Massachusetts Dartmouth, 285 Old
Westport Rd, Room 214, North Dartmouth, MA, 02747,
United States,
gwang1@umassd.eduThis paper studies an integrated operations scheduling problem under service
level contracts over a capacitated supply chain and considers three different
scheduling sub-problems in terms of the types of service level: 1) The first sub-
problem takes into account no specified service level (e.g., one-time transaction in
spot contracts); 2) the second is regarding single service level contracts, where
penalty function is convex; and 3) the third deals with multiple service level
contracts.
WD39
207A-MCC
Learning and Model Uncertainty in
Stochastic Systems
Sponsored: Applied Probability
Sponsored Session
Chair: Yuan Zhong, Columbia University, New York, NY, 10025,
United States,
yz2561@columbia.edu1 - Staffing Service Systems With Distributional Uncertainty
John Hasenbein, University of Texas-Austin,
jhas@mail.utexas.edu, Ying Chen
We examine the problem of staffing service systems in which either the exact
arrival rate or even the arrival rate distribution is unknown. The decision maker’s
goal is to minimize staffing costs while satisfying quality-of-service constraints on
the probability that a customer is delayed. We use bounds related to the Halfin-
Whitt approximation and prove asymptotic optimality of the proposed methods.
2 - Ambiguous Partially Observable Markov Decision Processes
Soroush Saghafian, Harvard University, Cambridge, MA,
United States,
soroush_saghafian@hks.harvard.eduWe present a generalization of Partially Observable Markov Decision Processes
(POMDPs) termed Ambiguous POMDP (APOMDP), which allows the decision
maker to take into account inevitable model ambiguities. We establish various
structural results, and discuss new opportunities for superior decision-making in
applications such as machine replacement, medical decision-making, inventory
control, revenue management, optimal search, bandit problems, and dynamic
principal-agent settings.
3 - How Being Distributionally Robust Can Improve Learning In
High Dimensions?
Karthyek R Murthy, Columbia University, New York, NY,
United States,
karthyek@gmail.com, Jose Blanchet, Yang Kang
In learning problems where the number of training samples is smaller than the
ambient dimension, usual empirical risk minimisation may not be enough to find
the best fit. We introduce RWPI, a novel learning methodology that is aimed at
enhancing out-of-sample performance in such settings. By casting the learning
problem as an optimization problem in the presence of model uncertainty, we
recover a wide range of regularisation procedures (such as generalized Lasso,
SVM) as particular cases. Further, an asymptotic analysis of a suitably defined
profile function allows to optimally select the regularisation parameter. We shall
discuss this optimality in the context of generalized Lasso.
4 - Learning And Hierarchies In Service Systems
Michail Markakis, Universitat Pompeu Fabra, Barcelona, Spain,
mihalis.markakis@upf.eduWe consider a service systems with servers that have different capabilities and
tasks whose types are ex-ante unknown. Information about a task’s type can only
be obtained while serving it. We show that the system’s stability region depends
on the entire distributions of service times, and that heavier tails cause greater
performance loss. We also consider endogenizing the servers’ capabilities, and find
that optimal designs have a “hierarchical” structure: all tasks are initially routed to
the least skilled servers and progressively move to more skilled ones, if necessary.
Comparative statics show that uncertainty in task types leads to higher training
costs and less specialized server pools.
WD40
207B-MCC
Applied Probability and Machine Learning III
Sponsored: Applied Probability
Sponsored Session
Chair: Daniel Russo, Northwestern University, 2001 Sheridan Road,
Evanston, IL, 60208-2009, United States,
dan.joseph.russo@gmail.com1 - Collaborative Filtering With Low Regret
Guy Bresler, MIT, Cambridge, MA, United States,
guy@mit.edu,
Devavrat Shah, Luis Voloch
Empirical evidence suggests that item-item collaborative filtering (CF) works well
in practice. Motivated to understand this, we provide a framework to design and
analyze recommendation algorithms. The setup amounts to online binary matrix
completion, where at each time a user requests a recommendation and the
algorithm chooses an entry to reveal in the user’s row. The goal is to maximize
the number of +1 entries revealed at any time. We analyze an item-item CF
algorithm that can achieve fundamentally better performance as compared to
user-user CF. Good “cold-start” performance is achieved by quickly making good
recommendations to new users about whom there is little information.
2 - Predicting The Unseen Mutations Provides A Roadmap For
Precision Medicine.
James Zou, Stanford University, Palo Alto, CA, 02139,
United States,
jamesyzou@gmail.comA fundamental question in genomics is to estimate the frequency distribution of
all the genetic variants in a population. This is a challenging task because we have
sequenced the genomes of relatively few individuals, and most existing mutations
are not observed in our samples. We give a non-parametric algorithm to estimate
the frequency distribution of all the variants, including the ones that not seen in
the sequenced individuals. We prove that also algorithm has strong finite-sample
convergence guarantees, and applied it to one of the largest human sequencing
data. Our estimates provide a roadmap for the discovery rate of large sequencing
efforts, including the Precision Medicine Initiative.
3 - Causal Inference With Random Forests
Stefan Wager, Stanford University, Stanford, CA, United States,
swager@stanford.eduMany scientific and engineering challenges, ranging from personalized medicine
to customized marketing recommendations, require an understanding of
treatment heterogeneity. We develop a non-parametric causal forest for
estimating heterogeneous treatment effects that extends Breiman’s widely used
random forest algorithm. Given a potential outcomes framework with
unconfoundedness, we show that causal forests are pointwise consistent for the
true treatment effect, and have an asymptotically Gaussian and centered sampling
distribution. We also propose a practical estimator for the asymptotic variance of
causal forests.
WD40