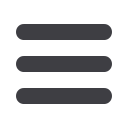

INFORMS Nashville – 2016
484
Wednesday, 4:30PM - 6:00PM
WE01
101A-MCC
Data Mining in Manufacturing
Sponsored: Data Mining
Sponsored Session
Chair: Mojtaba Khanzadeh, Mississippi State University,
21 Ace Avenue, 21 Apartments, Starkville, MS, 39759, United States,
mk1349@msstate.edu1 - A Congestion Prediction-based Dynamic Routing Model In
Automated Material Handling Systems
Sang Min Lee, Senior Researcher, Samsung Electronics, 816
ChanguiKwan, Korea Univ., 145 Anam-ro, Seongbuk-gu, Seoul,
Korea, Republic of,
smlee5679@gmail.com, Jee Hyuk Park
In automated material handling systems of semiconductor manufacturing,
vehicular congestion is a persistent problem resulting in the reduction of
production efficiency. In order to effectively route vehicles to reduce traffic
congestion, this study presents a congestion-avoidance routing model based on
congestion prediction. A congestion prediction model is proposed to predict the
possibility of probable heavy congestion that can lead to significant production
loss. The effectiveness of the proposed model is demonstrated by using real data
supplied by a semiconductor fabrication plant in South Korea.
2 - Profile Monitoring And Fault Diagnosis Via Sensor Fusion For
Multi-stream Data
Weihong Guo, Assistant Professor, Rutgers, The State University of
New Jersey, 96 Frelinghuysen Rd, CoRE Rm 220, Piscataway, NJ,
08854, United States,
wg152@rutgers.eduWhen multiple signals are acquired from different sources, sensor fusion and
data dimension reduction are two major issues to achieve a better comprehension
of the process. Methods for analyzing multi-stream profiles based on multilinear
discriminant analysis and ensemble learning are proposed in this research for the
purpose of profile monitoring, fault detection, and fault diagnosis. The proposed
methods are compared with state-of-the-art methods with both simulated and
real data.
WE02
101B-MCC
Data Mining Applications
Sponsored: Data Mining
Sponsored Session
Chair: Leily Farrokhvar, West Virginia University, 395 Evansdale Drive,
Morgantown, WV, 26506, United States,
leily@vt.edu1 - An Analysis Of Charitable Givings And Donor Behavior
Negar Darabi, Graduate Student Researcher, West Virginia
University, Morgantown, WV, 26506, United States,
nedarabi@mix.wvu.edu, Leily Farrokhvar, Azadeh Ansari
While charitable givings are typically a noticeable portion of the GDP and there is
abundant data available through tax forms, there has been few systematic studies
to identify contributing factors and predict donor behavior. Additionally, disasters
are shown to have a significant temporary effect on charitable givings. In this
study, we analyze the historic data using regression models to identify the most
influential factors and analyze impact of natural disasters on donor behavior.
WE03
101C-MCC
Big Data I
Contributed Session
1 - Crew Assignment Subject To Flight Delay Risks
Hing Kai Chan, Associate Professor, University of Nottingham
Ningbo China, 199 Taikang East Road, Room AB260, Ningbo,
315100, China,
hingkai.chan@nottingham.edu.cn, Sai Ho Chung,
Jing Dai
Crew cost ranks as the second highest cost of flight operations, but failure of
assigning sufficient crew members to a flight will lead to flight disruption such as
delay. The dilemma is obvious. This study adopts a big-data approach by utilizing
historical flight arrival delay data and a learning algorithm to predict such risks for
optimizing crew assignment. Numerical experiment demonstrates that the
proposed algorithm can increase the flight stability, meanwhile minimize the total
disruption cost induced.
2 - Developing A Dynamic Tool For Transplant Survival Analysis
Hamidreza Ahady Dolatsara, PhD Candidate, Auburn University,
Suite 3301, 345 West Magnolia Ave., Auburn, AL, 36849,
United States,
hamid@auburn.edu, Ali Dag, Bin Weng,
Fadel Mounir Megahed
This study present a toll developed for three types of survival analysis for the
transplants. In the first type, it estimates if a patient could survive certain time
windows which are integer multiples of one year. As the second type, it yield
probability of survival. This tool also estimates expected survival time. Surgeons
or other practitioners could utilize it based on their available data from their
patients and donors. These data are collected during the registration, waiting list,
operation, and after the operation. The tool utilizes machines learning methods to
identify the importation features and then utilizes the features for model training
and delivering a requested analysis.
3 - An Optimization Approach To Detection Of Epistatic Effects
Maryam Nikouei Mehr, PhD Student, Iowa State University,
3004 Black Engineering, Ames, IA, 50011, United States,
mnmehr@iastate.edu, Lizhi Wang
Epistasis refers to the phenomenon where the interaction of multiple genes affects
a certain phenotype more than they do separately. Similar epistatic effects are also
ubiquitous in other application areas, where a certain effect is only observable
when a particular combination of multiple factors is present. Due to the
enormous solution space, it’s hard to detect the epistatic effect. We propose an
optimization model that attempts to detect epistatic effects where a large number
of observations are available for a relatively small number of explanatory factors.
We will share our preliminary results and discuss future research directions.
4 - Warehouse Process Improvement Through Data Analytics
And Optimization
Vedat Bayram, Postdoctoral Research Fellow, University of
Waterloo, 200 University Ave. West, Waterloo, ON, N2L 3G1,
Canada,
vbayram@uwaterloo.ca, Fatma Gzara, Samir Elhedhli
With the advance of the technologies on collecting and storing data, warehouses,
from the most automated to the manual, generate large amounts of data.
Warehouse management companies are seeking ways to get the full value from
the massive amounts of data and use it as a competitive advantage in the
marketplace. In this presentation, we report on data analytics solutions for a
warehouse management and control systems company. We develop descriptive
tools to analyze the big data of e-retailing warehouses and identify process
improvement opportunities. We develop data driven optimization solutions and
validate by comparing to real system operation.
WE04
101D-MCC
Capacity-Expansion Planning with Increasing
Renewable Levels
Sponsored: Energy, Natural Res & the Environment, Energy I
Electricity
Sponsored Session
Chair: Ramteen Sioshansi, Ohio State University, 1971 Neil Avenue,
Columbus, OH, 43210, United States,
sioshansi.1@osu.eduCo-Chair: Antonio J. Conejo, The Ohio State University, 1971 Neil
Avenue, Columbus, OH, 43210, United States,
conejonavarro.1@osu.edu1 - Analyzing European Climate And Energy Policy Using
Stochastic Optimization
Asgeir Tomasgard, NTNU,
asgeir.tomasgard@iot.ntnu.noThe paper presents a modeling based analyses of decarbonization options for the
European power sector. Different support schemes designed to incentive early
development of CCS are studied, like public grants, feed-in premiums and
emission portfolio standards are evaluated. As an alternative we study storage and
transmission expansion in combination with a high renewable share. For the
analysis we use the EMPIRE model, a multi-horizon stochastic investment model
for the European power system that combines long-term capacity expansion with
operational modeling under different load and generation scenarios.
WE01