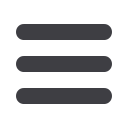

INFORMS Nashville – 2016
487
2 - Optimization Of Large Scale Tennessee Bioenergy Production
From Field To Blending Facility
Lixia He-Lambert, University of Tennessee, 2621 Morgan Circle,
310 Morgan Hall, Knoxville, TN, 37996, United States,
llamber3@utk.edu,Burton C. English, James Larson, Tun-Hsiang
Edward Yu, Bradly Wilson, Oleg Shylo
A large scale spatial-oriented mixed integer linear model was developed to
maximize the net present value of the Tennessee switchgrass-based biofuel supply
chain. Location of the production of feedstock within each five square-mile
hexagon was determined along with the location of candidate preprocessing
centers and biorefinaries. Shipping routes from field, and from biorefinary to
biorefinary and to blending facilities were determined.
WE11
104A-MCC
Sequential Stochastic Optimization
Sponsored: Optimization, Optimization Under Uncertainty
Sponsored Session
Chair: Xiangyu Gao, University of Illinois, 04 Transportation Building,
104 S. Mathews Ave, Urbana, IL, 61801, United States,
xgao12@illinois.edu1 - A Transformation Technique For Stochastic Optimization With
Decisions Truncated By Random Variables
Xiangyu Gao, UIUC, Urbana, IL, United States,
xgao12@illinois.edu,Xin Chen, Zhan Pang
A common technical issue encountered in many operations management models
is that decision variables are truncated by some random variables. The challenge
arising from this problem is that the objective functions are not convex in the
decision variables due to the truncation. To address this challenge, we develop a
powerful transformation technique which converts a non-convex minimization
problem to an equivalent convex minimization problem. We show that such a
transformation enables us to prove the preservation of some desired structural
properties,such as convexity, submodularity and L^natural-convexity, under
optimization operations.
2 - Stochastic Optimal Control Based Rental Of Production Capacity
Under Uncertain Production Requirements
Maurizio Tomasella, University of Edinburgh, Edinburgh, United
Kingdom,
Maurizio.Tomasella@ed.ac.uk,Artur Korinski
We model and analyse problems related to the optimal rental of manufacturing
capacity, when requirements for both demand and product technical
specifications evolve stochastically over time. We study a number of variants of
business models of capacity rental. Adopting Stochastic Optimal Control based
formulations, we derive the optimal closed-form policies that lead to choosing
rental solutions with respect to the more established models of full ownership of
manufacturing technology. A numerical case example involving high power fiber
laser sources, one of the leading technologies in materials processing, shows the
applicability of our optimal policies to real production environments.
WE12
104B-MCC
Recent Advances in Optimization and Related Topics
Sponsored: Optimization, Integer and Discrete Optimization
Sponsored Session
Chair: Sebastian Pokutta, Georgia Institute of Technology, Ferst Dr.,
Atlanta, GA, 30308, United States,
sebastian.pokutta@isye.gatech.edu1 - Structured Learning Revisited
Daniel Zink, Georgia Institute of Technology, Atlanta, GA, United
States,
daniel.zink@gatech.edu, Sebastian Pokutta, Gabor Braun
Frank-Wolfe techniques are popular due to their simplicity and more recently
Frank-Wolfe algorithms also gained significant traction for online learning. We
propose a new algorithm in the setting of online combinatorial optimization,
where the underlying feasible region is a 0/1 polytope P (e.g., paths in a graph).
We achieve the same regret bounds and convergence rates as Frank-Wolfe
techniques both in the online case and offline case, while gaining a significant
speed up in computation time. Moreover, our bounds hold in the online
structured learning setting, only depending on the l1-diameter of P and
independent of its actual (very large) dimension d.
2 - Approximate Hierarchical Clustering Via Lps
Aurko Roy, Georgia Institute of Technology, Atlanta, GA, United
States,
aurko@gatech.edu, Sebastian Pokutta
We study a cost function for hierarchical clusterings introduced by a recent work
of Dasgupta for which a top-down algorithm was given that returns a hierarchical
clustering of cost at most O(log1.5 n) times the optimal cost, using the ARV
sparsest cut algorithm as a subroutine. We improve this by giving an O(log n)-
approximation algorithm for this problem. Our main technical ingredients are a
combinatorial characterization of ultrametrics induced by this cost function,
deriving an IP formulation for this family of ultrametrics, and showing how to
round an LP relaxation of this formulation by using the idea of sphere growing
which has been extensively used in the context of graph partitioning.
3 - On The Computational Complexity Of Optimizing Over The
Chvatal Closure Of A Polytope
Dabeen Lee, PhD Student, Carnegie Mellon University, 5000
Forbes Avenue, Pittsburgh, PA, 15213, United States,
dabeenl@andrew.cmu.edu,Gerard P Cornuejols, Yanjun Li
In this paper, we study the computational complexity of some problems that arise
when taking the Chvatal closure of a polyhedron. Given a rational polytope
contained in the unit hypercube or a rational simplex that does not contain any
integer point, we prove that deciding emptiness of its Chvatal closure is NP-
complete. It has two implications; first, it is NP-hard to check whether a given
rational polytope contained in the unit hypercube or a rational simplex has
Chvatal rank 1; second, the optimization and separation problem over the
Chvatal closure of a given rational polytope contained in the unit hypercube or a
rational simplex is NP-hard as well.
WE13
104C-MCC
Disciplined Convex Programming for Nonconvex
Problems
Sponsored: Optimization, Computational Optimization and Software
Sponsored Session
Chair: Miles Lubin, Massachusetts Institute of Technology-ORC, MIT,
Cambridge, MA, 00000, United States,
mlubin@mit.edu1 - Polyhedral Approximation In Mixed-integer Convex Optimization
Miles Lubin, Massachusetts Institute of Technology, Cambridge,
MA, United States,
mlubin@mit.edu, Emre Yamangil, Juan Pablo
Vielma, Russell Bent
We present recent developments in the polyhedral outer approximation algorithm
for mixed-integer convex optimization derived from using disciplined convex
programming to automatically generate extended formulations from a user’s
algebraic input.
2 - Disciplined Convex-concave Programming
Steven Diamond, Stanford University, Stanford, CA, United States,
diamond@cs.stanford.edu, Xinyue Shen, Yuantao Gu, Stephen P
Boyd
In this talk we introduce disciplined convex-concave programming (DCCP),
which combines the ideas of disciplined convex programming (DCP) with
convex-concave programming (CCP). Convex-concave programming is an
organized heuristic for solving nonconvex problems that involve objective and
constraint functions that are a sum of a convex and a concave term. By
combining CCP with DCP, we obtain an automated system for applying CCP that
correctly handles subtleties such as the domains of functions and
nondifferentiability on the boundary of domains. We describe a Python
implementation called DCCP, which extends CVXPY.
3 - Outer-approximation Techniques For Mixed-integer Semidefinite
Optimization
Christopher Coey, Doctoral Student, Massachusetts Institute of
Technology, Cambridge, MA, 02139, United States,
coey@mit.edu,Miles Lubin, Emre Yamangil, Juan Pablo Vielma, Russell Bent
We will present an extension of the outer-approximation algorithm in Pajarito for
mixed-integer semidefinite optimization problems, and test the new approach
with computational experiments.
WE13