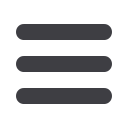

INFORMS Nashville – 2016
485
2 - A Convex Optimization Model For The Combined Electricity And
Natural Gas Expansion Planning Problem Under Gas-price
Volatility Considerations
Conrado Borraz-Sánchez, Associate Postdoctoral Researcher,
Los Alamos National Laboratory, Los Alamos, NM, United States,
conradob@lanl.gov,Russell Bent, Pascal Van Hentenryck,
Seth Blumsack, Hassan Lionel Hijazi
Recent trends towards installation of gas-fired power plants have increased the
economic growth and mutual dependency of electric power and natural gas
industries. These industries, however, have commercial, political and technical
constraints that often force them to plan, operate and manage in isolation. As a
result, adverse upshots may arise such as those experienced by both systems
during the winter of 2013/2014 in the US Northeast. Here, we present a joint gas-
grid elastic model to optimize required expansions to meet peak demand under
consideration of gas-price volatility caused by congested areas. We conduct
experiments on integrated test systems that include the New England area.
3 - Electricity Capacity Expansion And Cost Recovery With
Renewables
Ramteen Sioshansi, The Ohio State University, Integrated Systems
Engineering, 1971 Neil Avenue, Columbus, OH, 43210, United
States,
sioshansi.1@osu.edu, Yixian Liu
High levels of renewables can suppress electricity prices, reduce revenue for all
generation resources, and lead to uneconomic retirements and failure to make
needed investments. To analyze this problem quantitatively, two models are
utilized: (1) a multi-stage stochastic model seeking an optimal investment plan
with consideration of uncertainties and operating constraints; (2) a unit
commitment model giving electricity and reserve prices based on the investments.
Policies such as emission restrictions and renewable subsidies are considered in
this analysis. The investment model is large in scale and solved effectively by the
progressive hedging algorithm.
WE05
101E-MCC
Wildland Fire Management II
Sponsored: Energy, Natural Res & the Environment II Forestry
Sponsored Session
Chair: Matthew Thompson, U.S. Forest Service, 800 E Beckwith,
Missoula, MT, 59801, United States,
mpthompson02@fs.fed.us1 - Filling Requests For National Interagency Hotshot Crews
Erin Belval, Colorado State University, Fort Collins, CO, United
States,
mccowene@gmail.com,David Calkin, Matthew Thompson,
Yu Wei
Interagency Hotshot Crews are groups of 18 to 20 highly trained personnel used
in the United States for fighting wildland fires. Dispatching criteria for these crews
include minimizing travel distance and time, not taking crews away from areas
with high levels of predicted near-term fire activity and balancing workloads
across crews. We employ a simulation-optimization routine to examine the effects
of these factors on dispatching decisions during a fire season. We compare our
solutions with historical decisions using data from the Resource Ordering and
Status System.
2 - Advancements In Spatial Fire Planning
Matthew Thompson, U.S. Forest Service, 800 E Beckwith,
Missoula, MT, 59801, United States,
mpthompson02@fs.fed.us,Yu
Wei, Christopher Dunn, Christopher O’Connor, Greg Dillon, David
Calkin
Pre-fire assessment and planning can support incident management decision
making by dampening time pressures, reducing uncertainties, expanding options,
and clarifying risk-benefit tradeoffs that unfold over different time horizons. This
presentation will highlight the role of simulation and optimization in spatial fire
planning on federal lands in the western US, with an emphasis on factors relating
to cost, responder exposure, probability of success, and consequences.
3 - A Stochastic Optimization Model To Account For Climate Change
In Forest Planning
Jordi Garcia-Gonzalo, Centre Tecnològic Forestal de Catalunya
(CTFC)., Solsona, Spain,
j.garcia@ctfc.es,Andres Weintraub,
Cristóbal Pais
We consider a short/medium term multi-objective forest planning problem
considering harvesting decisions in the presence of uncertainty due to climate
change which impacts in the forest production. We introduce a multistage
Stochastic model considering multiple climate change scenarios and including the
corresponding non-anticipativity constraints. This enables the planner to make
more robust decisions than using a single average scenario.
4 - Learning Optimal Mobility And Demand Pressure from Fire
Resource Ordering Data
Alex Taylor Masarie, CSU and U.S. Forest Service, Fort Collins, CO,
80523, United States,
alex.masarie@gmail.com, Yu Wei, Matthew
Thompson, Michael Bevers, Iuliana Oprea, Erin Belval, David
Calkin
An inverse partial differential equation model is used to evaluate how fire
suppression resource allocation patterns vary with environmental and managerial
factors. This presentation will convey the physical basis for the applied math
technique demonstrating a finite difference approach to the spatial dynamics of
allocation. A calibration case study will relate features of continuous optimization
to operational research methodologies and present preliminary results.
WE06
102A-MCC
Optimization in Data Mining 2
Sponsored: Data Mining
Sponsored Session
Chair: Taghi Khaniyev, University of Waterloo, 200 University Ave.,
CPH 3669, Waterloo, ON, N2L 3G1, Canada,
thanalio@uwaterloo.ca1 - A Linear Separation Based MILP Model For Multi-class Data
Classification Problem
Fatih Rahim, Koç University, Rumelifeneri Yolu, Sarıyer, stanbul,
34450, Turkey,
frahim@ku.edu.tr,Metin Turkay
We address the multi-class data classification problem by a mixed integer linear
programming model (MILP). We split each class’s data set into subsets such that
the subsets of different classes are separable by a hyperplane. The hyperplanes
that separate a subset form a polyhedral region and the regions of different classes
are disjoint. A MILP model is used to find the optimal separation by minimizing
the total number of regions and misclassified data points. A preprocessing step is
proposed to decompose or simplify the problem considering pairwise separation
of classes. We evaluated two approaches for the testing phase, based on the
convex hulls of subsets and the regions defined by the hyperplanes.
2 - Robust Multicategory Support Vector Machines Using Difference
Convex Algorithm
Minh Pham, Postdoc Associate, University of Virginia, 1555
Montessori Ter, Charlottesville, VA, 22911, United States,
ptuanminh@gmail.comThe Support Vector Machine (SVM) is one of the most popular classification
methods in the machinelearning literature. In this paper, we focus on
classification in the angle-based framework,which is free of the explicit sum-to-
zero constraint, hence more efficient, and propose two robust MSVM methods
using truncated hinge loss functions. We show that our new classifiers can enjoy
Fisher consistency, and simultaneously alleviate the impact of outliers to achieve
more stable classification performance. To implement our proposed classifiers, we
employ the difference convex algorithm (DCA) for efficient computation.
3 - Scaling For Training Set Selection In Classification
Walter Dean Bennette, Air Force Research Lab, 7280 Lake View
Dr, Ava, NY, 13303, United States,
wdbennette@gmail.comTo allow for faster and better predictions from instance based classifiers such as k-
Nearest Neighbors, Training Set Selection techniques can be used to intelligently
select the classifier’s training dataset. However, Training Set Selection techniques
are limited in the size of datasets to which they can be practically applied. In this
work scaling approaches are introduced that improve the execution time of
Training Set Selection techniques. Results show that scaling methods maintain
data reduction rates and achieve acceptable levels of accuracy for experimental
datasets.
4 - Structure Detection In Mixed Integer Programs
Taghi Khaniyev, PhD Student, University of Waterloo, 200
University Ave., CPH 3669, Waterloo, ON, N2L 3G1, Canada,
thanalio@uwaterloo.ca,Samir Elhedhli, Safa F. Erenay
Bordered block diagonal structure in constraint matrices of integer programs lends
itself to Dantzig-Wolfe decomposition. We introduce a new measure of goodness
to capture desired features in such structures. We then use it to propose a new
approach to identify the best structure inherent in the constraint matrix. The
main building block of the proposed approach is the use of community detection
which alleviates one major drawback of the existing approaches in the literature:
predefining the number of blocks. When compared against the state-of-the-art
techniques, the proposed algorithm is found to identify very good structures,
require shorter CPU time, and lead to comparable dual bounds.
WE06