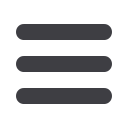

INFORMS Nashville – 2016
68
SB86
GIbson Board Room-Omni
Manufacturing II
Contributed Session
Chair: Gourav Dwivedi, Doctoral Student, Indian Institute of
Management, Indian Institute of Management, IIM Road, off Sitapur
Road, Lucknow, 226013, India,
fpm14013@iiml.ac.in1 - A Lagrangian Relaxation Approach For A Multiproduct Stochastic
Production Planning Problem
Reha Uzsoy, North Carolina State University, Dept. of Industrial &
Systems Engg, 300 Daniels Hall Camps Box 7906, Raleigh, NC,
27695-7906, United States,
ruzsoy@ncsu.edu, Erinc Albey,
Karl Kempf
We model a single-stage multi item capacitated production-inventory system with
stochastic demand. We present a chance-constrained production planning model
that considers forecast evolution, which is solve using Lagrangian relaxation.
Computational results show that the proposed approach outperforms previous
myopic capacity allocation procedures.
2 - Order Scheduling For A Class Of Electronic Ceramic
Manufacturers In Make To Order Environments
Zhongshun Shi, Peking University, Haidian Chengfu Road 298,
Founder Building Room 512, Beijing, 100871, China,
zhongshun@pku.edu.cn,Hongqiang Gao, Leyuan Shi
Motivated by the applications for a class of electronic ceramic manufacturers, we
study the order scheduling on sintering operations in make-to-order
environments, where sintering furnaces are modeled as batch processing
machines. The order consists of multiple types of jobs with specific demand
quantity. We consider the total weighted order completion time as objective
function and prove the problem is strongly NP-hard. Efficient heuristics with
worst-case analysis and asymptotic performance analysis are also developed.
Numerical results demonstrate that the proposed heuristics can give near-optimal
solutions for different production scenarios.
3 - A Lagrangian Approach For Coordinating Capacity Negotiations
In A Semiconductor Firm
Reha Uzsoy, North Carolina State University, Dept. of Industrial &
Systems Engg, 300 Daniels Hall Camps Box 7906, Raleigh, NC,
27695-7906, United States,
ruzsoy@ncsu.edu, Ankit Bansal,
Karl Kempf
We model the negotiations between a product development organization and a
production organization for access to manufacturing capacity for product
development activities in the semiconductor industry. We develop a negotiation
framework based on Lagrangian decomposition that maximizes overall firm
contribution subject to the resource constraints of both organizations. The
approach aims to achieve coordinated decisions between the two organizations,
and provides a benchmark for alternative models of negotiations.
4 - Modeling And Solution For Supply Chain Scheduling In
Cold Rolling
Shengnan Zhao, PhD Candidate, Northeastern University,
Shenyang, China,
zhaoshengnan_neu@163.com,Lixin Tang,
Qingxin Guo
This paper studies a supply chain scheduling problem which is derived from steel
production. The problem is to make coil schedules with the aim of balancing the
capacity of each production line, and minimizing the total setup cost. To describe
the problem, we formulate a MILP model with consideration of practical
technological requirements. Then we develop an improved discrete differential
evolution (DE) algorithm to solve it. The computational experiments show that
the proposed DE algorithm outperforms the compared DE algorithms for solving
this problem. In addition, the proposed algorithm is also competitive in
comparison with the commercial optimization solver CPLEX.
5 - Analysis Of The Barriers To Implement Additive Manufacturing
Technology In The Indian Automotive Sector:
A Fuzzy-ISM Approach
Gourav Dwivedi, Doctoral Student, Indian Institute of
Management, Indian Institute of Management, IIM Road, Off
Sitapur Road, Lucknow, 226013, India,
fpm14013@iiml.ac.in,
Rajiv K Srivastava, Samir K Srivastava
This paper analyzes the interaction among barriers to implement additive
manufacturing (AM) technology in the Indian automotive sector. We use Fuzzy-
Interpretive Structural Modeling (Fuzzy-ISM) method to derive hierarchy and
direction and to measure the strength of relations among these barriers.
Dominant barriers are identified using this approach. The findings may be useful
for managers to develop suitable mitigation strategies. This study contributes to
AM literature by the structured presentation of the barriers.
SB94
5th Avenue Lobby- MCC
Technologoy Tutorial: Palisade Corporation/Bayesia
1 - Palisade: Introduction To Risk And Decision Analysis Using
@RISK And The Decision Tools Suite
José Raúl Castro, Palisade Corporation, Ithaca, NY,
raul.castro.gc@gmail.comThis software presentation is designed to provide an entry-level introduction into
probabilistic analysis and will show how Monte Carlo simulation and other
techniques can be applied to your everyday business analyses. Using Monte Carlo
simulation, @RISK will analyze many different scenarios all at once, giving you
more insight into what could happen. We’ll look at example models including a
basic revenues/cost/profits model, an NPV model, and a Cost Estimation model, to
give you an idea of how quickly you can get started in probabilistic modeling in
Excel. If you build models in Excel then Palisade solutions can almost certainly
help you to make more informed decisions, right from your desktop. Palisade
software and solutions have been used to make better decisions. Cost estimation,
NPV analysis, operational risk registers, portfolio analysis, insurance loss
modeling, reserves estimation, schedule risk analysis, budgeting, sales forecasting,
and demand forecasting are just some of the ways in which the tools are applied.
This presentation will demonstrate how easy - and necessary - it is to implement
quantitative risk analysis in any business.
2 - Bayesian Networks & BayesiaLab: Artificial Intelligence for
Research, Analytics, and Reasoning
Stefan Conrady, BAYESIA USA, Franklin, TN, Contact:
stefan.conrady@bayesia.usThe objective of this workshop is to show that “Artificial Intelligence” should not
be perceived as a quasi-magic technology that is mostly incomprehensible to
normal mortals. We want to illustrate how scientists in any field of study—rather
than only computer scientists—can employ AI to explore complex problems. For
this purpose, we present Bayesian networks as the framework and BayesiaLab as
the software platform. In this context, we demonstrate BayesiaLab’s supervised
and unsupervised machine learning algorithms for knowledge discovery in high-
dimensional, unknown domains. Also, while AI is commonly associated with
another buzzword, “Big Data”, we wish to prove that AI can be useful for dealing
with problems for which we possess little or no data. Here, expert knowledge
modeling is critical, and we describe how even a minimal amount of expertise can
serve as a basis for sound reasoning aided by AI.
Sunday, 1:30PM - 3:00PM
SC01
101A-MCC
Supervised and Unsupervised Methods
Sponsored: Data Mining
Sponsored Session
Chair: Wenjun Zhou, University of Tennessee, 916 Volunteer Blvd,
255 Stokely Management Center, Knoxville, TN, 37996-0525,
United States,
wzhou7@gmail.com1 - Group-wise Sufficient Dimension Reduction: With Applications In
Forecasting The Equity Risk Premium
Haileab Tesfe Hilafu, University of Tennessee,
hhilafu@utk.eduWhen there is prior domain knowledge concerning a grouping structure of the
predictors, two different approaches of dimension reduction exist: carry out
dimension reduction of predictors in each group separately which ignores the
inter-dependence among the groups; ignore the grouping structure and reduce
the dimension of the predictors jointly. We present a method that bridges these
two approaches in the sense that it, simultaneously, utilizes the prior domain
knowledge and accounts for potential inter-dependence among the groups of
predictors. The proposed method is applied to forecast the equity risk premium
from a set of well known macroeconomic and a set of technical variables.
2 - Sufficient Dimension Reduction For Treatment Effect Estimation
Craig Anthony Rolling, Saint Louis University College for Public
Health and Social Justice, 1 North Grand Boulevard, Saint Louis,
MO, 63103, United States,
rollingca@slu.edu, Wenbo Wu
For nonparametric methods of estimating the treatment effect, if the dimension of
the baseline covariates is large, implementation becomes difficult and sometimes
infeasible due to the curse of dimensionality. Hence, sufficient dimension
reduction of baseline covariates can be useful before estimating the treatment
effect. We refer to such a dimension reduction subspace as a central treatment
effect subspace (CTES). We propose methods to estimate the CTES and its
structural dimension, investigate the theoretical properties of these estimators,
and demonstrate their effectiveness with numerical studies.
SB86