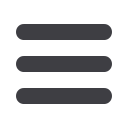

INFORMS Nashville – 2016
73
SC13
104C-MCC”
Advances in Mixed Integer Polynomial Optimization
Sponsored: Optimization, Global Optimization
Sponsored Session
Chair: Akshay Gupte, Clemson University, O-321 Martin Hall, Clemson
University, Clemson, SC, 29634, United States,
agupte@clemson.edu1 - Exploiting Permutation Invariance To Construct Tight Relaxations
Mohit Tawarmalani, Purdue University, West Lafayette, IN,
United States,
mtawarma@purdue.edu,Jinhak Kim,
Jean-Philippe P. Richard
We construct the convex hull for a set that does not change when the variables
are permuted. We illustrate the technique by developing (1) convex hull of
matrices with bounded rank and spectral norms, (2) convex envelopes of multi-
linear functions over certain domains, and (3) a novel reformulation and
relaxation for sparse principal component analysis.
2 - Intersection Cuts And S-free Sets For Polynomial Optimization
Chen Chen, Columbia University, New York, NY, United States,
chen.chen@columbia.edu, Daniel Bienstock, Gonzalo Munoz
We develop an intersection cut for generic problems with closed sets. The cut
relies on a violation distance oracle and it can be computed in polynomial time in
the special case of polynomial optimization. Additional cuts are presented based
on S-free sets or convex forbidden zones; for polynomial optimization we adopt
the specialized term of outer-product-free sets. We provide some insight into the
nature of maximal outer-product-free sets and present two classes of such sets.
These two classes give intersection cuts that can be computed in polynomial time.
Furthermore, the associated intersection cuts can be strengthened in the case of
intersections at infinity.
3 - On The Strength Of Linear Approximations For
Multilinear Monomials
Yibo Xu, Clemson University, Clemson, SC, United States,
yibox@clemson.edu, Warren P Adams, Akshay Gupte
We analyze worst-case errors associated with approximating multi-linear terms
over bounded variables when using linear functions. The error associated with a
linear function at a given point is the absolute difference between the actual and
functional values. These errors turn out to be dependent on the variable bounds.
We identify “best” linear functions that yield the smallest worst-case errors for
various sets of bounds, and identify those points at which these errors are
realized. The errors favorably compare with those obtained by convex hull
representations.
4 - Iterative LP And SOCP-based Approximations To
Semidefinite Programs
Georgina Hall, Princeton University, Princeton, NJ, United States,
gh4@princeton.edu, Amir Ali Ahmadi
We develop techniques for approximating SDPs with LPs and SOCPs. Our
algorithms iteratively grow an inner approximation to the PSD cone using a
column generation scheme and/or a change of basis scheme involving Cholesky
decompositions.
SC14
104D-MCC
Syngenta Crop Challenge in Analytics
Invited: Agricultural Analytics
Invited Session
Chair: Durai Sundaramoorthi, Washington University in Saint Louis,
Campus Box 1156, One Brookings Drive, Saint Louis, MO, 63130-
4899, United States,
dsundaramoorthi@gmail.com1 - Hierarchical Modeling Of Soybean Variety Yield And Decision
Making For Future Planting Plan
Huaiyang Zhong, Stanford University,
hzhong34@stanford.edu,
Xiaocheng Li, David J Lovell
We introduce a novel hierarchical machine learning mechanism for predicting
soybean yield that can achieve a median absolute error of 3.74 bushels per acre in
five-fold cross-validation. Further, we integrate this prediction mechanism with a
weather forecasting model, and propose three different approaches for decision
making under uncertainty to balance yield maximization and risk.
2 - Balancing Weather Risk And Crop Yield For Soybean
Variety Selection
Ling Tong, University of Iowa, Iowa City, IA, United States,
ling-tong@uiowa.edu, Bhupesh Shetty, Samuel Burer
We propose an optimization-based method to assist a farmer’s choice of soybean
varieties to plant in order to maximize expected yield while also managing risk,
where the primary uncertainty faced by the farmer is due to seasonal weather
patterns. By solving a sequence of MIPs, we calculate the efficient frontier
between the two competing objectives of maximizing expected yield and
guaranteed yield over all possible season types. The coefficients of the MIPs are
estimated using a multiple-linear-regression model and a Bayesian-updating
scheme applied to the training and evaluation data. Using the efficient frontier,
the farmer may choose an optimal solution that fits his/her risk-reward profile.
3 - Decision Assist Tool For Seed Variety To Provide Best Yield In
Known Soil And Uncertain Future Weather Conditions
Mehul Bansal, Robert Bosch Engineering and Business Solutions,
Bengaluru, Karnataka, India,
Mehul.Bansal@in.bosch.comNataraj Vusirikala
The gap between agriculture produce and demand is ever increasing due to
growing world population. There is an urgent need for utilizing all possible
methods and technology solutions to bridge this gap. One of the key challenges to
increase the agricultural produce is the ability to take right decisions under
uncertain climate and weather conditions. In this paper we discuss a method to
provide decision assist to the farmer on the best variety of soybean seed to be
sown at the start of a season. In order to optimize the yield under uncertain
conditions, we use a combination of crop yield modeling, weather forecasting and
portfolio optimization techniques to suggest best combination of soybean seed
variety. The data used in this method is the historical soybean produce data and
the corresponding soil and weather conditions under which the yield was
produced, day-wise weather data (temperature, precipitation and solar radiation)
at farm sites from 2008 to2014. We recommend planting the following varieties
with the given percentages at site 2290 for year 2016: (i) 10% of Variety V107,
(ii) 35% of Variety V179, (iii) 10% of Variety V189, (iv) 10% of Variety V193, and
(v) 35% of Variety V46.
SC15
104E-MCC
Optimization and Learning in
Biomedical Applications
Invited: Modeling and Methodologies in Big Data
Invited Session
Chair: Mengdi Wang, Princeton University, NY, United States,
mengdiw@princeton.edu1 - Latent Graphical Models For Mixed Data
Yang Ning, Princeton University, Princeton, NJ, United States,
yning@exchange.Princeton.EDU, Jianqing Fan, Han Liu, Hui Zou
Graphical models are commonly used tools for modeling multivariate random
variables. While there exist many convenient multivariate distributions such as
Gaussian distribution for continuous data, mixed data with the presence of
discrete variables or a combination of both continuous and discrete variables
poses new challenges in statistical modeling. In this talk, we propose a
semiparametric model named latent Gaussian copula model for binary and mixed
data.
2 - Hierarchical Knowledge-gradient With Stochastic Binary
Feedbacks With An Application In Personalized Health Care
Yingfei Wang, Princeton University, Princeton, NJ, United States,
yingfei@cs.princeton.edu, Warren B. Powell
Motivated by personalized health care problems, we consider the problem of
sequentially making decisions that are rewarded by ``successes’’ and ``failures’’
which can be predicted through an unknown relationship that depends on a
partially controllable vector of attributes for each instance. The learner takes an
active role in selecting samples from the instance pool. The goal is to maximize
the probability of success. Our problem is motivated by healthcare applications
where the highly sparsity makes leaning difficult. With the adaptation of an
online boosting framework, we develop a knowledge-gradient (KG) policy to
guide the experiment by maximizing the expected value of information.
3 - Approximate Newton-type Methods With Cubic Regularization
Saeed Ghadimi, Princeton University, Princeton, NJ, United States,
sghadimi@princeton.edu, Tong Zhang, Han Liu
In this talk, we consider a class of second order methods for solving convex
optimization problems. In particular, we propose Newton-type methods with
cubic regularization when hessian of the objective function is not completely
available. Convergence analysis of these methods under different conditions like
stochastic setting are also presented.
SC15