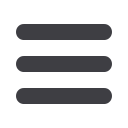

S102
ESTRO 36 2017
_______________________________________________________________________________________________
Making accurate prediction for these outcomes requires
data on many patients, preferably from many institutions
using different treatment protocols. Also these data needs
to be of high quality, rich in its content and will come from
many data domains (clinical, imaging, omics, society,
quantified self). Integration of data across these domains
and across hospitals worldwide and analysis of such
complex datasets is the topic of this seminar.
With lung cancer as the running example, known
prognostic and predictive factors for outcomes after
radiotherapy will be summarized to show the domains
from which data needs to be integrated and the challenges
associated with each domain. The problem of
dimensionality reduction, risk of over-fitting and need for
robust validation will be detailed. Then the need and
barriers for integration data across vertical data partitions
(e.g. departments in a hospital having a ‘slice’ of data for
a give patient) and across horizontal partitions (e.g. each
hospital has a patient cohort with data) will be discussed.
Finally, some example implementations and first results
of efforts to integrate and analyse these complex data will
be shown.
SP-0203 Innovative clinical trial designs for
Personalised Radiation Oncology
S. Brown
1
1
University of Leeds, Institute of Clinical Trials
Research, Leeds, United Kingdom
Radiation oncology research requires use of appropriate
novel trial designs and robust statistical strategies to
ensure reliable decision making. Phase I/II drug trials
typically focus on identifying the maximum tolerated dose
of a novel therapy. In the context of radiation oncology,
this concept is not so clear cut. Various aims of phase I/II
trials investigating radiotherapy-novel agent combinations
include identifying optimum scheduling, determining the
optimum biologic dose, and obtaining preliminary
estimates of efficacy. Difficulties in attributing toxicities
and evaluating longer-term toxicity also pose problems in
designing early phase radiation oncology trials.
Opportunities exist to apply novel clinical trial designs to
the setting of phase I and II trials in radiation oncology, to
overcome some of these difficulties, and to also enable
investigation of a number of novel agent-radiotherapy
combinations together. Several practical designs
addressing this will be discussed in this session, as well as
those taking into account known biomarkers in the
evaluation of efficacy.
SP-0204 Decision support systems and shared decision
making
M.A. Gambacorta
1
, G. Chiloiro
1
, C. Masciocchi
1
, N.
Dinapoli
1
, F. Cellini
1
, A. Re
1
, V. Valentini
1
1
Università Cattolica del Sacro Cuore -Policlinico A.
Gemelli, Radiation Oncology, Rome, Italy
The personalized approach to cancer patient requires a
shared decision which derives from the contribution of
every single specialist involved in the cure of any single
person affected by cancer. The tumor behaviour and
patient outcomes are related to several factors. These
factors and their interactions are sometimes poorly known
by the doctors who will take the therapeutic decision for
the patients. Different branches of medicine have
developed their own lines of research which are
sometimes difficult to be interpreted, difficult to be
integrated with classical clinical factors and for these
reasons, difficult to be applied in clinical practice. In the
clinical prediction and decision making process, results
provided by these researches are rarely included, whereas
clinicians usually use few clinical and imaging data for
understanding tumor behaviour, predicting patients'
outcomes and thus for choosing the the most
suitable treatment. In the last years, in the literature,
several studies based on the analysis of large data-base
started to appear. These type of studies uses an advanced
statistic aiming to find the connections between different
covariates in predicting outcomes. The clinical decision
is usually based on general guidelines which extrapolate
information from randomized clinical trial (RCT).
Moreover independent factors, derived from several RCT,
are used by the Oncologists to make his prevision on tumor
behaviour and consequently to choose the „right
treatment“ for a specific patient. RCT enclose patients
with characteristics chosen beforehand and usually the
innovative information are never or rarely included. This
lead to a potential miss of several information that could
refine prediction and thus promote personalized
treatments and to an erroneous outcomes prediction that
can lead to un-appropriate treatment decision for a
specific patient. Integrative data analysis has the
potential to correlate data of different origins (genetic,
radiology, clinic,...) with patient’s outcomes and to
create a consistent dataset useful to obtain a trustful
analysis for the Decision Support System (DSS). The DSS
can easily be applied in clinical practice helping the
Oncologist to merge several information that otherwise
would be excluded in the process of decision making. The
possibility to predict the outcome for a certain patient in
combination with a specific treatment with more
accuracy, will lead to better identification of risk groups
and thus better treatment decisions in individual patients,
but it will also stimulate research focused on specific risk
groups which try to find new treatment options or other
combinations of treatment options for these subgroups.
These treatments will be more personalized, which will
not only save patients from unnecessary toxicity and
inconvenience, but will also facilitate the choice of the
most appropriate treatment . The resulting predictive
models, based on patient features, enable a more patient
specific selection from the treatment options menu and a
possibility to share decisions with patients based on an
objective evaluation of risks and benefits. Finally,
considering the important role that predictive models
could play in the clinical practice, clinicians must be
aware of the limits of these prediction models. They need
to be internally validated taking into account the quality
of the collected data. An external validation of models is
also essential to support general applicability of the
prediction model. Therefore structural collaboration
between different groups is crucial to generate enough
anonymized large databases from patients included or not
in clinical trials.
Symposium: Safety and clinical and cost effectiveness of
multi-modality IGRT and ART
SP-0205 What evidence is needed to assess cost-
effectiveness of new technology and how can we get it
(easily)?
M. Johannesma
1
1
Health Insurance Company CZ, Department Innovation &
Advice, Tilburg, The Netherlands
The incidence of cancer is increasing worldwide. By this,
but also due to the rapid diffusion of new technologies,
there is a continues rise in health care costs. For decision-
making, primary studies of costs of health technology are
gaining importance and cost-effectiveness analysis are
increasingly used to estimate the incremental health gain
for an incremental use of resources. Economic evaluation
in health care, the comparative analysis of alternative
interventions in terms of both their costs and
consequences, are performed with the aim to determine
the value for money of new treatments compared to the
prevailing standard. In their aim to support decision
making in health care, they represent one aspect within