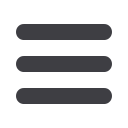

S437
ESTRO 36 2017
_______________________________________________________________________________________________
quality. Furthermore this approach enables the
identification of problematic plans beforehand.
PO-0822 An evolutionary model improvement strategy
for knowledge-based planning
Y. Zhang
1
, F. Jiang
1
, H. Yue
1
, S. Li
1
, Q. Hu
1
, M. Wang
2
, H.
Wu
1
1
Key Laboratory of Carcinogenesis and Translational
Research Ministry of Education/Beijing- Department of
Radiation Oncology- Peking University Cancer Hospital &
Institute, Department of Radiation Oncology, Beijing,
China
2
National Institute for Radiological Protection- China
CDC, National Institute for Radiological Protection-,
Beijing, China
Purpose or Objective
It was reported that RapidPlan, a knowledge-based
solution, can improve planning efficiency, quality and
consistency. Should the performance of RapidPlan be
dependent on the quality of the plans training the model,
this study hypothesizes that RapidPlan can improve its
constituent plans (closed-loop) and improve the model
itself by incorporating the better knowledge (evolution).
Moreover, the maximum number of iterations to exhaust
the full potential was also tested.
Material and Methods
An initial RapidPlan model (M
0
) for pre-surgical rectal
cancer patients was configured using 81 best-effort
manual VMAT plans (P
0
) with SIB (50.6 Gy and 41.8 Gy to
95% PTV
boost
and PTV respectively). For simplification,
decreased or increased mean dose to both femoral head
(FH) and urinary bladder (UB) were considered as
improved (P
+
) or worsened plans (P
-
) respectively. P
±
denoted intertwined plans. The first closed-loop iteration
of re-optimizing P
0
using M
0
yielded P
1
: 69 P
1+
, 12 P
1±
and
0 P
1-
. By substituting P
1+
for their corresponding P
0
, the
library of model M
1+
consisted of 12 P
0
and 69 P
1+
. The
second closed-loop iteration of re-optimizing P
0
using M
1+
produced 35 P
2+
that were superior to both P
0
and P
1
,
hence the knowledge base of M
2+
composed 9 P
0
, 37 P
1+
and 35 P
2+
. As open-loop validation, 30 clinical plans (P
v
)
that were not included in the model were re-optimized
using each model. Re-optimization maintained all
parameters except the MLC sequences were redesigned
using the objectives generated by the models.
Renormalizations to target prescriptions were performed
to make OAR dose comparable.
Results
Consistent with literature, knowledge-based so lution
improved the plan quality in both closed- and open-loop
validations than the conventional trial-and-error process.
In the first closed-loop evolution, the mean±SD of D
mean_FH
and D
mean_UB
for 69 P
1+
were 12.88±1.38 Gy and 23.06±3.11
Gy respectively, which were significantly lower by 23.70%
and 9.53% than the corresponding values of P
0
(P<0.05). In
the second round of closed-loop re-optimization, the
D
mean_FH
of the 35 P
2+
decreased to 11.12±1.48 Gy
(corresponding P
0
: 17.13±2.06 Gy; P
1
: 13.08±1.36 Gy), and
D
mean_UB
decreased to 22.80±3.72 Gy (corresponding P
0
:
25.79±3.34 Gy; P
1
: 23.41±3.59 Gy). Table 1 and figure 1
display the open-loop validation results for various
models. The marginal disparities of HI and CI
(magnitudes
≦
0.04) and largely overlapped DVH lines
indicated comparable target dose distribution. In line with
the closed-loop test, RapidPlan reduced the dose to OARs
massively than clinical plans in the open-loop validation
(Fig 1). The first model evolution has greatly and
significantly lowered the dose to FH at comparable dose
to the UB and targets. The second iteration has made little
difference though.
Conclusion
RapidPlan model evolves when the sub-optimal
constituent training sets were replaced by the improved
plans that were re-optimized by the model. One iteration
is most cost-effective.
PO-0823 Hierarchical constrained optimization for
automated SBRT paraspinal IMRT planning
M. Zarepisheh
1
, L. Hong
1
, J.G. Mechalakos
1
, M.A. Hunt
1
,
G.S. Mageras
1
, J.O. Deasy
1
1
Memorial Sloan Kettering, Medical Physics, New York,
United States Minor Outlying Island
Purpose or Objective
To develop a fully automated approach to IMRT treatment
planning using hierarchical constrained optimization and
the Eclipse API for SBRT paraspinal cases.
Material and Methods
This study formulates the IMRT treatment planning
problem as a hierarchical constrained optimization (HCO)
problem (also known as prioritized optimization). HCO
prioritizes the clinical goals and optimizes them in ordered
steps. In this study, we maximize tumor coverage first and
then minimize critical organ doses in the subsequent steps
based on their clinical priorities (e.g., (1) maximize tumor
coverage, (2) minimize cord or cauda dose, (3) minimize
esophagus dose,...). For each organ we define an
objective function, based on the gEUD concept, which
correlates with the clinical criterion. At each step, we
preserve the results obtained in the prior steps by treating
them as hard constraints with a slight relaxation or ”slip”
to provide space for subsequent improvement. Maximum
dose criteria to the tumor and other organs is always
respected through hard constraints applied at all steps.
To solve the resultant large-scale constrained
optimization problems, we use two commerical solvers,
knitro
and
ampl
. The Eclipse API is used to pull patient
data needed for optimization (e.g., beam geometries,