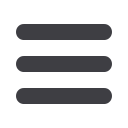

S849
ESTRO 36 2017
_______________________________________________________________________________________________
therapy.
Conclusion
We found significant correlations between the occurence
of early toxicities and dose-volume parameters of
associated organs at risk for patients with primary brain
tumours or prostate cancer receiving proton therapy. A
reduction of NTCP could be predicted for proton therapy
based on comparative treatment planning. After
validation, these results may be used to identify patients
who are likely to benefit most from proton therapy, as
suggested by the model-based approach [1].
[1] Langendijk JA et al. (2013) Radiother Oncol 107, 267 -
273.
EP-1596 Developing and validating a survival prediction
model for NSCLC patients using distributed learning
A. Jochems
1
, T. Deist
1
, I. El-Naqa
2
, M. Kessler
2
, C. Mayo
2
,
J. Reeves
2
, S. Jolly
2
, M. Matuszak
2
, R. Ten Haken
2
, J. Van
Soes
1
, C. Oberije
1
, C. Faivre-Finn
3
, G. Price
3
, P. Lambin
1
,
A. Dekker
1
1
MAASTRO Clinic, Radiotherapy, Maastricht, The
Netherlands
2
University of Michigan, Radiation oncology, Ann-Arbor,
USA
3
The University of Manchester, Manchester Academic
Health Science Centre, Manchester, United Kingdom
Purpose or Objective
The golden standard for survival prediction in NSCLC
patients, the TNM stage system, is of limited quality for
patients receiving (chemo)radiotherapy[1]. In this work,
we develop an up-to-date predictive model for survival
prediction based on a large volume of patients using a big
data distributed learning approach. Distributed learning is
defined as learning from multiple patient datasets without
these data leaving their respective hospitals.
Furthermore, we compare performance of our model to a
TNM stage based model. We demonstrate that the TNM
stage system performs poorly on the validation cohorts,
whereas our model performs significantly above the
chance level.
Material and Methods
Clinical data from 1299 lung cancer patients, treated with
curative intent with chemoradiation (CRT) or radiotherapy
(RT) alone were collected and stored in 3 different cancer
institutes. Two-year post-treatment survival was chosen
as the endpoint. Data from two institutes (1152 patients
at Institute 1 and 147 at Institute 2) was used to develop
the model while data from the 3
rd
institute (207 patients
at Institute 3) was used for model validation.
A Bayesian network model using clinical and dosimetric
variables was adapted for distributed learning (watch the
animation: link censored). The Institute 1 cohort data is
publicly available at (link censored) and the developed
models can be found at (link censored).
Results
A Bayesian network (BN) structure was determined based
on expert advice and can be observed in figure 1. Variables
included in the final model were TNM stage, age,
performance status, and total tumor dose. The BN model
has an AUC of 0.67 (95% CI: 0.59–0.75) on the external
validation set and an AUC of 0.65 on a 5-fold cross-
validation on the training data. A model based on TNM
stage performed with an AUC of 0.49 (95% CI: 0.39-0.59)
on the validation set.
Conclusion
The distributed learning model outperformed the TNM
stage based model for predicting 2-year survival in a
cohort of NSCLC patients in an external validation set (AUC
0.67 vs. 0.49). This approach enables learning of
prediction models from multiple hospitals while avoiding
many boundaries associated with sharing data. We believe
that Distributed learning is the future of Big data in health
care.
References
[1] Dehing-Oberije C. et al. Int J Radiat Oncol Biol Phys
2008;70:1039–44. doi:10.1016/j.ijrobp.2007.07.2323.
EP-1597 Focal dose escalation in prostate cancer with
PSMA-PET/CT and MRI: planning study based on
histology
C. Zamboglou
1
, I. Sachpazidis
2
, K. Koubar
2
, V. Drendel
3
,
M. Werner
3
, H.C. Rischke
1
, M. Langer
4
, F. Schiller
5
, T.
Krauss
4
, R. Wiehle
2
, P.T. Meyer
5
, A.L. Grosu
1
, D. Baltas
2
1
Medical Center - University of Freiburg, Department of
Radiation Oncology, Freiburg, Germany
2
Medical Center - University of Freiburg, Division of
Medical Physics- Department of Radiation Oncology,
Freiburg, Germany
3
Medical Center - University of Freiburg, Department of
Pathology, Freiburg, Germany
4
Medical Center - University of Freiburg, Department of
Radiology, Freiburg, Germany
5
Medical Center - University of Freiburg, Department of
Nuclear Medicine, Freiburg, Germany
Purpose or Objective
First studies could show an increase in sensitivity when
primary prostate cancer (PCa) was detected by addition of
MRI and PSMA PET/CT information. On the other side the
highest specificity was achieved when the intersection
volume between MRI and PSMA PET/CT was considered.
Aim of this study was to demonstrate the technical
feasibility and to evaluate the tumor control probability
(TCP) and normal tissue complication probability (NTCP)
of IMRT dose painting using combined
68
Ga-HBED-CC PSMA-
PET/CT and multiparametric MRI (mpMRI) information in
patients with primary PCa.
Material and Methods
7 patients (5 intermediate + 2 high risk) with biopsy-
proven primary PCa underwent
68
Ga-HBED-CC-PSMA
PET/CT and mpMRI followed by prostatectomy. Resected
prostates were scanned by ex-vivo CT in a localizer and
prepared for histopathology. PCa was delineated on
histologic slices and matched to ex-vivo CT to obtain GTV-
histo. Ex-vivo CT including GTV-histo and MRI data were
matched to in-vivo CT(PET). Contours based on MRI (GTV-
MRI, consensus volume by two experienced radiologist),
PSMA PET (GTV-PET, semiautomatic using 30% of SUVmax
within the prostate) or the combination of both (GTV-
union/-intersection) were created. Three IMRT plans were
generated for each patient: PLAN77, which consisted of
whole-prostate radiation therapy to 77 Gy in 2.2 Gy per
fraction; PLAN95, which consisted of whole-prostate RT to
77 Gy in 2.2 Gy per fraction, and a simultaneous
integrated boost to the GTV-union (Plan95
union
)/-
intersection (Plan95
intersection
) to 95 Gy in 2.71 Gy per
fraction. The feasibility of these plans was judged by their
ability to reach prescription doses while adhering to the
FLAME trial protocol. TCPs based on co-registered
histology after prostatectomy (TCP-histo), and normal
tissue complication probabilities (NTCP) for rectum and
bladder were compared between the plans.
Results